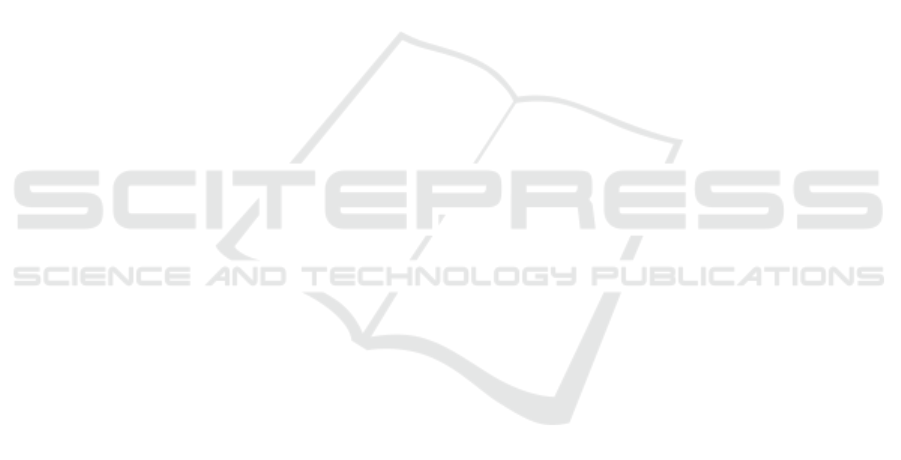
cancer histology images. IEEE transactions on medi-
cal imaging, 35(5):1313–1321.
Bardou, D., Zhang, K., and Ahmad, S. M. (2018). Clas-
sification of breast cancer based on histology images
using convolutional neural networks. IEEE Access,
6:24680–24693.
Bosch, A., Zisserman, A., and Mu
˜
noz, X. (2008). Scene
classification using a hybrid generative/discriminative
approach. IEEE transactions on pattern analysis and
machine intelligence, 30(4):712–727.
Breiman, L. (1996). Bagging predictors. Machine learning,
24(2):123–140.
Dalal, N. and Triggs, B. (2005). Histograms of Oriented
Gradients for human detection. In international Con-
ference on computer vision & Pattern Recognition
(CVPR’05), volume 1, pages 886–893. IEEE Com-
puter Society.
Esteva, A., Kuprel, B., Novoa, R. A., Ko, J., Swetter, S. M.,
Blau, H. M., and Thrun, S. (2017). Dermatologist-
level classification of skin cancer with deep neural net-
works. Nature, 542(7639):115.
Gandomkar, Z., Brennan, P. C., and Mello-Thoms, C.
(2018). Mudern: Multi-category classification of
breast histopathological image using deep residual
networks. Artificial intelligence in medicine, 88:14–
24.
Geusebroek, J.-M., Van den Boomgaard, R., Smeulders, A.
W. M., and Geerts, H. (2001). Color invariance. IEEE
Transactions on Pattern analysis and machine intelli-
gence, 23(12):1338–1350.
Gheisari, S., Catchpoole, D., Charlton, A., Melegh, Z.,
Gradhand, E., and Kennedy, P. (2018). Computer
aided classification of neuroblastoma histological im-
ages using scale invariant feature transform with fea-
ture encoding. Diagnostics, 8(3):56.
Han, Z., Wei, B., Zheng, Y., Yin, Y., Li, K., and Li,
S. (2017). Breast cancer multi-classification from
histopathological images with structured deep learn-
ing model. Scientific reports, 7(1):4172.
He, K., Zhang, X., Ren, S., and Sun, J. (2016). Deep resid-
ual learning for image recognition. In Proceedings of
the IEEE conference on computer vision and pattern
recognition, pages 770–778.
Ke, Y., Sukthankar, R., et al. (2004). PCA-SIFT: A more
distinctive representation for local image descriptors.
CVPR (2), 4:506–513.
Khan, S. and Yong, S.-P. (2016). A comparison of deep
learning and hand crafted features in medical image
modality classification. In 2016 3rd International
Conference on Computer and Information Sciences
(ICCOINS), pages 633–638. IEEE.
Kittler, J., Hatef, M., Duin, R. P., and Matas, J. (1998). On
combining classifiers. IEEE Trans. Pattern Analysis
and Machine Intelligence, 20(3):226–239.
Koziarski, M., Kwolek, B., and Cyganek, B. (2018).
Convolutional neural network-based classification of
histopathological images affected by data imbal-
ance. In Video Analytics. Face and Facial Expression
Recognition, pages 1–11. Springer.
Krizhevsky, A., Sutskever, I., and Hinton, G. E. (2012). Im-
ageNet classification with deep convolutional neural
networks. In Advances in neural information process-
ing systems, pages 1097–1105.
Lowe, D. G. (1999). Object recognition from local scale-
invariant features. In Computer vision, 1999. The pro-
ceedings of the seventh IEEE international conference
on, volume 2, pages 1150–1157. IEEE.
Mhala, N. C. and Bhandari, S. H. (2016). Improved ap-
proach towards classification of histopathology im-
ages using bag-of-features. In 2016 International
Conference on Signal and Information Processing
(IConSIP), pages 1–5. IEEE.
Pal, R. and Saraswat, M. (2019). Histopathological image
classification using enhanced bag-of-feature with spi-
ral biogeography-based optimization. Applied Intelli-
gence, pages 1–19.
Raza, S. H., Parry, R. M., Moffitt, R. A., Young, A. N.,
and Wang, M. D. (2011). An analysis of scale
and rotation invariance in the bag-of-features method
for histopathological image classification. In Inter-
national Conference on Medical Image Computing
and Computer-Assisted Intervention, pages 66–74.
Springer.
Simonyan, K. and Zisserman, A. (2014). Very deep con-
volutional networks for large-scale image recognition.
arXiv preprint arXiv:1409.1556.
Spanhol, F. A., Oliveira, L. S., Petitjean, C., and Heutte,
L. (2016a). Breast cancer histopathological image
classification using convolutional neural networks. In
2016 international joint conference on neural net-
works (IJCNN), pages 2560–2567. IEEE.
Spanhol, F. A., Oliveira, L. S., Petitjean, C., and Heutte, L.
(2016b). A dataset for breast cancer histopathological
image classification. IEEE Transactions on Biomedi-
cal Engineering, 63(7):1455–1462.
Van De Sande, K., Gevers, T., and Snoek, C. (2009). Eval-
uating color descriptors for object and scene recogni-
tion. IEEE transactions on pattern analysis and ma-
chine intelligence, 32(9):1582–1596.
Van de Weijer, J., Gevers, T., and Bagdanov, A. D. (2006).
Boosting color saliency in image feature detection.
IEEE transactions on pattern analysis and machine
intelligence, 28(1):150–156.
Wang, D., Khosla, A., Gargeya, R., Irshad, H., and Beck,
A. H. (2016). Deep learning for identifying metastatic
breast cancer. arXiv preprint arXiv:1606.05718.
VISAPP 2020 - 15th International Conference on Computer Vision Theory and Applications
512