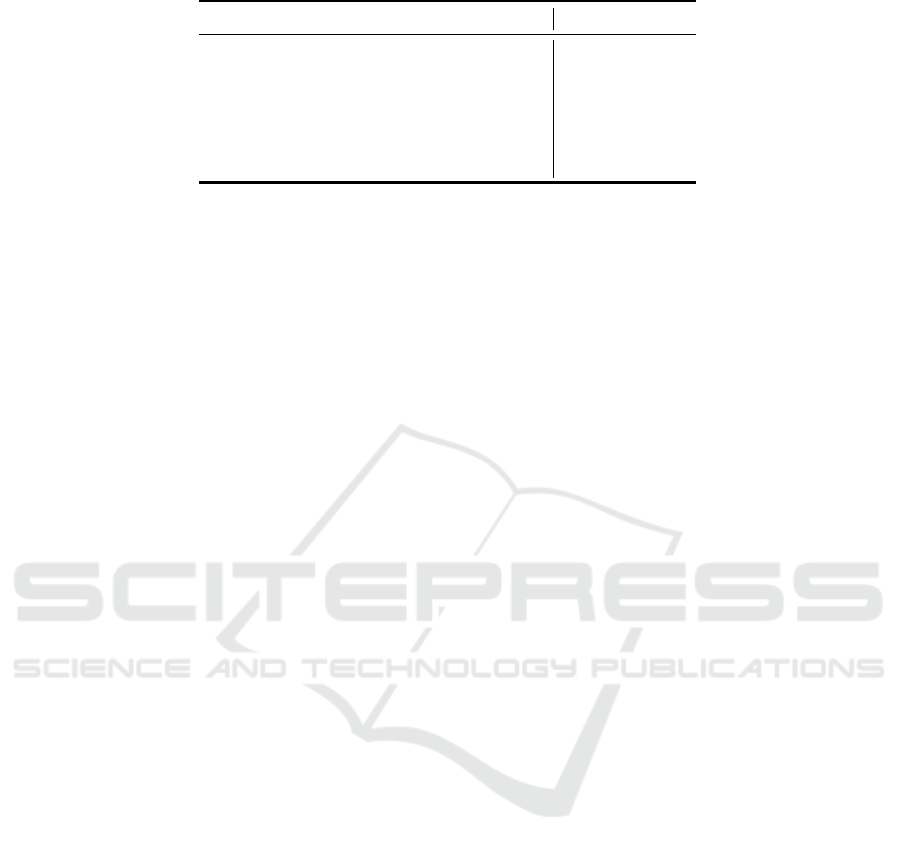
Table 4: Comparison result of the proposed method on Flavia dataset with the state-of-the-art other methods.
Method Accuracy (%)
Thi-Lan Le,2014 (Nguyen et al., 2017) 97.5
Wu et al., 2007 (Wu et al., 2007) 90.3
Kadir, 2014 (Kadir, 2014)
97.2
Pierre Barr, 2017 (Barr
´
e et al., 2017) 97.9
Serestina Viriri, 2016 (Kala et al., 2016b) 95.0
G-KDES+LBP-KDES+DBoW (Our) 98.0
organs for plant species identification as they remain
available throughout the year, other organs of plant
such as stem and fruits can also be considered for dif-
ferent plant species identification. Furthermore, this
work can also be extended to achieve favorable results
by utilizing deep convolutional neural networks in or-
der to evaluate their ability to identify plant species at
a large-scale.
ACKNOWLEDGEMENTS
The authors would like to acknowledge the support
by the Centre for Computer Vision Research (C
2
VR)
and Swarm Robotics Lab-NCRA, University of Engi-
neering and Technology (UET) Taxila, Pakistan.
REFERENCES
Barr
´
e, P., St
¨
over, B. C., M
¨
uller, K. F., and Steinhage, V.
(2017). Leafnet: A computer vision system for au-
tomatic plant species identification. Ecological Infor-
matics, 40:50–56.
Bo, L., Ren, X., and Fox, D. (2010). Kernel descriptors for
visual recognition. In Advances in neural information
processing systems, pages 244–252.
Chen, Q., Abedini, M., Garnavi, R., and Liang, X. (2014).
Ibm research australia at lifeclef2014: Plant identifi-
cation task. In CLEF (Working Notes), pages 693–
704.
Go
¨
eau, H., Bonnet, P., Barbe, J., Bakic, V., Joly, A.,
Molino, J.-F., Barthelemy, D., and Boujemaa, N.
(2012). Multi-organ plant identification. In Proceed-
ings of the 1st ACM international workshop on Mul-
timedia analysis for ecological data, pages 41–44.
ACM.
Go
¨
eau, H., Joly, A., Bonnet, P., Selmi, S., Molino, J.-F.,
Barth
´
el
´
emy, D., and Boujemaa, N. (2014a). Lifeclef
plant identification task 2014. In CLEF2014 Work-
ing Notes. Working Notes for CLEF 2014 Conference,
Sheffield, UK, September 15-18, 2014, pages 598–
615. CEUR-WS.
Go
¨
eau, H., Joly, A., Yahiaoui, I., Baki
´
c, V., Verroust-
Blondet, A., Bonnet, P., Barth
´
el
´
emy, D., Boujemaa,
N., and Molino, J.-F. (2014b). Plantnet participation
at lifeclef2014 plant identification task.
Kadir, A. (2014). A model of plant identification system us-
ing glcm, lacunarity and shen features. arXiv preprint
arXiv:1410.0969.
Kala, J. R., Viriri, S., and Moodley, D. (2016a). Sinuos-
ity coefficients for leaf shape characterisation. In Ad-
vances in Nature and Biologically Inspired Comput-
ing, pages 141–150. Springer.
Kala, J. R., Viriri, S., Moodley, D., and Tapamo, J. R.
(2016b). Leaf classification using convexity measure
of polygons. In International Conference on Image
and Signal Processing, pages 51–60. Springer.
Mehdipour Ghazi, M., Yanıko
˘
glu, B., Aptoula, E., Muslu,
¨
O., and
¨
Ozdemir, M. C. (2015). Sabanci-okan sys-
tem in lifeclef 2015 plant identification competition.
CLEF (Conference and Labs of the Evaluation Fo-
rum).
Murtaza, F., HaroonYousaf, M., and Velastin, S. A. (2018).
Da-vlad: Discriminative action vector of locally ag-
gregated descriptors for action recognition. In 2018
25th IEEE International Conference on Image Pro-
cessing (ICIP), pages 3993–3997. IEEE.
Nguyen, T. T.-N., Le, T.-L., Vu, H., Nguyen, H.-H., and
Hoang, V.-S. (2017). A combination of deep learn-
ing and hand-designed feature for plant identification
based on leaf and flower images. In Asian Confer-
ence on Intelligent Information and Database Sys-
tems, pages 223–233. Springer.
Wu, S. G., Bao, F. S., Xu, E. Y., Wang, Y.-X., Chang, Y.-
F., and Xiang, Q.-L. (2007). A leaf recognition algo-
rithm for plant classification using probabilistic neural
network. In 2007 IEEE international symposium on
signal processing and information technology, pages
11–16. IEEE.
Plant Species Identification using Discriminant Bag of Words (DBoW)
505