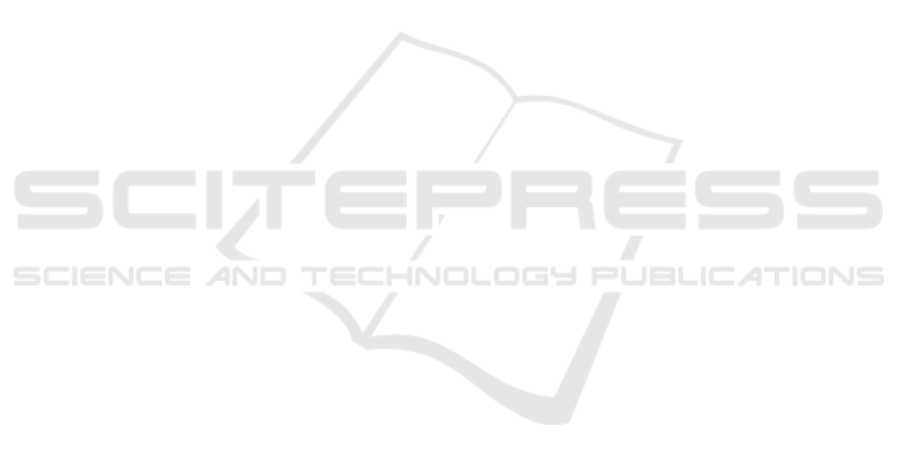
ture, as shown in Fig.7, for the purpose of pointing
out the contribution of inverse exponential map layer.
With reference to this architecture, the performance
of our proposed method improves with 4.84% using
cross-subject protocol and with 8.71% using cross-
view protocol.
Table.2 shows also that our non-Euclidean CNN-
LSTM based model achieves competitive result to the
state of the art (Shahroudy et al., 2016) in terms of
cross-subject accuracy. In fact, our model reaches
61.45% accuracy versus 62.93% in (Shahroudy et al.,
2016). For cross-view accuracy, our method outper-
forms the state of the art with 0.76% increase.
6 CONCLUSIONS
In this paper, we have proposed, for action recogni-
tion, to map skeleton sequences from the Riemannian
manifold to linear spaces, previous to feature extrac-
tion and learning layers. We proposed a first non-
Euclidean architecture based on CNNs to extract a
compact representation of each skeletons frame.We
then propose a second non-Euclidean temporally-
aware architecture based on CNN-LSTM networks.
We have tested the proposed approaches using two
datasets, namely Parkinson’s Vision-Based Pose Es-
timation dataset and NTU RGB+D dataset. Exper-
imental results have shown the effectiveness of the
proposed architectures compared to state of the art
models. However, for future work, we are working
1) on integrating our method with state of the art ar-
chitectures to consolidate its performance and 2) on
improving the geometry awareness of deep learning
architecture for action recognition by modifying the
inner operations of the CNN network.
ACKNOWLEDGEMENTS
This work has been jointly supported by Talan In-
novation Factory, Talan Tunisia, Talan Group. Talan
is a French digital transformation Consulting Group,
based in Paris, with offices in London, Geneva,
Madrid, Luxembourg, New York, Chicago, Montreal,
Toronto,Tunis, Rabat and Singapore. Talan Innova-
tion Factory provides expertise relative to disruptive
technologies such as Blockchain, Artificial Intelli-
gence, Data Science and Internet of Things. In the
frame of an academic-industry collaboration, Talan
has been persistently contributing to this work by pro-
viding Hardware resources (Deep learning platform),
mentoring and financial support.
REFERENCES
Baccouche, M., Mamalet, F., Wolf, C., Garcia, C., and
Baskurt, A. (2011). Sequential deep learning for hu-
man action recognition. In Human Behavior Un-
derstanding - Second International Workshop, HBU
2011, Amsterdam, The Netherlands, November 16,
2011. Proceedings, pages 29–39.
Bronstein, M. M., Bruna, J., LeCun, Y., Szlam, A., and
Vandergheynst, P. (2017). Geometric deep learning:
Going beyond euclidean data. IEEE Signal Process.
Mag., 34(4):18–42.
Ciresan, D. C., Meier, U., and Schmidhuber, J. (2012).
Multi-column deep neural networks for image classifi-
cation. In 2012 IEEE Conference on Computer Vision
and Pattern Recognition, Providence, RI, USA, June
16-21, 2012, pages 3642–3649.
Cohen, T. S., Geiger, M., Koehler, J., and Welling, M.
(2018). Spherical cnns.
Donahue, J., Hendricks, L. A., Rohrbach, M., Venugopalan,
S., Guadarrama, S., Saenko, K., and Darrell, T.
(2017a). Long-term recurrent convolutional networks
for visual recognition and description. IEEE Trans.
Pattern Anal. Mach. Intell., 39(4):677–691.
Donahue, J., Hendricks, L. A., Rohrbach, M., Venugopalan,
S., Guadarrama, S., Saenko, K., and Darrell, T.
(2017b). Long-term recurrent convolutional networks
for visual recognition and description. IEEE Trans.
Pattern Anal. Mach. Intell., 39(4):677–691.
Du, Y., Wang, W., and Wang, L. (2015). Hierarchical recur-
rent neural network for skeleton based action recog-
nition. In IEEE Conference on Computer Vision and
Pattern Recognition, CVPR 2015, Boston, MA, USA,
June 7-12, 2015, pages 1110–1118.
Graves, A. (2012). Supervised Sequence Labelling with Re-
current Neural Networks, volume 385 of Studies in
Computational Intelligence. Springer.
Graves, A., Mohamed, A., and Hinton, G. E. (2013).
Speech recognition with deep recurrent neural net-
works. In IEEE International Conference on Acous-
tics, Speech and Signal Processing, ICASSP 2013,
Vancouver, BC, Canada, May 26-31, 2013, pages
6645–6649.
Ke, Q., An, S., Bennamoun, M., Sohel, F. A., and Boussaïd,
F. (2017). Skeletonnet: Mining deep part features for
3-d action recognition. IEEE Signal Process. Lett.,
24(6):731–735.
Ke, Q. and Li, Y. (2014). Is rotation a nuisance in shape
recognition? In 2014 IEEE Conference on Computer
Vision and Pattern Recognition, CVPR 2014, Colum-
bus, OH, USA, June 23-28, 2014, pages 4146–4153.
Krizhevsky, A., Sutskever, I., and Hinton, G. E. (2012). Im-
agenet classification with deep convolutional neural
networks. In Advances in Neural Information Pro-
cessing Systems 25: 26th Annual Conference on Neu-
ral Information Processing Systems 2012. Proceed-
ings of a meeting held December 3-6, 2012, Lake
Tahoe, Nevada, United States, pages 1106–1114.
LeCun, Y. and Bengio, Y. (1998). The handbook of brain
theory and neural networks. chapter Convolutional
Geometric Deep Learning on Skeleton Sequences for 2D/3D Action Recognition
203