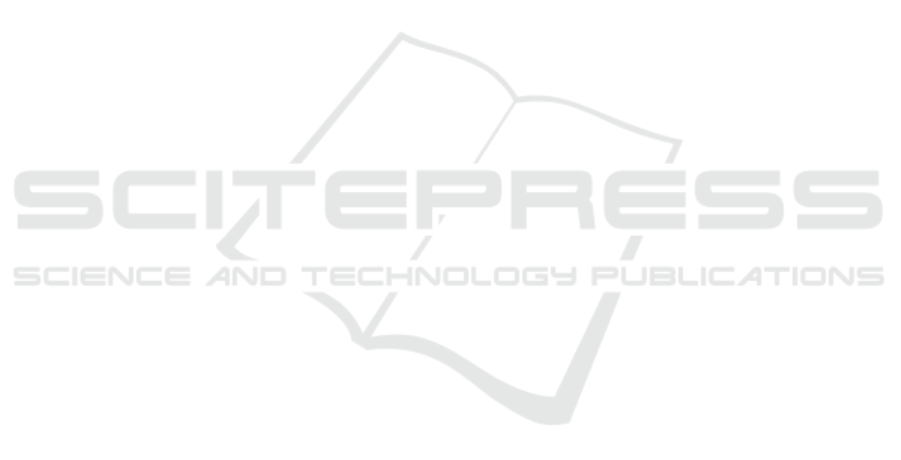
dimethylsiloxane (pdms) microchannels. Microma-
chines, 9(8):384.
Bu
ˇ
s
´
ık, M. and Cimr
´
ak, I. (2017). The calibration of fluid-
object interaction in immersed boundary method. In
EPJ Web of Conferences, volume 143, page 02013.
EDP Sciences.
Bu
ˇ
s
´
ık, M., Jan
ˇ
cigov
´
a, I., T
´
othov
´
a, R., and Cimr
´
ak, I.
(2016). Simulation study of rare cell trajectories and
capture rate in periodic obstacle arrays. Journal of
Computational Science, 17:370–376.
Chen, J., Li, J., and Sun, Y. (2012). Microfluidic approaches
for cancer cell detection, characterization, and separa-
tion. Lab on a Chip, 12(10):1753–1767.
Chovanec, M., Bachrat
´
y, H., Jasen
ˇ
c
´
akov
´
a, K., and
Bachrat
´
a, K. (2019). Convolutional neural networks
for red blood cell trajectory prediction in simulation
of blood flow. In International Work-Conference on
Bioinformatics and Biomedical Engineering, pages
284–296. Springer.
Cimr
´
ak, I., Gusenbauer, M., and Jan
ˇ
cigov
´
a, I. (2014).
An espresso implementation of elastic objects im-
mersed in a fluid. Computer Physics Communications,
185(3):900–907.
Cimr
´
ak, I. and Jan
ˇ
cigov
´
a, I. (2018). Computational Blood
Cell Mechanics: Road Towards Models and Biomedi-
cal Applications. CRC Press.
Dao, M., Lim, C. T., and Suresh, S. (2003). Mechanics of
the human red blood cell deformed by optical tweez-
ers. Journal of the Mechanics and Physics of Solids,
51(11-12):2259–2280.
Gleghorn, J. P., Pratt, E. D., Denning, D., Liu, H., Bander,
N. H., Tagawa, S. T., Nanus, D. M., Giannakakou,
P. A., and Kirby, B. J. (2010). Capture of circulat-
ing tumor cells from whole blood of prostate cancer
patients using geometrically enhanced differential im-
munocapture (gedi) and a prostate-specific antibody.
Lab on a chip, 10(1):27–29.
Hou, H. W., Li, Q., Lee, G., Kumar, A., Ong, C., and Lim,
C. T. (2009). Deformability study of breast cancer
cells using microfluidics. Biomedical microdevices,
11(3):557–564.
Huang, L. R., Cox, E. C., Austin, R. H., and Sturm,
J. C. (2004). Continuous particle separation
through deterministic lateral displacement. Science,
304(5673):987–990.
Inglis, D. W., Davis, J. A., Austin, R. H., and Sturm,
J. C. (2006). Critical particle size for fractionation
by deterministic lateral displacement. Lab on a Chip,
6(5):655–658.
Kaj
´
anek, F. and Cimr
´
ak, I. (2019). Evaluation of detec-
tion of red blood cells using convolutional neural net-
works. In 2019 International Conference on Informa-
tion and Digital Technologies (IDT), pages 198–202.
IEEE.
Koval
ˇ
c
´
ıkov
´
a, K. (2019). Discretization of simulation
elements–feasibility limits and accuracy of results. In
2019 Proceedings of MIST Conference on Mathemat-
ics in Science and Technologies (2019, In press).
Koval
ˇ
c
´
ıkov
´
a, K., Slav
´
ık, M., Bachrat
´
a, K., Bachrat
´
y, H.,
and Bohinikov
´
a, A. (2018). Volumetric flow rate in
simulations of microfluidic devices. In EPJ Web of
Conferences, volume 180, page 02046. EDP Sciences.
Lima, R., Ishikawa, T., Imai, Y., Takeda, M., Wada, S., and
Yamaguchi, T. (2008). Radial dispersion of red blood
cells in blood flowing through glass capillaries: the
role of hematocrit and geometry. Journal of biome-
chanics, 41(10):2188–2196.
Nagrath, S., Sequist, L. V., Maheswaran, S., Bell, D. W., Ir-
imia, D., Ulkus, L., Smith, M. R., Kwak, E. L., Digu-
marthy, S., Muzikansky, A., et al. (2007). Isolation
of rare circulating tumour cells in cancer patients by
microchip technology. Nature, 450(7173):1235.
Pinho, D., Yaginuma, T., and Lima, R. (2013). A microflu-
idic device for partial cell separation and deformabil-
ity assessment. BioChip Journal, 7(4):367–374.
Qi, Q. M. and Shaqfeh, E. S. (2017). Theory to predict
particle migration and margination in the pressure-
driven channel flow of blood. Physical Review Fluids,
2(9):093102.
Sajeesh, P. and Sen, A. K. (2014). Particle separation and
sorting in microfluidic devices: a review. Microflu-
idics and nanofluidics, 17(1):1–52.
Schneider, C. A., Rasband, W. S., and Eliceiri, K. W.
(2012). Nih image to imagej: 25 years of image anal-
ysis. Nature methods, 9(7):671.
Shields IV, C. W., Reyes, C. D., and L
´
opez, G. P. (2015).
Microfluidic cell sorting: a review of the advances in
the separation of cells from debulking to rare cell iso-
lation. Lab on a Chip, 15(5):1230–1249.
Slav
´
ık, M., Bachrat
´
a, K., Bachrat
´
y, H., and Koval
ˇ
c
´
ıkov
´
a,
K. (2017). The sensitivity of the statistical charac-
teristics to the selected parameters of the simulation
model in the red blood cell flow simulations. In 2017
International Conference on Information and Digital
Technologies (IDT), pages 344–349. IEEE.
Smie
ˇ
skov
´
a, M. and Bachrat
´
a, K. (2019). Validation of bulk
properties of red blood cells in simulations. In 2019
International Conference on Information and Digital
Technologies (IDT), pages 417–423. IEEE.
Warkiani, M. E., Guan, G., Luan, K. B., Lee, W. C., Bhagat,
A. A. S., Chaudhuri, P. K., Tan, D. S.-W., Lim, W. T.,
Lee, S. C., Chen, P. C., et al. (2014). Slanted spiral
microfluidics for the ultra-fast, label-free isolation of
circulating tumor cells. Lab on a Chip, 14(1):128–
137.
Weik, F., Weeber, R., Szuttor, K., Breitsprecher, K.,
de Graaf, J., Kuron, M., Landsgesell, J., Menke, H.,
Sean, D., and Holm, C. (2019). Espresso 4.0 – an
extensible software package for simulating soft mat-
ter systems. The European Physical Journal Special
Topics, 227(14):1789–1816.
Zhang, Z. and Nagrath, S. (2013). Microfluidics and can-
cer: are we there yet? Biomedical microdevices,
15(4):595–609.
Assessment of Computational Cell Model Benefits for Optimization of Microfluidic Devices
287