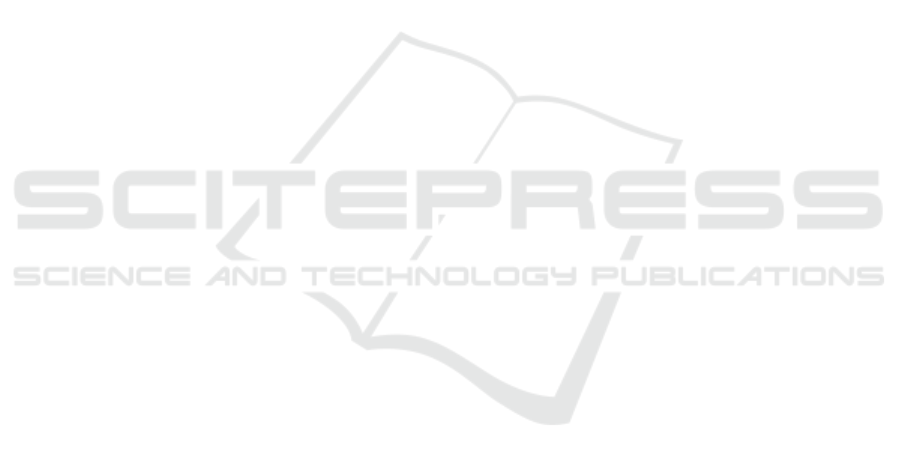
American Thematic Network on ICT Applications for
Smart Cities” (REF-518RT0559) and the NVIDIA
Corporation for the donation of the Titan Xp GPU.
The first author has been supported by Ecuador gov-
ernment under a SENESCYT scholarship contract
CZ05-000040-2018.
REFERENCES
Aanæs, H., Jensen, R. R., Vogiatzis, G., Tola, E., and Dahl,
A. B. (2016). Large-scale data for multiple-view stere-
opsis. International Journal of Computer Vision.
Bay, H., Tuytelaars, T., and Gool, L. J. V. (2006). SURF:
Speeded Up Robust Features. In Proceedings of the
9th European Conference on Computer Vision, Graz,
Austria, May 7-13, pages 404–417.
Calonder, M., Lepetit, V.,
¨
Ozuysal, M., Trzcinski, T.,
Strecha, C., and Fua, P. (2012). BRIEF: Computing
a local binary descriptor very fast. IEEE Trans. Pat-
tern Anal. Mach. Intell., 34(7):1281–1298.
Charco, J. L., Vintimilla, B. X., and Sappa, A. D. (2018).
Deep learning based camera pose estimation in multi-
view environment. In 2018 14th International Confer-
ence on Signal-Image Technology & Internet-Based
Systems (SITIS), pages 224–228. IEEE.
Clevert, D.-A., Unterthiner, T., and Hochreiter, S.
(2015). Fast and accurate deep network learning
by exponential linear units (elus). arXiv preprint
arXiv:1511.07289.
Dornaika, F.,
´
Alvarez, J. M., Sappa, A. D., and L
´
opez,
A. M. (2011). A new framework for stereo sen-
sor pose through road segmentation and registration.
IEEE Transactions on Intelligent Transportation Sys-
tems, 12(4):954–966.
Dosovitskiy, A., Ros, G., Codevilla, F., Lopez, A., and
Koltun, V. (2017). CARLA: An open urban driving
simulator. In Proceedings of the 1st Annual Confer-
ence on Robot Learning, pages 1–16.
En, S., Lechervy, A., and Jurie, F. (2018). Rpnet: an end-
to-end network for relative camera pose estimation.
In Proceedings of the European Conference on Com-
puter Vision (ECCV).
Hartley, R. I. (1994). Self-calibration from multiple views
with a rotating camera. In European Conference on
Computer Vision, pages 471–478. Springer.
He, K., Zhang, X., Ren, S., and Sun, J. (2016). Deep resid-
ual learning for image recognition. In Proceedings of
the IEEE conference on computer vision and pattern
recognition, pages 770–778.
Iyer, G., Ram, R. K., Murthy, J. K., and Krishna, K. M.
(2018). Calibnet: Geometrically supervised extrinsic
calibration using 3d spatial transformer networks. In
2018 IEEE/RSJ International Conference on Intelli-
gent Robots and Systems (IROS). IEEE.
Jalal, M., Spjut, J., Boudaoud, B., and Betke, M. (2019).
Sidod: A synthetic image dataset for 3d object pose
recognition with distractors. In Proceedings of the
IEEE Conference on Computer Vision and Pattern
Recognition Workshops, pages 0–0.
Kamnitsas, K., Ledig, C., Newcombe, V. F., Simpson,
J. P., Kane, A. D., Menon, D. K., Rueckert, D., and
Glocker, B. (2017). Efficient multi-scale 3d cnn with
fully connected crf for accurate brain lesion segmen-
tation. Medical image analysis, 36:61–78.
Kendall, A. and Cipolla, R. (2017). Geometric loss func-
tions for camera pose regression with deep learning.
In Proceedings of the IEEE Conference on Computer
Vision and Pattern Recognition, pages 5974–5983.
Kendall, A., Grimes, M., and Cipolla, R. (2015). Posenet: A
convolutional network for real-time 6-dof camera re-
localization. In Proceedings of the IEEE international
conference on computer vision, pages 2938–2946.
Lin, Y., Liu, Z., Huang, J., Wang, C., Du, G., Bai, J., and
Lian, S. (2019). Deep global-relative networks for
end-to-end 6-dof visual localization and odometry. In
Pacific Rim International Conference on Artificial In-
telligence, pages 454–467. Springer.
Liu, R., Zhang, H., Liu, M., Xia, X., and Hu, T. (2009).
Stereo cameras self-calibration based on sift. In 2009
International Conference on Measuring Technology
and Mechatronics Automation, volume 1.
Lowe, D. G. (1999). Object recognition from local scale-
invariant features. In Proceedings of the IEEE In-
ternational Conference on Computer Vision, Kerkyra,
Greece, September 20-27, pages 1150–1157.
Moulon, P., Monasse, P., Marlet, R., and Others (2016).
Openmvg. an open multiple view geometry library.
https://github.com/openMVG/openMVG.
Onkarappa, N. and Sappa, A. D. (2015). Synthetic se-
quences and ground-truth flow field generation for al-
gorithm validation. Multimedia Tools and Applica-
tions, 74(9):3121–3135.
Rivadeneira, R. E., Su
´
arez, P. L., Sappa, A. D., and Vin-
timilla, B. X. (2019). Thermal image superresolution
through deep convolutional neural network. In Inter-
national Conference on Image Analysis and Recogni-
tion, pages 417–426. Springer.
Sappa, A., Ger
´
onimo, D., Dornaika, F., and L
´
opez, A.
(2006). On-board camera extrinsic parameter estima-
tion. Electronics Letters, 42(13):745–747.
Schonberger, J. L. and Frahm, J.-M. (2016). Structure-
from-motion revisited. In Proceedings of the IEEE
Conference on Computer Vision and Pattern Recogni-
tion, pages 4104–4113.
Shalnov, E. and Konushin, A. (2017). Convolutional neural
network for camera pose estimation from object detec-
tions. International Archives of the Photogrammetry,
Remote Sensing & Spatial Information Sciences, 42.
Shotton, J., Glocker, B., Zach, C., Izadi, S., Criminisi, A.,
and Fitzgibbon, A. (2013). Scene coordinate regres-
sion forests for camera relocalization in rgb-d images.
In 2013 IEEE Conference on Computer Vision and
Pattern Recognition, pages 2930–2937.
Wang, J., Yang, Y., Mao, J., Huang, Z., Huang, C., and Xu,
W. (2016). Cnn-rnn: A unified framework for multi-
label image classification. In Proceedings of the IEEE
conference on computer vision and pattern recogni-
tion, pages 2285–2294.
Transfer Learning from Synthetic Data in the Camera Pose Estimation Problem
505