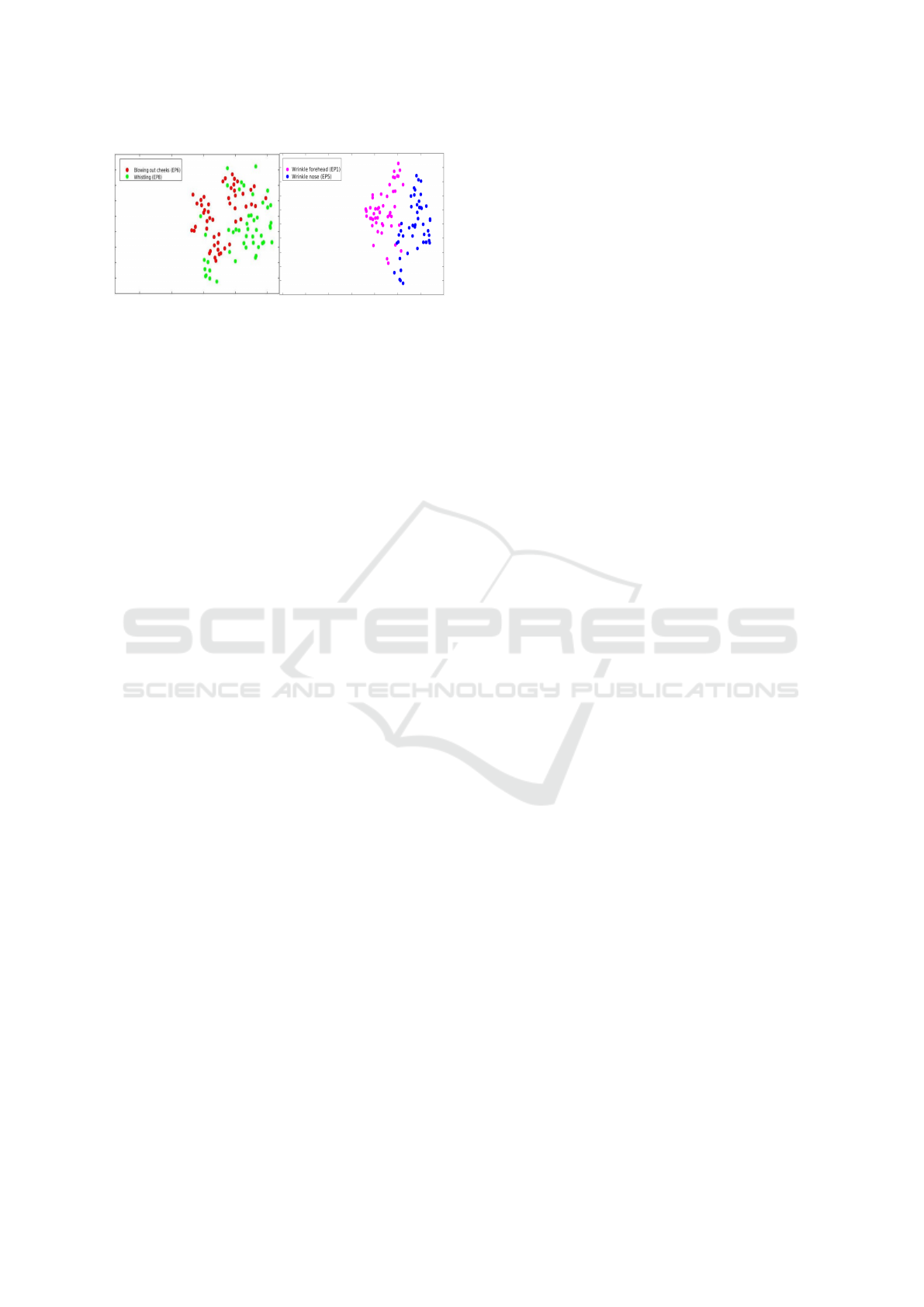
(a) (b)
Figure 8: t-sne plot for the expressions of facial paralysis
using MBH and GMM-MIK dynamic kernel based SVM
for 256 components for 3-class grading score (best viewed
in color). In (a) t-sne plot for expression blowing out cheeks
(EP6) and whistling (EP8) and in (b) for expression wrinkle
forehead (EP1) and wrinkle nose (EP5).
5 CONCLUSION
In this paper, we introduce a novel representation
of the facial features for variable length pattern us-
ing dynamic kernel-based classification, which pro-
vide the quantitative assessment to the patients suffer-
ing from facial paralysis. Dynamic kernels are used
for representing the varying length videos efficiently
by capturing both local facial dynamics and preserv-
ing the global context. A universal Gaussian mixture
model (GMM) is trained on spatio-temporal features
to compute the posteriors, first-order, and second-
order statistics for computing dynamic kernel-based
representations. We have shown that the efficacy of
the proposed approach using different dynamic ker-
nels on the collected video dataset of facially par-
alyzed patients. Also, we have shown the compu-
tation complexity and classification performance of
each dynamic kernels, where the matching based in-
termediate matching kernel (IMK) is computationally
efficient as compared to other dynamic kernels. How-
ever, probability-based mean interval kernel (MIK) is
more discriminative but computationally complex. In
the future, the classification performance has to be im-
proved further by improving the modeling of expres-
sions for better quantitative assessment of the facial
paralysis. Also, various quantitative assessment using
Perveen et al. (2012); Perveen et al. (2018); Perveen
et al. (2016) are need to be explore and compare for
better classification performance.
REFERENCES
Banks, C. A., Bhama, P. K., Park, J., Hadlock, C. R., and
Hadlock, T. A. (2015). Clinician-Graded Electronic
Facial Paralysis Assessment: The eFACE. Plast. Re-
constr. Surg., 136(2):223e–230e.
Cortes, C. and Vapnik, V. (1995). Support-vector networks.
Machine Learning, 20(3):273–297.
Dileep, A. D. and Sekhar, C. C. (2014). Gmm-based inter-
mediate matching kernel for classification of varying
length patterns of long duration speech using support
vector machines. IEEE Transactions on Neural Net-
works and Learning Systems, 25(8):1421–1432.
Guo, Z., Shen, M., Duan, L., Zhou, Y., Xiang, J., Ding, H.,
Chen, S., Deussen, O., and Dan, G. (2017). Deep as-
sessment process: Objective assessment process for
unilateral peripheral facial paralysis via deep con-
volutional neural network. In 2017 IEEE 14th In-
ternational Symposium on Biomedical Imaging (ISBI
2017), pages 135–138.
Hato, N., Fujiwara, T., Gyo, K., and Yanagihara, N. (2014).
Yanagihara facial nerve grading system as a prognos-
tic tool in Bell’s palsy. Otol. Neurotol., 35(9):1669–
1672.
He, S., Soraghan, J. J., O’Reilly, B. F., and Xing, D. (2009).
Quantitative analysis of facial paralysis using local bi-
nary patterns in biomedical videos. IEEE Transac-
tions on Biomedical Engineering, 56(7):1864–1870.
House, J. W. and Brackmann, D. E. (1985). Facial nerve
grading system. Otolaryngology-Head and Neck
Surgery, 93(2):146–147. PMID: 3921901.
Liu, X., Dong, S., An, M., Bai, L., and Luan, J. (2015).
Quantitative assessment of facial paralysis using in-
frared thermal imaging. In 2015 8th International
Conference on Biomedical Engineering and Informat-
ics (BMEI), pages 106–110.
NGO, T. H., CHEN, Y.-W., MATSUSHIRO, N., and SEO,
M. (2016). Quantitative assessment of facial paralysis
based on spatiotemporal features. IEICE Transactions
on Information and Systems, E99.D(1):187–196.
Ngo, T. H., Chen, Y. W., Seo, M., Matsushiro, N., and
Xiong, W. (2016). Quantitative analysis of facial
paralysis based on three-dimensional features. In 2016
IEEE International Conference on Image Processing
(ICIP), pages 1319–1323.
Ngo, T. H., Seo, M., Chen, Y.-W., and Matsushiro, N.
(2014). Quantitative assessment of facial paralysis us-
ing local binary patterns and gabor filters. In Proceed-
ings of the Fifth Symposium on Information and Com-
munication Technology, SoICT ’14, pages 155–161,
New York, NY, USA. ACM.
Perveen, N., Gupta, S., and Verma, K. (2012). Facial ex-
pression recognition using facial characteristic points
and gini index. In 2012 Students Conference on Engi-
neering and Systems, pages 1–6.
Perveen, N., Roy, D., and Mohan, C. K. (2018). Sponta-
neous expression recognition using universal attribute
model. IEEE Transactions on Image Processing,
27(11):5575–5584.
Perveen, N., Singh, D., and Mohan, C. K. (2016). Sponta-
neous facial expression recognition: A part based ap-
proach. In 2016 15th IEEE International Conference
on Machine Learning and Applications (ICMLA),
pages 819–824.
Satoh, Y., Kanzaki, J., and Yoshihara, S. (2000). A com-
parison and conversion table of ’the house-brackmann
Quantitative Analysis of Facial Paralysis using GMM and Dynamic Kernels
183