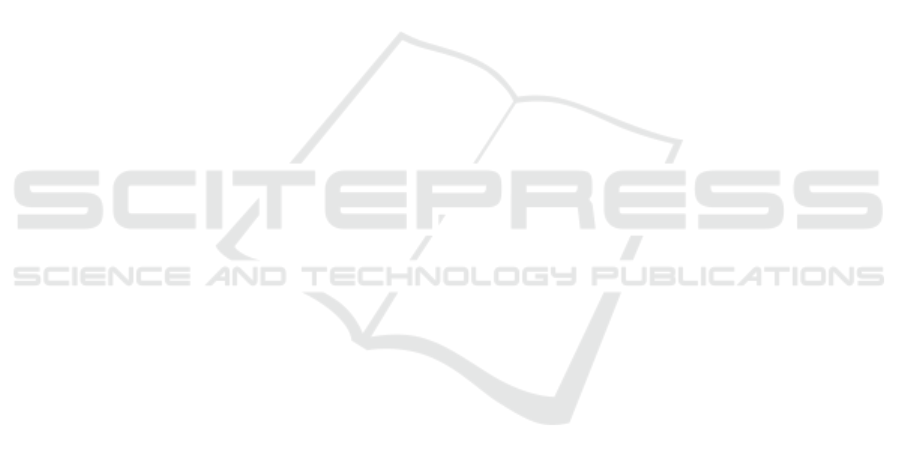
simplified 2D scenario which can be easily gener-
alised to the more complete and realistic case of three-
dimensional breast.
In the framework of imaging techniques,
microwave-based tomographic breast imaging may
represent a valid alternative or a complementary
medical exam, since it is safe compared to the
standard mammography and less expensive rather
than magnetic resonance imaging.
For the generation of the training data set, a
randomly-shaped breast profile generator has been
proposed whose tissues electric parameters were se-
lected according to proper statistical distributions as
reported in the scientific literature (Lazebnik et al.,
2007). Regarding the network design, a three fully-
connected layers network architecture was proposed
and compared with a classical inversion scheme
(DBIM). It is worth to underline the capability of the
proposed approach to retrieve the imaginary part of
complex permittivity with a good accuracy compared
with classical approaches, as well as the capability of
correctly estimating the thickness of the skin layer.
Future work will focus on testing new network ar-
chitectures and on the proper design of the training
data set.
REFERENCES
Aghababaee, H., Amini, J., and Tzeng, Y.-C. (2013). Im-
proving change detection methods of sar images using
fractals. Scientia Iranica, 20(1):15–22.
Ahsan, S., Guo, Z., Miao, Z., Sotiriou, I., Koutsoupidou,
M., Kallos, E., Palikaras, G., and Kosmas, P. (2018).
Design and experimental validation of a multiple-
frequency microwave tomography system employing
the dbim-twist algorithm. Sensors, 18(10):3491.
Ambrosanio, M., Kosmasy, P., and Pascazio, V. (2016). An
adaptive multi-threshold iterative shrinkage algorithm
for microwave imaging applications. In 2016 10th
European Conference on Antennas and Propagation
(EuCAP), pages 1–3. IEEE.
Ambrosanio, M. and Pascazio, V. (2015). Numerical analy-
sis of a compressive sensing approach for ground pen-
etrating radar applications. In 2015 16th International
Radar Symposium (IRS), pages 410–415. IEEE.
Ashtari, A., Noghanian, S., Sabouni, A., Aronsson, J.,
Thomas, G., and Pistorius, S. (2010). Using a-priori
information for regularization in breast microwave
image reconstruction. IEEE Transactions on Biomed-
ical Engineering, 57(9):2197–2208.
Bevacqua, M. T., Bellizzi, G. G., Crocco, L., and Iser-
nia, T. (2019). A method for quantitative imaging
of electrical properties of human tissues from only
amplitude electromagnetic data. Inverse Problems,
35(2):025006.
Bevacqua, M. T. and Isernia, T. (2018). Boundary indica-
tor for aspect limited sensing of hidden dielectric ob-
jects. IEEE Geoscience and Remote Sensing Letters,
15(6):838–842.
Caorsi, S. and Gamba, P. (1999). Electromagnetic detection
of dielectric cylinders by a neural network approach.
IEEE transactions on geoscience and remote sensing,
37(2):820–827.
Catapano, I., Crocco, L., Di Donato, L., Angiulli, G., Is-
ernia, T., Morabito, A., Tringali, S., and Bucci, O.
(2010). Guidelines for effective microwave breast
imaging: a numerical assessment against 3d anthro-
pomorphic phantoms. In Proceedings of the Fourth
European Conference on Antennas and Propagation,
pages 1–5. IEEE.
Colton, D. and Kress, R. (2012). Inverse acoustic and elec-
tromagnetic scattering theory, volume 93. Springer
Science & Business Media.
Estatico, C., Fedeli, A., Pastorino, M., and Randazzo, A.
(2016). A banach space regularization approach for
multifrequency microwave imaging. International
Journal of Antennas and Propagation, 2016.
Hornik, K., Stinchcombe, M., and White, H. (1990). Uni-
versal approximation of an unknown mapping and
its derivatives using multilayer feedforward networks.
Neural networks, 3(5):551–560.
Isernia, T., Pascazio, V., and Pierri, R. (1997). A non-
linear estimation method in tomographic imaging.
IEEE Transactions on Geoscience and Remote Sens-
ing, 35(4):910–923.
Lazebnik, M., Popovic, D., McCartney, L., Watkins, C. B.,
Lindstrom, M., Harter, J., Sewall, S., Ogilvie, T.,
Magliocco, A., Breslin, T. M., et al. (2007). A large-
scale study of the ultrawideband microwave dielec-
tric properties of normal, benign and malignant breast
tissues obtained from cancer surgeries. Physics in
Medicine & Biology, 52(20):6093.
Lucas, A., Iliadis, M., Molina, R., and Katsaggelos, A. K.
(2018). Using deep neural networks for inverse prob-
lems in imaging: beyond analytical methods. IEEE
Signal Processing Magazine, 35(1):20–36.
Massa, A., Donelli, M., Pastorino, M., and Rosani, A.
(2005). Microwave imaging for non-destructive evalu-
ation of civil structures. Insight-Non-Destructive Test-
ing and Condition Monitoring, 47(1):11–14.
Persico, R., Ludeno, G., Soldovieri, F., De Coster, A., and
Lambot, S. (2018). Improvement of ground penetrat-
ing radar (gpr) data interpretability by an enhanced
inverse scattering strategy. Surveys in Geophysics,
39(6):1069–1079.
Schertzer, D. and Lovejoy, S. (1989). Nonlinear variabil-
ity in geophysics: Multifractal simulations and analy-
sis. In Fractals’ Physical Origin and Properties, pages
49–79. Springer.
Shah, P. and Moghaddam, M. (2017). Super resolution for
microwave imaging: A deep learning approach. In
2017 IEEE International Symposium on Antennas and
Propagation & USNC/URSI National Radio Science
Meeting, pages 849–850. IEEE.
Vitale, S., Ferraioli, G., and Pascazio, V. (2019). A new
ratio image based cnn algorithm for sar despeckling.
arXiv preprint arXiv:1906.04111.
BIOIMAGING 2020 - 7th International Conference on Bioimaging
208