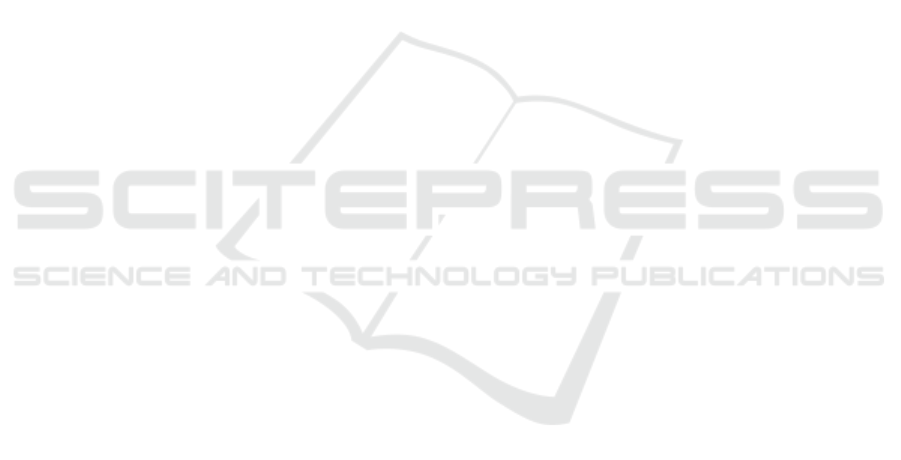
REFERENCES
Almeida, J. R., Guimar
˜
aes, J., and Oliveira, J. L. (2018).
Simplifying the digitization of clinical protocols for
diabetes management. In 2018 IEEE 31st Interna-
tional Symposium on Computer-Based Medical Sys-
tems (CBMS), pages 176–181. IEEE.
Almeida, J. R. and Oliveira, J. L. (2019). GenericCDSS-
a generic clinical decision support system. In 2019
IEEE 32nd International Symposium on Computer-
Based Medical Systems (CBMS), pages 186–191.
IEEE.
Antunes., R., Silva., J. F., Pereira., A., and Matos., S.
(2019). Rule-based and machine learning hybrid sys-
tem for patient cohort selection. In Proceedings of
the 12th International Joint Conference on Biomed-
ical Engineering Systems and Technologies - Volume
2: HEALTHINF,, pages 59–67. INSTICC, SciTePress.
Boxwala, A. A., Peleg, M., Tu, S., Ogunyemi, O., Zeng,
Q. T., Wang, D., Patel, V. L., Greenes, R. A., and
Shortliffe, E. H. (2004). Glif3: a representation for-
mat for sharable computer-interpretable clinical prac-
tice guidelines. Journal of biomedical informatics,
37(3):147–161.
Cohen, R., Elhadad, M., and Elhadad, N. (2013). Re-
dundancy in electronic health record corpora: analy-
sis, impact on text mining performance and mitigation
strategies. BMC Bioinformatics, 14(10).
Costa, C. M. A. (2004). Concepc¸
˜
ao, desenvolvimento e
avaliac¸
˜
ao de um modelo integrado de acesso a reg-
istos cl
´
ınicos electr
´
onicos. PhD thesis, University of
Aveiro.
Ferr
˜
ao, J. C., Janela, F., Oliveira, M. D., and Martins, H.
M. G. (2013). Using structured EHR data and SVM
to support ICD-9-CM coding. In 2013 IEEE Interna-
tional Conference on Healthcare Informatics, pages
511–516, Philadelphia, PA, USA. IEEE.
Ferr
˜
ao, J. C., Oliveira, M. D., Janela, F., and Martins, H.
M. G. (2016). Preprocessing structured clinical data
for predictive modeling and decision support. Applied
Clinical Informatics, 07(04):1135–1153.
HMS (2018). 2018 n2c2 - Track 1: Cohort Selection for
Clinical Trials.
HMS (2019a). 2019 n2c2 Shared-Task and Workshop,
Track2: n2c2/OHNLP Track on Family History Ex-
traction.
HMS (2019b). 2019 n2c2 Shared-Task and Workshop,
Track3: n2c2/UMass Track on Clinical Concept Nor-
malization.
Hripcsak, G. and Albers, D. J. (2012). Next-generation
phenotyping of electronic health records. Journal
of the American Medical Informatics Association,
20(1):117–121.
Inzucchi, S. E. (2006). Management of hyperglycemia in
the hospital setting. New England journal of medicine,
355(18):1903–1911.
Jensen, K., Soguero-Ruiz, C., Oyvind Mikalsen, K., Lind-
setmo, R.-O., Kouskoumvekaki, I., Girolami, M.,
Olav Skrovseth, S., and Augestad, K. M. (2017).
Analysis of free text in electronic health records for
identification of cancer patient trajectories. Scientific
Reports, 7(46226).
Johnson, A. E. W., Pollard, T. J., Shen, L., Lehman, L.-
w. H., Feng, M., Ghassemi, M., Moody, B., Szolovits,
P., Anthony Celi, L., and Mark, R. G. (2016). MIMIC-
III, a freely accessible critical care database. Scientific
Data, 3.
Katehakis, D. G. and Tsiknakis, M. (2006). Electronic
health record. In Wiley Encyclopedia of Biomedical
Engineering. Wiley.
Kim, Y., Rajan, K. B., Sims, S. A., Wroblewski, K. E., and
Reutrakul, S. (2014). Impact of glycemic variabil-
ity and hypoglycemia on adverse hospital outcomes
in non-critically ill patients. Diabetes research and
clinical practice, 103(3):437–443.
Manning, C. D., Surdeanu, M., Bauer, J., Finkel, J.,
Bethard, S. J., and McClosky, D. (2014). The Stan-
ford CoreNLP natural language processing toolkit. In
Association for Computational Linguistics (ACL) Sys-
tem Demonstrations, pages 55–60.
Matos, S. (2018). Configurable web-services for biomedi-
cal document annotation. Journal of cheminformatics,
10(1):68.
Miotto, R., Wang, F., Wang, S., Jiang, X., and Dudley, J. T.
(2017). Deep learning for healthcare: review, oppor-
tunities and challenges. Briefings in Bioinformatics.
NCCIH (2017). Clinical Practice Guidelines.
Neinstein, A., MacMaster, H. W., Sullivan, M. M., and
Rushakoff, R. (2014). A detailed description of the
implementation of inpatient insulin orders with a com-
mercial electronic health record system. Journal of
diabetes science and technology, 8(4):641–651.
Nelson, S. J., Zeng, K., Kilbourne, J., Powell, T., and
Moore, R. (2011). Normalized names for clinical
drugs: RxNorm at 6 years. Journal of the American
Medical Informatics Association, 18(4):441.
Neustein, A., Imambi, S. S., Rodrigues, M., Teixeira, A.,
and Ferreira, L. (2014). Application of text mining to
biomedical knowledge extraction: analyzing clinical
narratives and medical literature. In Text Mining of
Web-based Medical Content, pages 3–32. De Gruyter.
O’Connor, P. J., Sperl-Hillen, J. M., Rush, W. A., Johnson,
P. E., Amundson, G. H., Asche, S. E., Ekstrom, H. L.,
and Gilmer, T. P. (2011). Impact of electronic health
record clinical decision support on diabetes care: a
randomized trial. Annals of family medicine, 9(1):12–
21.
Pivovarov, R. and Elhadad, N. (2015). Automated meth-
ods for the summarization of electronic health records.
Journal of the American Medical Informatics Associ-
ation, 22(5):938–947.
Singh, H., Giardina, T. D., Meyer, A. N. D., Forjuoh, S. N.,
Reis, M. D., and Thomas, E. J. (2013). Types and
origins of diagnostic errors in primary care settings.
JAMA Internal Medicine, 173(6):418–425.
Stearns, M. Q., Price, C., Kent A. Spackman, and Wang,
A. Y. (2001). SNOMED clinical terms: overview of
the development process and project status. In Pro-
ceedings of the AMIA Symposium, pages 662–666,
Enhancing Decision-making Systems with Relevant Patient Information by Leveraging Clinical Notes
261