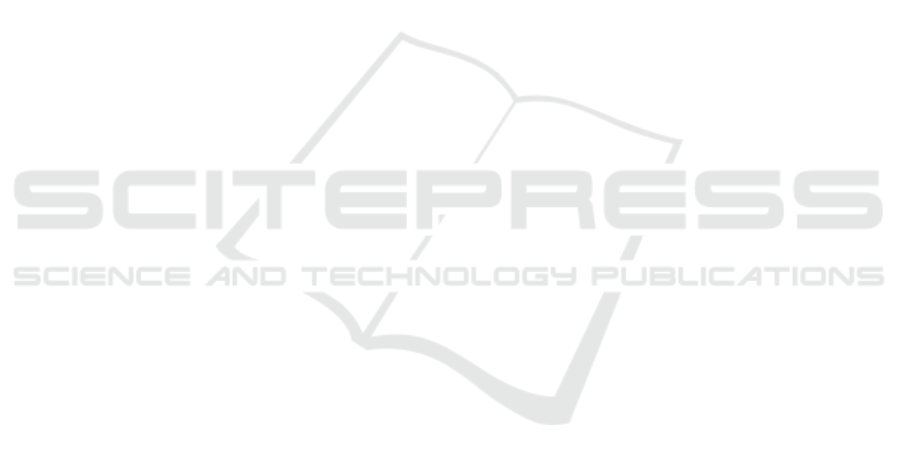
icolaou slide, are obtained between 10 to 15 thousand
images with a 40x magnification and, as in a realis-
tic environment there is too many of these slides for
analysis, in order that the structure here proposed has
functional applicability in this environment, the clas-
sification of an image must happen quickly. Making
elementary techniques stand out in relation to robust
techniques.
5 CONCLUSION
Precisely classifying cell nuclei in real images of Pap
smear is a necessary condition to provide more ac-
curate and reliable diagnostics. Since in this type
of image the information about nuclear and cytoplas-
mic boundaries is complex and computationally in-
feasible, this paper presents a structure for the clas-
sification of real cervical cytology images from the
extraction of non-geometric textural features without
the use of learning from neural networks or from seg-
mentation algorithms.
The proposed structure has realistic application to
help in the diagnosis and screening of cervical cancer.
A supervised learning classifier, SVM, was used for
classification and system performance was measured
with actual cervical cytology images, created from the
Pap smear, provided by a private searchable imaging
database.
The results obtained by our experiments indicate
the optimum performance of the proposed system in
the process of categorization of lesions present in Pap
smears images, presenting high precision and low
false negative index. This implies that the efficient
characterization of an actual cervical cytology image
is feasible only with the use of non-geometric extrac-
tion features.
In the medical and biological context, the pro-
posed structure serves as a preliminary Pap smears
classifier that determines the likelihood of lesions in
an image and signals them to be analyzed by a cy-
topathologist when necessary and provide a definitive
diagnosis of more practical, efficient and with quality
assurance. In addition, correlating biology and com-
putation, pertinent conclusions can be inferred regard-
ing the rigidity or flexibility required for a system to
have effective applicability in real environments.
6 FUTURE WORKS
As future works we intend to investigate the efficiency
of the proposed system when applied in a larger num-
ber of real images not cataloged and to construct a
system that allows the return of these regions to the
analysis of the cytopathologist. Another important
factor to be examined are the sets of descriptors, per-
forming a more systematic investigation for the in-
clusion of information that allows the increase of the
precision of the method.
ACKNOWLEDGEMENTS
The authors thanks Conselho Nacional de De-
senvolvimento Cient
´
ıfico e Tecnol
´
ogico (PIBITI-
CNPq), Universidade Federal de Ouro Preto (UFOP),
Fundac¸
˜
ao de Amparo
`
a Pesquisa do Estado de Mi-
nas Gerais (PPSUS-FAPEMIG/APQ-03740-17), the
Moore-Sloan Foundation, and Office of Science,
of the U.S. Department of Energy under Contract
No. DE-AC02-05CH11231 for also supporting this
research. Any opinion, findings, and conclusions or
recommendations expressed in this material are those
of the authors and do not necessarily reflect the views
of the Department of Energy or the University of Cal-
ifornia.
REFERENCES
Arajo, F. H., Silva, R. R., Ushizima, D. M., Rezende, M. T.,
Carneiro, C. M., Bianchi, A. G. C., and Medeiros,
F. N. (2019). Deep learning for cell image segmen-
tation and ranking. Computerized Medical Imaging
and Graphics, 72:13 – 21.
Bishop, C. M. (2006). Pattern Recognition and Ma-
chine Learning (Information Science and Statistics).
Springer-Verlag, Berlin, Heidelberg.
Haralick, R. M. (1979). Statistical and structural approaches
to texture. Proceedings of the IEEE, 67(5):786–804.
Jantzen, J., Norup, J., Dounias, G., and Bjerregaard, B.
(2005). Pap-smear benchmark data for pattern clas-
sification. Nature Inspired Smart Information Systems
(NiSIS).
Lakshmi, G. K. and Krishnaveni, K. (2014). Multiple fea-
ture extraction from cervical cytology images by gaus-
sian mixture model. In 2014 World Congress on Com-
puting and Communication Technologies, pages 309–
311.
Lorenzo-Ginori, J. V., Curbelo-Jardines, W., L
´
opez-
Cabrera, J. D., and Huergo-Su
´
arez, S. B. (2013). Cer-
vical cell classification using features related to mor-
phometry and texture of nuclei. In Ruiz-Shulcloper,
J. and Sanniti di Baja, G., editors, Progress in Pat-
tern Recognition, Image Analysis, Computer Vision,
and Applications, pages 222–229, Berlin, Heidelberg.
Springer Berlin Heidelberg.
Mariarputham, E. J. and Stephen, A. (2015). Nominated
texture based cervical cancer classification. In Com-
putational and Mathematical Methods in Medicine.
Automatic Classification of Cervical Cell Patches based on Non-geometric Characteristics
851