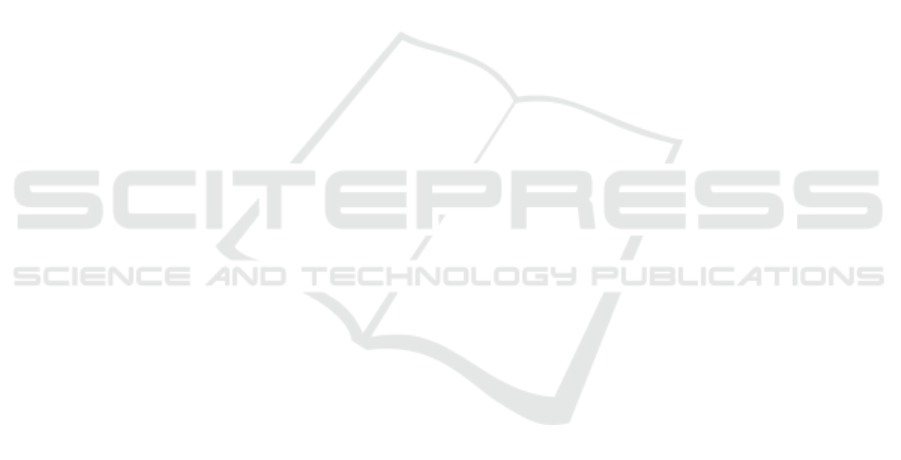
tial of the presented methodology. The most impor-
tant step would consist of creating larger and more
diversified datasets. It would be then possible to per-
form parallel training on two models with different ar-
chitectures: one specialized in multi-pose controlled
face photographs and the second for uncontrolled im-
ages or for images coming from a predefined type of
capture device. It was shown here that the network
training can be set up in the way that embeddings in
both models are forced to enclose to same set of proxy
points.
ACKNOWLEDGEMENTS
This work was supported by NCBiR grant DOB-
BIO7/18/02/2015. Computations made in this paper
would not be possible without the support of NVIDIA
Corporation that donated the GPU to author.
REFERENCES
Banerjee, S., Brogan, J., Krizaj, J., Bharati, A., Webster,
B. R., Struc, V., Flynn, P. J., and Scheirer, W. J.
(2018). To frontalize or not to frontalize: Do we really
need elaborate pre-processing to improve face recog-
nition? In 2018 IEEE Winter Conference on Applica-
tions of Computer Vision (WACV), pages 20–29.
Goldberger, J., Hinton, G. E., Roweis, S. T., and Salakhutdi-
nov, R. R. (2005). Neighbourhood components anal-
ysis. In Saul, L. K., Weiss, Y., and Bottou, L., editors,
Advances in Neural Information Processing Systems
17, pages 513–520. MIT Press.
Ho, C.-H., Morgado, P., Persekian, A., and Vasconcelos, N.
(2019). Pies: Pose invariant embeddings. In The IEEE
Conference on Computer Vision and Pattern Recogni-
tion (CVPR).
Huang, G. B., Ramesh, M., Berg, T., and Learned-Miller,
E. (2007). Labeled faces in the wild: A database for
studying face recognition in unconstrained environ-
ments. Technical Report 07-49, University of Mas-
sachusetts, Amherst.
King, D. E. (2009). Dlib-ml: A machine learning toolkit.
Journal of Machine Learning Research, 10:1755–
1758.
Maze, B., Adams, J., Duncan, J. A., Kalka, N., Miller, T.,
Otto, C., Jain, A. K., Niggel, W. T., Anderson, J., Ch-
eney, J., and Grother, P. (2018). IARPA Janus Bench-
mark - C: Face Dataset and Protocol. In 2018 Inter-
national Conference on Biometrics (ICB), pages 158–
165.
Movshovitz-Attias, Y., Toshev, A., Leung, T. K., Ioffe, S.,
and Singh, S. (2017). No fuss distance metric learning
using proxies. CoRR, abs/1703.07464.
Parkhi, O. M., Vedaldi, A., and Zisserman, A. (2015). Deep
face recognition. In British Machine Vision Confer-
ence.
Proenc¸a, H., Nixon, M., Nappi, M., Ghaleb, E.,
¨
Ozbulak,
G., Gao, H., Ekenel, H. K., Grm, K., Struc, V., Shi,
H., Zhu, X., Liao, S., Lei, Z., Li, S. Z., Gutfeter, W.,
Pacut, A., Brogan, J., Scheirer, W. J., Gonzalez-Sosa,
E., Vera-Rodriguez, R., Fierrez, J., Ortega-Garcia, J.,
Riccio, D., and De Maio, L. (2018). Trends and con-
troversies. IEEE Intelligent Systems, 33(3):41–67.
Quis-campi dataset (2015). Biometric Recognition in
Surveillance Environments. http://http://quiscampi.di.
ubi.pt/. Accessed: 2020-01-01.
Su, H., Maji, S., Kalogerakis, E., and Learned-Miller, E. G.
(2015). Multi-view convolutional neural networks for
3d shape recognition. In Proc. ICCV.
VISAPP 2020 - 15th International Conference on Computer Vision Theory and Applications
520