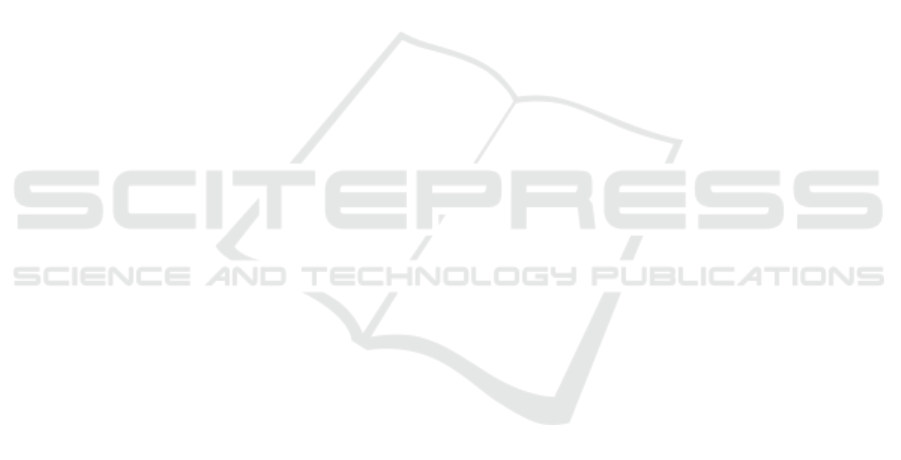
3 useful learning techniques for anomaly detection.
For future work, the very next steps is evaluate
our framework on 3 benchmarks following experi-
ment plan. Then we investigate to integrate as much
as components for an end-to-end network.
REFERENCES
Finn, C., Goodfellow, I. J., and Levine, S. (2016). Unsuper-
vised learning for physical interaction through video
prediction. NIPS.
Gao, R., Xiong, B., and Grauman, K. (2017). Im2flow: Mo-
tion hallucination from static images for action recog-
nition. 2018 IEEE/CVF Conference on Computer Vi-
sion and Pattern Recognition, pages 5937–5947.
Giorno, A. D., Bagnell, J. A., and Hebert, M. (2016). A dis-
criminative framework for anomaly detection in large
videos. In ECCV.
Hasan, M., Choi, J., Neumann, J., Roy-Chowdhury, A. K.,
and Davis, L. S. (2016). Learning temporal regularity
in video sequences. 2016 IEEE Conference on Com-
puter Vision and Pattern Recognition (CVPR), pages
733–742.
Hinami, R., Mei, T., and Satoh, S. (2017). Joint detection
and recounting of abnormal events by learning deep
generic knowledge. 2017 IEEE International Confer-
ence on Computer Vision (ICCV), pages 3639–3647.
Ionescu, R. T., Khan, F. S., Georgescu, M.-I., and Shao,
L. (2018). Object-centric auto-encoders and dummy
anomalies for abnormal event detection in video. In
CVPR.
Ionescu, R. T., Smeureanu, S., Alexe, B., and Popescu,
M. (2017). Unmasking the abnormal events in video.
2017 IEEE International Conference on Computer Vi-
sion (ICCV), pages 2914–2922.
Kim, J. and Grauman, K. (2009). Observe locally, infer
globally: A space-time mrf for detecting abnormal ac-
tivities with incremental updates. In 2009 IEEE Con-
ference on Computer Vision and Pattern Recognition,
pages 2921–2928.
Liu, W., Luo, W., Lian, D., and Gao, S. (2017). Future
frame prediction for anomaly detection - a new base-
line. 2018 IEEE/CVF Conference on Computer Vision
and Pattern Recognition, pages 6536–6545.
Lotter, W., Kreiman, G., and Cox, D. D. (2016). Deep pre-
dictive coding networks for video prediction and un-
supervised learning. ICLR.
Lu, C., Shi, J., and Jia, J. (2013). Abnormal event detection
at 150 fps in matlab.
Luo, W., Liu, W., and Gao, S. (2017). A revisit of sparse
coding based anomaly detection in stacked rnn frame-
work. 2017 IEEE International Conference on Com-
puter Vision (ICCV), pages 341–349.
Mahadevan, V., Li, W., Bhalodia, V., and Vasconcelos, N.
(2010). Anomaly detection in crowded scenes. In
2010 IEEE Computer Society Conference on Com-
puter Vision and Pattern Recognition, pages 1975–
1981.
Mathieu, M., Couprie, C., and LeCun, Y. (2015). Deep
multi-scale video prediction beyond mean square er-
ror. ICLR, abs/1511.05440.
Medioni, G., Cohen, I., Bremond, F., Hongeng, S., and
Nevatia, R. (2001). Event detection and analysis from
video streams. IEEE Transactions on Pattern Analysis
and Machine Intelligence, 23(8):873–889.
Nguyen, T. N. and Meunier, J. (2019). Anomaly detection
in video sequence with appearance-motion correspon-
dence. ICCV.
Oliu, M., Selva, J., and Escalera, S. (2017). Folded recur-
rent neural networks for future video prediction. In
ECCV.
Villegas, R., Yang, J., Hong, S., Lin, X., and Lee, H. (2017).
Decomposing motion and content for natural video se-
quence prediction. ICLR, abs/1706.08033.
Walker, J., Gupta, A., and Hebert, M. (2015). Dense optical
flow prediction from a static image. 2015 IEEE In-
ternational Conference on Computer Vision (ICCV),
pages 2443–2451.
Wang, J. and Cherian, A. (2019). Gods: Generalized one-
class discriminative subspaces for anomaly detection.
ICCV.
Wang, T. and Snoussi, H. (2012). Histograms of optical
flow orientation for visual abnormal events detection.
In 2012 IEEE Ninth International Conference on Ad-
vanced Video and Signal-Based Surveillance, pages
13–18.
Yin, Z., Darrell, T., and Yu, F. (2019). Hierarchical discrete
distribution decomposition for match density estima-
tion. In CVPR.
Zhang, T., Lu, H., and Li, S. Z. (2009). Learning seman-
tic scene models by object classification and trajectory
clustering. In 2009 IEEE Conference on Computer Vi-
sion and Pattern Recognition, pages 1940–1947.
VISAPP 2020 - 15th International Conference on Computer Vision Theory and Applications
490