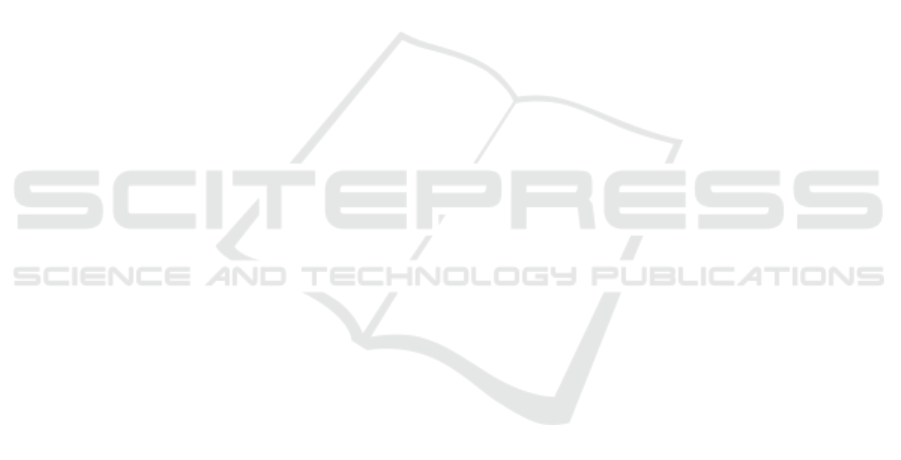
be repaired, thus working towards achieving the goals
set by the World Health Organization on reducing the
deaths of pedestrians.
ACKNOWLEDGEMENTS
This project has received funding from the European
Unions Horizon 2020 research and innovation pro-
gramme under grant agreement No 739578 comple-
mented by the Government of the Republic of Cyprus
through the Directorate General for European Pro-
grammes, Coordination and Development.
REFERENCES
Bano, S., Suveges, T., Zhang, J., and McKenna, S. (2018).
Multimodal egocentric analysis of focused interac-
tions. IEEE Access, 6:1–13.
Bola
˜
nos, M., Dimiccoli, M., and Radeva, P. (2015).
Toward storytelling from visual lifelogging: An
overview. IEEE Transactions on Human-Machine
Systems, 47:77–90.
Bolaos, M. and Radeva, P. (2016). Simultaneous food lo-
calization and recognition. In 23rd International Con-
ference on Pattern Recognition (ICPR), pages 3140–
3145.
Bullock, I. M., Feix, T., and Dollar, A. M. (2015). The yale
human grasping dataset: Grasp, object, and task data
in household and machine shop environments. The In-
ternational Journal of Robotics Research, 34(3):251–
255.
Chollet, F. et al. (2015). Keras. https://keras.io.
Climent-Prez, P., Spinsante, S., Michailidis, A., and Flrez-
Revuelta, F. (2020). A review on video-based active
and assisted living technologies for automated lifelog-
ging. Expert Systems with Applications, 139:112847.
Damen, D., Doughty, H., Farinella, G. M., Fidler, S.,
Furnari, A., Kazakos, E., Moltisanti, D., Munro, J.,
Perrett, T., Price, W., and Wray, M. (2018). Scaling
egocentric vision: The epic-kitchens dataset. In Euro-
pean Conference on Computer Vision (ECCV).
Dutta, A. and Zisserman, A. (2019). The VIA annotation
software for images, audio and video. arXiv preprint
arXiv:1904.10699.
Everingham, M., Van Gool, L., Williams, C. K., Winn, J.,
and Zisserman, A. (2007). The pascal visual object
classes challenge 2007 (voc2007) results.
Furnari, A., Farinella, G. M., and Battiato, S. (2017). Rec-
ognizing personal locations from egocentric videos.
IEEE Transactions on Human-Machine Systems,
47(1):6–18.
Gopalakrishnan, K. (2018). Deep learning in data-driven
pavement image analysis and automated distress de-
tection: A review. Data, 3(3):28.
Gu, J., Wang, Z., Kuen, J., Ma, L., Shahroudy, A., Shuai, B.,
Liu, T., Wang, X., Wang, G., Cai, J., et al. (2018). Re-
cent advances in convolutional neural networks. Pat-
tern Recognition, 77:354–377.
Gurrin, C., Joho, H., Hopfgartner, F., Zhou, L., and Albatal,
R. (2016). Overview of ntcir-12 lifelog task.
He, K., Zhang, X., Ren, S., and Sun, J. (2016). Deep resid-
ual learning for image recognition. In Proceedings of
the IEEE conference on computer vision and pattern
recognition, pages 770–778.
Herruzo, P., Portell, L., Soto, A., and Remeseiro, B.
(2017). Analyzing first-person stories based on so-
cializing, eating and sedentary patterns. In Battiato,
S., Farinella, G. M., Leo, M., and Gallo, G., edi-
tors, New Trends in Image Analysis and Processing
– ICIAP 2017.
Jain, S. and Gruteser, M. (2017). Recognizing textures
with mobile cameras for pedestrian safety applica-
tions. IEEE Transactions on Mobile Computing, PP.
Jiang, B., Yang, J., Lv, Z., and Song, H. (2019). Wearable
vision assistance system based on binocular sensors
for visually impaired users. IEEE Internet of Things
Journal, 6(2):1375–1383.
Krizhevsky, A., Sutskever, I., and Hinton, G. E. (2012). Im-
agenet classification with deep convolutional neural
networks. In Advances in neural information process-
ing systems, pages 1097–1105.
LeCun, Y., Bottou, L., Bengio, Y., Haffner, P., et al. (1998).
Gradient-based learning applied to document recogni-
tion. Proceedings of the IEEE, 86(11):2278–2324.
Lin, T.-Y., Maire, M., Belongie, S., Hays, J., Perona, P.,
Ramanan, D., Doll
´
ar, P., and Zitnick, C. L. (2014).
Microsoft coco: Common objects in context. In Euro-
pean conference on computer vision, pages 740–755.
Maeda, H., Sekimoto, Y., Seto, T., Kashiyama, T., and
Omata, H. (2018). Road damage detection and classi-
fication using deep neural networks with smartphone
images: Road damage detection and classification.
Computer-Aided Civil and Infrastructure Engineer-
ing, 33.
Mayol, W. W. and Murray, D. W. (2005). Wearable hand
activity recognition for event summarization. In Ninth
IEEE International Symposium on Wearable Comput-
ers (ISWC’05), pages 122–129.
Nesoff, E., Porter, K., Bailey, M., and Gielen, A. (2018).
Knowledge and beliefs about pedestrian safety in an
urban community: Implications for promoting safe
walking. Journal of Community Health, 44.
Oliveira-Barra, G., Bolaos, M., Talavera, E., Gelonch, O.,
Garolera, M., and Radeva, P. (2019). Lifelog retrieval
for memory stimulation of people with memory im-
pairment, pages 135–158.
Prathiba, T., Thamaraiselvi, M., Mohanasundari, M., and
Veerelakshmi, R. (2015). Pothole detection in road us-
ing image processing. International Journal of Man-
agement, Information Technology and Engineering,
3(4):13–20.
Russakovsky, O., Deng, J., Su, H., Krause, J., Satheesh, S.,
Ma, S., Huang, Z., Karpathy, A., Khosla, A., Bern-
stein, M., et al. (2015). Imagenet large scale visual
A First-person Database for Detecting Barriers for Pedestrians
665