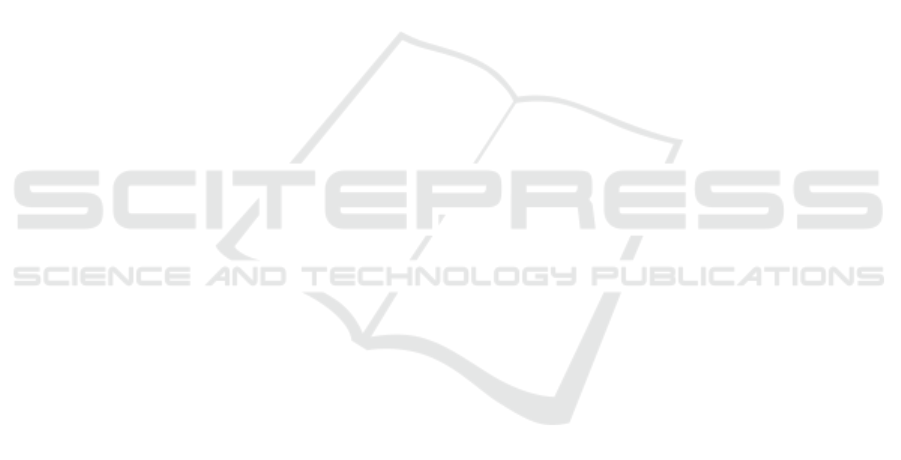
groups, as shown, by defining different characteris-
tics.
The implementation of multi-state models is not
straightforward, especially when considering more
complex states structures than the disability model.
Several issues must be taken into account, which were
out of the scope of this work. First, a Markov dis-
ability model has been considered, hence discarding
a semi-Markov model, i.e. a model in which sur-
vival time for a patient who has experienced a post-
treatment MI depends on the time from treatment
to MI. Therefore, the Markov assumption must be
checked during the analysis. Second, the choice of
the survival models to be use for transition times is
of great importance, and only some options can be
found already implemented in commercial software.
Estimation of the transition intensities will be inac-
curate when a low number of events are observed for
a transition, as can be observed in the results of this
work. Third, model assessment and variable selection
in this setting are still open questions.
Future versions of this work will consider
Bayesian inference in order to be able to work with
more sophisticated states structures and different sur-
vival models, as in (Armero et al., 2016). In that
work, although a relatively simple parametric model
for the hazard function (of each transition) was con-
sidered, posterior distributions of parameters had to
be approximated as software was unavailable, even
for a disability model. Our future research will surely
be a challenge in this sense, especially if a more com-
plex state structure is to be considered. That said,
Bayesian inference also has the advantage of stating
results in terms of probabilities, which are directly in-
terpretable. Such approach would allow us to give
personalized predictions, for example, the probabil-
ity of suffering a MI in the next three months taking
into account the patient’s history. Additionally, the
Bayesian approach provides an ideal framework for
the assessment of the model and for variable selection
in multi-state models, via the Bayes factor and model
simulation.
ACKNOWLEDGEMENTS
The authors want to thank the Epic foundation for
providing the data and useful insights about the re-
sults.
REFERENCES
Andersen, P., Borgan, P., Gill, R., and et al. (1993). Statis-
tical models based on counting processes. Springer,
New York.
Armero, C., Cabras, S., Castellanos, M., Perra, S., Quir
´
os,
A., Oruezabal, M., and Sanchez-Rubio, J. (2016).
Bayesian analysis of a disability model for lung can-
cer survival. Statistical Methods in Medical Research,
25(1):336–351.
Austin, P., Lee, D., and Fine, J. (2016). Introduction to the
analysis of survival data in the presence of competing
risks. Circulation, 133:601–609.
De la Torre Hernandez, J., Moreno, R., Gonzalo, N.,
Rivera, R., Linares, J., Veiga Fernandez, G., Gomez
Menchero, A., Garcia del Blanco, B., Hernandez,
F., Benito Gonzalez, T., Subinas, A., and Escaned,
J. (2018). The pt-cr everolimus stent with bioab-
sorbable polymer in the treatment of patients with
acute cononary syndromes. results from the syn-
ergy acs registry. Cardiovascular Revascularization
Medicine.
Ferreira-Gonzalez, I., Alonso-Coello, P., Sola, I., Pacheco-
Huergo, V., Domingo-Salvany, A., Alonso, J., Mon-
tori, V., and Permanyer-Miralda, G. (2008). Compos-
ite endpoints in clinical trials. Revista Espa
˜
nola de
Cardiolog
´
ıa, 61(3):283–290.
Irony, T. (2017). The “utility” in composite out-
come measures. measuring what is important to pa-
tients. Journal of the American Medical Association,
318(18):1820.
Jackson, C. (2011). Multi-state models for panel data: The
msm package for R. Journal of Statistical Software,
38(8):1–29.
Kassambara, A., Kosinski, M., and Biecek, P. (2019).
survminer: Drawing Survival Curves using ’ggplot2’.
Kip, K., Hollabaugh, K., Marroquin, O., and Williams, D.
(2008). The problem with composite end points in
cardiovascular studies: The story of major adverse
cardiac events and percutaneous coronary interven-
tion. Journal of the American College of Cardiology,
51(7):701–707.
McCoy, C. (2018). Understanding the use of composite
endpoints in clinical trials. Western Journal of Emer-
gency Medicine, 19(4):631–634.
Meira-Machado, L., de U
˜
na-
´
Alvarez, J., Cadarso-Su
´
arez,
C., and Andersen, P. (2009). Multi-state models for
the analysis of time-to-event data. Statistical Methods
in Medical Research, 18:195–222.
N
´
u
˜
nez, E., Steyerberg, E., and N
´
u
˜
nez, J. (2011). Estrate-
gias para la elaboraci
´
on de modelos estad
´
ısticos de re-
gresi
´
on. Revista Espa
˜
nola de Cardiolog
´
ıa, 64:501–
507.
Putter, H., Fiocco, M., and Geskus, R. (2007). Tutorial in
biostatistics: competing risks and multi-state models.
Statistics in Medicine, 26:142–161.
R Core Team (2019). R: A Language and Environment for
Statistical Computing. R Foundation for Statistical
Computing, Vienna, Austria.
Therneau, T. M. (2015). A Package for Survival Analysis in
S.
Wickham, H. (2016). ggplot2: Elegant Graphics for Data
Analysis. Springer-Verlag New York.
Multi-state Models for the Analysis of Survival Studies in Biomedical Research: An Alternative to Composite Endpoints
199