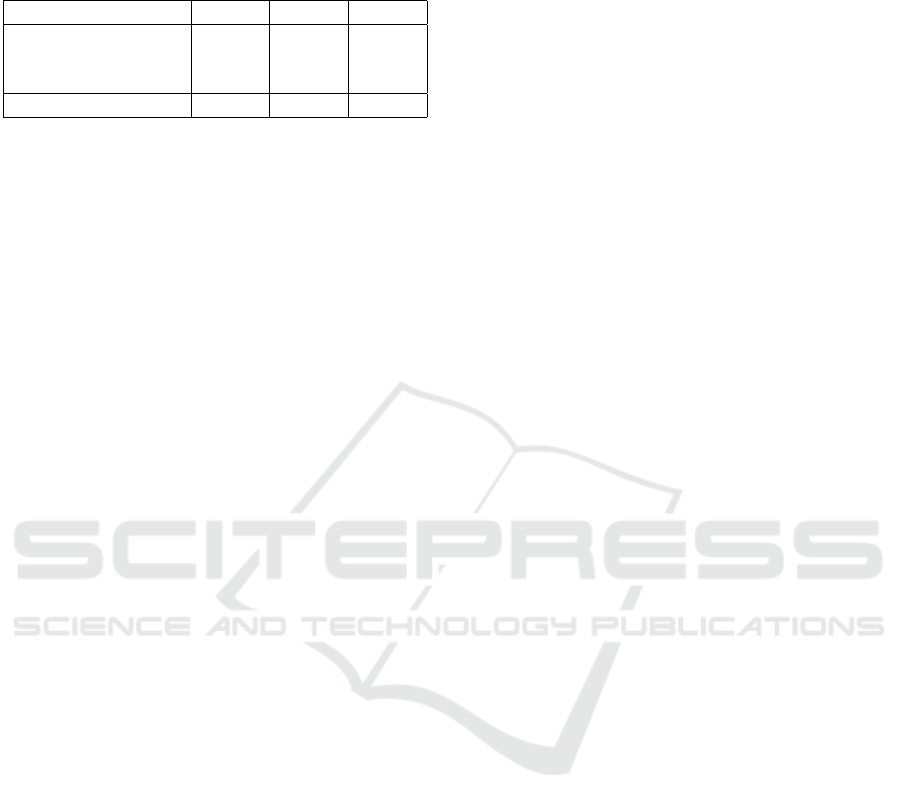
Table 3: Amounts of macronutrients in kcal for the food
ingested by the three users participating to the CheckY-
ourMeal! experiment.
User 1 User 2 User 3
Carbohydrates taken 9993 6810 15128
Proteins taken 1819 1200 1937
Lipids taken 4906 3845 7078
Total energy taken 16718 11855 24143
of whole grain rice correspond to 7 servings of non-
refined cereals. Finally, in step (4), from Table 1 we
find that this quantity contributes to the weekly MED
score with 5 points.
In Table 2 we report the MED scores for three dis-
tinct users participating to the CheckYourMeal! ex-
periment. The three users have assumed consider-
ably different amounts of food and of macronutrients,
as shown by Table 3, where User 3 has consumed a
high amount of food during the week (in average 3442
kcal/day), User 2 a low amount (1694 kcal/day) and
User 1 an intermediate value (2388 kcal/day). The
MED scores for the three users are similar (Table 2).
One can notice that the use of a Mediterranean recipe
book has resulted in a high MED score for all three
users. In particular, there are high scores for the con-
sumption of non-refined cereals, olive oil and vegeta-
bles for all the users, and a moderate score for the
consumption of potatoes. This example confirms the
intuition that quantitative constraints arising from di-
etary reference values on macronutrients and qualita-
tive constraints arising from the principles of Mediter-
ranean diet are two different notions that needed to be
treated with different mechanisms.
5 CONCLUSIONS
In this paper we have described a first proposal for the
ontological formalization of the Mediterranean score
MED as proposed in (Panagiotakos et al., 2007; Pana-
giotakos et al., 2006) as an extension of the food on-
tology PerkApp (Maimone et al., 2018). Moreover,
we have discussed how to effectively implement the
computation of MED in the framework of MADiMan,
a system for diet management. By using data from the
CheckYourMeal! experiment, we have computed in
MADiMan the MED score for three distinct users and
so we have empirically showed that macronutrients
and MED score are independent measures for rating
the quality of meals for a healthy diet, and as a conse-
quence it necessary to compute both independently.
To the best of our knowledge, this is the first work
that formalizes the MED score in terms of ontolog-
ical constraints and proposes an effective procedure
for computing it.
In future work we intend to use the computation
of the MED score to augment the answers returned
by MADiMan with an explanation about the compat-
ibility of a specific dish with the Mediterranean diet.
REFERENCES
Anselma, L. and Mazzei, A. (2015). Towards Diet Manage-
ment with Automatic Reasoning and Persuasive Natu-
ral Language Generation. In Progress in Artificial In-
telligence - 17th Portuguese Conference on Artificial
Intelligence, EPIA 2015, Coimbra, Portugal, Septem-
ber 8-11, 2015. Proceedings, pages 79–90.
Anselma, L. and Mazzei, A. (2017). An approach for ex-
plaining reasoning on the diet domain. In Basile, P.,
Croce, D., and Guerini, M., editors, Proc. of the 1st
Workshop on Natural Language for Artificial Intelli-
gence co-located with AI*IA 2017, Bari, Italy., vol-
ume 1983 of CEUR Workshop Proceedings, pages 4–
17. CEUR-WS.org.
Anselma, L. and Mazzei, A. (2018). Designing and testing
the messages produced by a virtual dietitian. In Krah-
mer, E., Gatt, A., and Goudbeek, M., editors, Pro-
ceedings of the 11th International Conference on Nat-
ural Language Generation, Tilburg University, The
Netherlands, November 5-8, 2018, pages 244–253.
Association for Computational Linguistics.
Anselma, L., Mazzei, A., and De Michieli, F. (2017).
An artificial intelligence framework for compensating
transgressions and its application to diet management.
Journal of Biomedical Informatics, 68:58–70.
Anselma, L., Mazzei, A., and Pirone, A. (2018). Automatic
reasoning evaluation in diet management based on an
Italian cookbook. In Proceedings of the Joint Work-
shop on Multimedia for Cooking and Eating Activities
and Multimedia Assisted Dietary Management, CEA/-
MADiMa ’18, pages 59–62, New York, NY, USA.
ACM.
Bailoni, T., Dragoni, M., Eccher, C., Guerini, M.,
and Maimone, R. (2016). Healthy lifestyle sup-
port: the perkapp ontology. In OWL: Experiences
and Directions–Reasoner Evaluation, pages 15–23.
Springer.
Bas, E. (2014). A robust optimization approach to diet prob-
lem with overall glycemic load as objective function.
Applied Mathematical Modelling, 38(19–20):4926 –
4940.
Buisson, J.-C. (2008). Nutri-educ, a nutrition software ap-
plication for balancing meals, using fuzzy arithmetic
and heuristic search algorithms. Artif. Intell. Med.,
42(3):213–227.
Donadello, I., Dragoni, M., and Eccher, C. (2019). Per-
suasive explanation of reasoning inferences on dietary
data. In Demidova, E., Dietze, S., Breslin, J. G.,
Gottschalk, S., Cimiano, P., Ell, B., Lawrynowicz,
A., Moss, L., and Ngomo, A. N., editors, Joint Pro-
ceedings of the 6th International Workshop on Dataset
Adopting the Mediterranean Diet Score in a Diet Management System
675