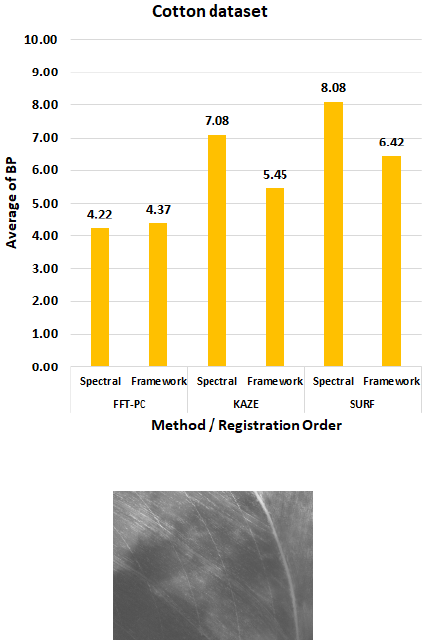
Figure 8: Average of BP error for the cotton dataset.
Figure 9: Example of shadow present in soybean dataset.
posed to work with keypoints based methods, so that
spectral domain methods (e.g. Phase Correlation) can
be used in the registration process.
Our modification generated, for both datasets
evaluated, the same registration order as obtained by
the original framewrok. However, our approach has
considerably reduced its execution time, thus making
it feasible to apply to large datasets. Moreover, our
modification reduced the back projection error of the
alignment when compared with the spectral order.
Although several methods in the literature use spa-
tial methods for multispectral image alignment ob-
tained by UAVs, the quality obtained by the spectral
method (FFT-PC) was considerably superior, which
corroborates this approach as an alternative for multi-
spectral registration of images obtained by UAVs.
ACKNOWLEDGEMENTS
The authors gratefully acknowledges CAPES (Co-
ordination for the Improvement of Higher Edu-
cation Personnel) (Finance Code 001) and CNPq
(National Council for Scientific and Technologi-
cal Development, Brazil) (Grant #301715/2018-1)
for the financial support and the company Sensix
(http://sensix.com.br) for providing the images used
in the tests.
REFERENCES
Alcantarilla, P. F., Bartoli, A., and Davison, A. J. (2012).
Kaze features. In Fitzgibbon, A., Lazebnik, S., Per-
ona, P., Sato, Y., and Schmid, C., editors, Computer
Vision – ECCV 2012, pages 214–227, Berlin, Heidel-
berg. Springer Berlin Heidelberg.
Banerjee, B. P., Raval, S. A., and Cullen, P. J. (2018). Align-
ment of uav-hyperspectral bands using keypoint de-
scriptors in a spectrally complex environment. Remote
Sensing Letters, 9(6):524–533.
Bay, H., Tuytelaars, T., and Van Gool, L. (2006). Surf:
Speeded up robust features. In Leonardis, A., Bischof,
H., and Pinz, A., editors, Computer Vision – ECCV
2006, pages 404–417, Berlin, Heidelberg. Springer
Berlin Heidelberg.
Berni, J. A. J., Zarco-Tejada, P. J., Suarez, L., and Fereres,
E. (2009). Thermal and narrowband multispectral re-
mote sensing for vegetation monitoring from an un-
manned aerial vehicle. IEEE Transactions on Geo-
science and Remote Sensing, 47(3):722–738.
Dias Junior, J. D., Backes, A., and Escarpinati, M. (2019).
Detection of control points for uav-multispectral
sensed data registration through the combining of fea-
ture descriptors. In Proceedings of the 14th Interna-
tional Joint Conference on Computer Vision, Imag-
ing and Computer Graphics Theory and Applications,
pages 444–451.
Diaz-Varela, R., Zarco-Tejada, P., Angileri, V., and Loud-
jani, P. (2014). Automatic identification of agricul-
tural terraces through object-oriented analysis of very
high resolution dsms and multispectral imagery ob-
tained from an unmanned aerial vehicle. Journal of
Environmental Management, 134:117 – 126.
Floyd, R. W. (1962). Algorithm 97: Shortest path. Com-
mun. ACM, 5(6):345–.
Gago, J., Douthe, C., Coopman, R., Gallego, P., Ribas-
Carbo, M., Flexas, J., Escalona, J., and Medrano, H.
(2015). Uavs challenge to assess water stress for sus-
tainable agriculture. Agricultural Water Management,
153:9 – 19.
Gevaert, C. M., Suomalainen, J., Tang, J., and Kooistra, L.
(2015). Generation of spectraltemporal response sur-
faces by combining multispectral satellite and hyper-
spectral uav imagery for precision agriculture appli-
cations. IEEE Journal of Selected Topics in Applied
Earth Observations and Remote Sensing, 8(6):3140–
3146.
Hunt, E. R., Hively, W. D., Fujikawa, S. J., Linden, D. S.,
Daughtry, C. S. T., and McCarty, G. W. (2010). Acqui-
sition of nir-green-blue digital photographs from un-
manned aircraft for crop monitoring. Remote Sensing,
2:290–305.