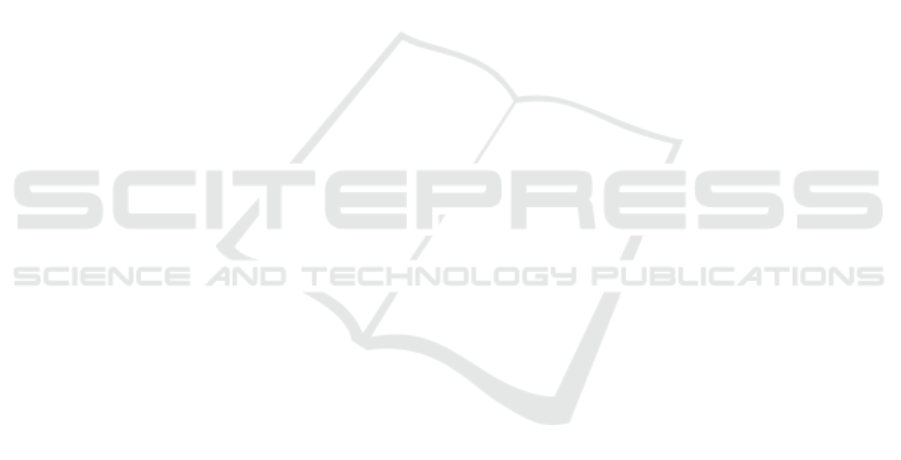
that is, how large can the missing regions be reduced.
This parameter should be one of the factors affecting
the accuracy improvement.
5 CONCLUSION
This paper proposed a recursive framework for rain-
drop removal in a vehicle video camera. The method
first detects raindrops in each of an input image se-
quence by using a method based on the technique
(Qian et al., 2018), and then restored each image con-
sidering the temporal consistency by using a method
based on the technique (Xu et al., 2019). The results
of the first preliminary experiment showed the effec-
tiveness and the problem of the method without intro-
ducing the concept of the recursive image restoration
toward raindrop detection and removal. The second
preliminary experiment showed the validity of the as-
sumption of the proposed concept, that is, the assump-
tion that the accuracy of optical flow restoration in the
outer part of the missing region is higher than the in-
ner part. The results of the main evaluation experi-
ments showed the proposed recursive framework has
the potential for improving the restoration accuracy.
The future work includes the study on 1) how to
deal with the error propagation and 2) how to reduce
missing regions over time in the proposed recursive
restoration. In addition, we will study a way for tak-
ing various possible situations into account, such as
small vehicle motion and many raindrops attached to
the lens of a camera, which may be the factors to
decrease the accuracy of raindrop removal. Further-
more, the proposed method restores the middle frame
of input frames. We will also investigate the restora-
tion accuracy with the last frame of input ones in order
to remove raindrops without delay.
REFERENCES
Barnum, P. C., Narasimhan, S., and Kanade, T. (2010).
Analysis of rain and snow in frequency space. Interna-
tional Journal of Computer Vision, 86(2-3):256–274.
Garg, K. and Nayar, S. K. (2007). Vision and rain. Interna-
tional Journal of Computer Vision, 75(1):3–27.
Goodfellow, I., Pouget-Abadie, J., Mirza, M., Xu, B.,
Warde-Farley, D., Ozair, S., Courville, A., and Ben-
gio, Y. (2014). Generative adversarial nets. In
Advances in neural information processing systems,
pages 2672–2680.
He, K., Sun, J., and Tang, X. (2011). Single image haze
removal using dark channel prior. IEEE Transac-
tions on Pattern Analysis and Machine Intelligence,
33(12):2341–2353.
He, K., Zhang, X., Ren, S., and Sun, J. (2016). Deep resid-
ual learning for image recognition. In Proceedings of
2016 IEEE Conference on Computer Vision and Pat-
tern Recognition, pages 770–778.
Huang, J.-B., Kang, S. B., Ahuja, N., and Kopf, J. (2016).
Temporally coherent completion of dynamic video.
ACM Transactions on Graphics, 35(6):1–11.
Iizuka, S., Simo-Serra, E., and Ishikawa, H. (2017). Glob-
ally and locally consistent image completion. ACM
Transactions on Graphics, 36(4):1–14.
Ilg, E., Mayer, N., Saikia, T., Keuper, M., Dosovitskiy, A.,
and Brox, T. (2017). FlowNet 2.0: Evolution of opti-
cal flow estimation with deep networks. In Proceed-
ings of 2017 IEEE Conference on Computer Vision
and Pattern Recognition, pages 2462–2470.
Kurihata, H., Takahashi, T., Ide, I., Mekada, Y., Murase, H.,
Tamatsu, Y., and Miyahara, T. (2005). Rainy weather
recognition from in-vehicle camera images for driver
assistance. In Proceedings of 2005 IEEE Intelligent
Vehicles Symposium, pages 205–210.
Liu, G., Reda, F. A., Shih, K. J., Wang, T.-C., Tao, A., and
Catanzaro, B. (2018). Image inpainting for irregu-
lar holes using partial convolutions. In Proceedings
of 2018 European Conference on Computer Vision,
pages 85–100.
Newson, A., Almansa, A., Fradet, M., Gousseau, Y., and
P
´
erez, P. (2014). Video inpainting of complex scenes.
SIAM Journal on Imaging Sciences, 7(4):1993–2019.
Qian, R., Tan, R. T., Yang, W., Su, J., and Liu, J. (2018).
Attentive generative adversarial network for raindrop
removal from a single image. In Proceedings of 2018
IEEE Conference on Computer Vision and Pattern
Recognition, pages 2482–2491.
Wexler, Y., Shechtman, E., and Irani, M. (2004). Space-
time video completion. In Proceedings of 2004 IEEE
Conference on Computer Vision and Pattern Recogni-
tion, volume 1, pages 120–127.
Xingjian, S., Chen, Z., Wang, H., Yeung, D.-Y., Wong, W.-
K., and Woo, W.-c. (2015). Convolutional lstm net-
work: A machine learning approach for precipitation
nowcasting. In Advances in neural information pro-
cessing systems 28, pages 802–810.
Xu, R., Li, X., Zhou, B., and Loy, C. C. (2019). Deep flow-
guided video inpainting. In Proceedings of 2019 IEEE
Conference on Computer Vision and Pattern Recogni-
tion, pages 3723–3732.
You, S., Tan, R. T., Kawakami, R., Mukaigawa, Y., and
Ikeuchi, K. (2015). Adherent raindrop modeling,
detection and removal in video. IEEE Transac-
tions on Pattern Analysis and Machine Intelligence,
38(9):1721–1733.
Yu, J., Lin, Z., Yang, J., Shen, X., Lu, X., and Huang, T. S.
(2018). Generative image inpainting with contextual
attention. In Proceedings of 2018 IEEE Conference
on Computer Vision and Pattern Recognition, pages
5506–5514.
VISAPP 2020 - 15th International Conference on Computer Vision Theory and Applications
436