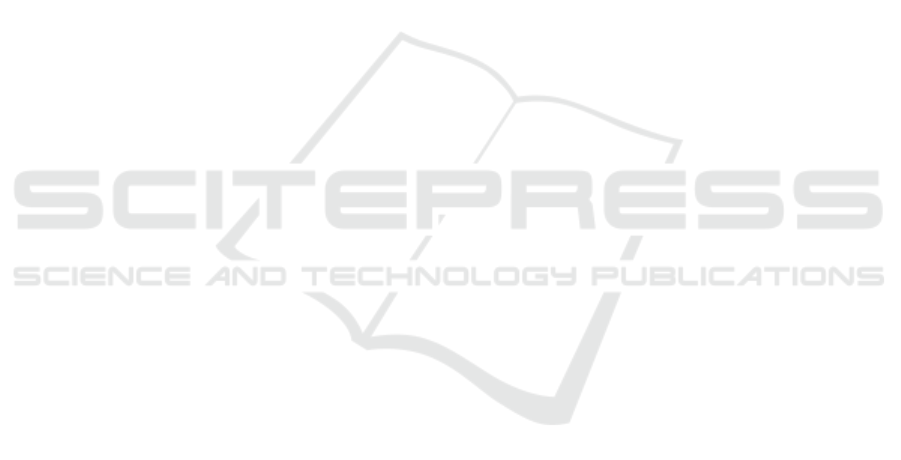
Lambin, P., Rios-Velazquez, E., Leijenaar, R., et al. (2012).
Radiomics: Extracting more information from medi-
cal images using advanced feature analysis. European
Journal of Cancer, 48(4):441–446.
Law, M., Yang, S., and Wang, H. a. (2003). Glioma grading:
Sensitivity, specificity, and predictive values of perfu-
sion MR imaging and proton MR spectroscopic imag-
ing compared with conventional MR imaging. Amer-
ican Journal of Neuroradiology, 24(10):1989–1998.
Lee, G., Lee, H. Y., Ko, E. S., et al. (2017). Radiomics
and imaging genomics in precision medicine. Precis
Future Med, 1(1):10–31.
Louis, D. N., Perry, A., Reifenberger, G., Von Deimling,
A., Figarella-Branger, D., Cavenee, W. K., Ohgaki,
H., Wiestler, O. D., Kleihues, P., and Ellison, D. W.
(2016). The 2016 World Health Organization clas-
sification of tumors of the central nervous system: a
summary. Acta neuropathologica, 131(6):803–820.
Luengo, A., Gui, D. Y., and Vander Heiden, M. G. (2017).
Targeting metabolism for cancer therapy. Cell chemi-
cal biology, 24(9):1161–1180.
Manzo, M. (2019). Kgearsrg: Kernel graph embedding on
attributed relational sift-based regions graph. Machine
Learning and Knowledge Extraction, 1(3):962–973.
Maratea, A., Petrosino, A., and Manzo, M. (2014). Ad-
justed f-measure and kernel scaling for imbalanced
data learning. Information Sciences, 257:331–341.
Menze, B. H., Jakab, A., Bauer, S., et al. (2015). The mul-
timodal brain tumor image segmentation benchmark
(brats). IEEE Trans Med Imaging, 34(10):1993–2024.
Paulovich, F. V., Nonato, L. G., Minghim, R., et al. (2008).
Least square projection: A fast high-precision mul-
tidimensional projection technique and its application
to document mapping. IEEE Trans Vis Comput Graph,
14(3):564–575.
Peeken, J. C., Bernhofer, M., Wiestler, B., et al. (2018).
Radiomics in radiooncology - challenging the medical
physicist. Physica Medica, 48:27–36.
Ranjbar, S. and Mitchell, J. R. (2017). Chapter 8 - an intro-
duction to radiomics: An evolving cornerstone of pre-
cision medicine. In Depeursinge, A., Al-Kadi, O. S.,
and Mitchell, J., editors, Biomedical Texture Analysis,
pages 223 – 245. Academic Press.
Rauber, P. E., Falcao, A. X., and Telea, A. C. (2018). Pro-
jections as visual aids for classification system design.
Information Visualization, 17(4):282–305.
Russo, D., Della Ragione, F., Rizzo, R., Sugiyama, E.,
Scalabr
`
ı, F., Hori, K., Capasso, S., Sticco, L., Fior-
iniello, S., De Gregorio, R., et al. (2018). Glycosph-
ingolipid metabolic reprogramming drives neural dif-
ferentiation. The EMBO journal, 37(7):e97674.
Sala, E. et al. (2017). Unravelling tumour heterogene-
ity using next-generation imaging: Radiomics, ra-
diogenomics, and habitat imaging. Clin. Radiol.,
72(1):3–10.
Santos, M. S., Soares, J. P., Abreu, P. H., et al. (2018).
Cross-validation for imbalanced datasets: Avoiding
overoptimistic and overfitting approaches [research
frontier]. IEEE Comp. Int. Mag., 13(4):59–76.
Smedley, N. F. and Hsu, W. (2018). Using deep neural net-
works for radiogenomic analysis. In 2018 IEEE 15th
Int. Symposium on Biomedical Imaging (ISBI 2018),
pages 1529–1533.
Soeda, A., Hara, A., Kunisada, T., Yoshimura, S.-i., Iwama,
T., and Park, D. M. (2015). The evidence of glioblas-
toma heterogeneity. Scientific reports, 5:7979.
Sun, Q., Li, S., Wang, Y., Peng, H., Zhang, X., Zheng, Y.,
Li, C., Li, L., Chen, R., Chen, X., et al. (2018). Phos-
phoglyceric acid mutase-1 contributes to oncogenic
mTOR-mediated tumor growth and confers non-small
cell lung cancer patients with poor prognosis. Cell
Death & Differentiation, 25(6):1160.
Togao, O., Hiwatashi, A., Yamashita, K., et al. (2016).
Differentiation of high-grade and low-grade diffuse
gliomas by intravoxel incoherent motion MR imaging.
Neuro-Oncology, 18(1):132–141.
van der Maaten, L. and Hinton, G. (2008). Visualizing data
using t-SNE. Journal of Machine Learning Research,
9:2579–2605.
Vapnik, V. (1995). The Nature of Statistical Learning The-
ory. Springer-Verlag.
Wang, L., Wang, Y., and Chang, Q. (2016). Feature se-
lection methods for big data bioinformatics: A survey
from the search perspective. Methods, 111:21–31. Big
Data Bioinformatics.
Wu, X., Kumar, V., Ross Quinlan, J., et al. (2007). Top 10
algorithms in data mining. Knowl. Inf. Syst., 14(1):1–
37.
Zacharaki, E. I., Wang, S., Chawla, S., et al. (2009). Classi-
fication of brain tumor type and grade using MRI tex-
ture and shape in a machine learning scheme. Magn
Reson Med, 62:a609–1618.
Zinn, P., Majadan, B., et al. (2011). Radiogenomic map-
ping of edema/cellular invasion MRI-phenotypes in
glioblastoma multiforme. PLoS ONE, 6(10).
BIOIMAGING 2020 - 7th International Conference on Bioimaging
92