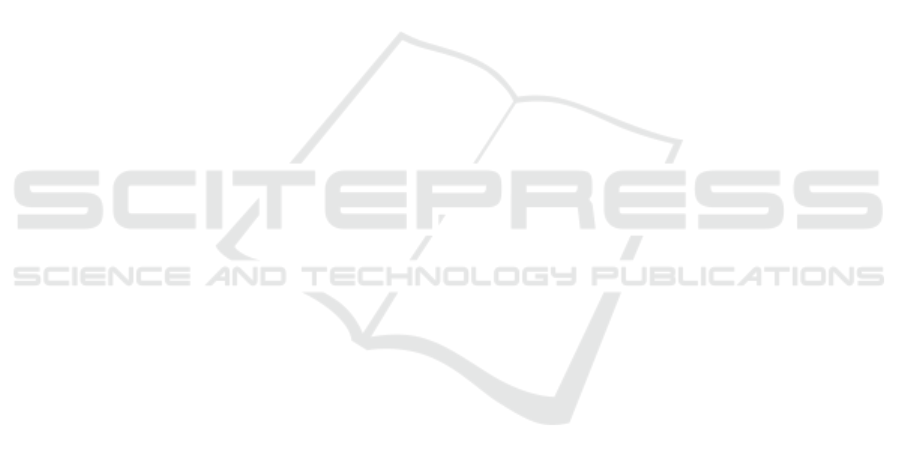
5 CONCLUSIONS
Bio-inspired fixational eye movements can transform
a static scene into a spatiotemporal input luminance
signal to the event-based camera. As a consequence,
the low temporal frequency power of a static scene
is shifted into a range that the DVS can properly de-
tect. Besides preventing “perceptual fading” of static
scenes, we show that FEMs can play a central role in
event-based vision by providing an efficient strategy
for acquiring and processing information from nat-
ural images, both enhancing the perception of fine
spatial details in the scene, and facilitating or im-
proving the extraction of important features. Par-
ticularly, due to camera motion, edges in the visual
scene will provoke highly time-correlated activity of
nearby pixels. Due to the randomness of such motion,
events with both polarities can be elicited over time
in each pixel as a result of a same spatial luminance
discontinuity. Therefore, synchronized events with
both polarities eventually encode the spatial structure
of the underlying static image. The push-pull con-
figuration, at which the network operates, exploits
the distinction between events’ polarities, inducing
appropriate excitation or inhibition of ON and OFF
events, for optimizing detection performances. The
whole artificial neural architecture proposed is fully
bio-inspired, both at single unit (neuron model) and at
network level, and is entirely conceived to satisfy the
constrains imposed by ultra low-power mixed signal
analog-digital neuromorphic processors for a future
hardware implementation.
ACKNOWLEDGEMENTS
This project has received funding from the European
Research Council under the Grant Agreement No.
724295 (NeuroAgents).
REFERENCES
Ahissar, E. and Arieli, A. (2001). Figuring space by time.
Neuron, 32(2):185–201.
Bartolozzi, C. and Indiveri, G. (2007). Synaptic dynamics
in analog vlsi. Neural computation, 19:2581–2603.
Blakemore, C., Carpenter, R. H., and Georgeson, M. A.
(1970). Lateral inhibition between orientation detec-
tors in the human visual system. Nature, 228:37–39.
Ditchburn, R. and Ginsborg, B. (1952). Vision with a stabi-
lized retinal image. Nature, 170(4314):36.
Field, D. J. (1987). Relations between the statistics of natu-
ral images and the response properties of cortical cells.
Journal of the Opt. Soc. of America, 4:2379–2394.
Gollisch, T. and Meister, M. (2008). Rapid neural coding
in the retina with relative spike latencies. Science,
319(5866):1108–1111.
Greschner, M., Bongard, M., Rujan, P., and Ammerm
¨
uller,
J. (2002). Retinal ganglion cell synchronization by
fixational eye movements improves feature estima-
tion. Nature neuroscience, 5(4):341.
Hirsch, J. A. and Martinez, L. M. (2006). Circuits that
build visual cortical receptive fields. Trends in neu-
rosciences, 29(1):30–39.
Hubel, D. H. and Wiesel, T. N. (1974). Sequence regular-
ity and geometry of orientation columns in the mon-
key striate cortex. Journal of Comparative Neurology,
158(3):267–293.
Indiveri, G., Linares-Barranco, B., Hamilton, T. J.,
Van Schaik, A., Etienne-Cummings, R., Delbruck, T.,
Liu, S.-C., Dudek, P., H
¨
afliger, P., Renaud, S., et al.
(2011). Neuromorphic silicon neuron circuits. Fron-
tiers in neuroscience, 5:73.
Kuang, X., Poletti, M., Victor, J. D., and Rucci, M. (2012).
Temporal encoding of spatial information during ac-
tive visual fixation. Current Biology, 22(6):510–514.
Land, M. (2019). Eye movements in man and other animals.
Vision research, 162:1–7.
Lichtsteiner, P., Posch, C., and Delbruck, T. (2008). A
128×128 120 dB 15µs latency asynchronous tempo-
ral contrast vision sensor. IEEE journal of solid-state
circuits, 43(2):566–576.
Maass, W. (1997). Networks of spiking neurons: the third
generation of neural network models. Neural net-
works, 10(9):1659–1671.
Martinez-Conde, S. and Macknik, S. L. (2008). Fixational
eye movements across vertebrates: comparative dy-
namics, physiology, and perception. Journal of Vision,
8:1–18.
Moradi, S., Qiao, N., Stefanini, F., and Indiveri, G. (2017).
A scalable multicore architecture with heterogeneous
memory structures for dynamic neuromorphic asyn-
chronous processors (DYNAPs). IEEE transactions
on biomedical circuits and systems, 12(1):106–122.
Orchard, G., Jayawant, A., Cohen, G. K., and Thakor, N.
(2015). Converting static image datasets to spiking
neuromorphic datasets using saccades. Frontiers in
neuroscience, 9:437.
Riggs, L. and Ratliff, F. (1952). The effects of counteracting
the normal movements of the eye. 42:872–873.
Rucci, M., Ahissar, E., and Burr, D. (2018). Temporal
coding of visual space. Trends in cognitive sciences,
22(10):883–895.
Rucci, M. and Victor, J. D. (2015). The unsteady eye: an
information-processing stage, not a bug. Trends in
neurosciences, 38(4):195–206.
Dynamic Detectors of Oriented Spatial Contrast from Isotropic Fixational Eye Movements
681