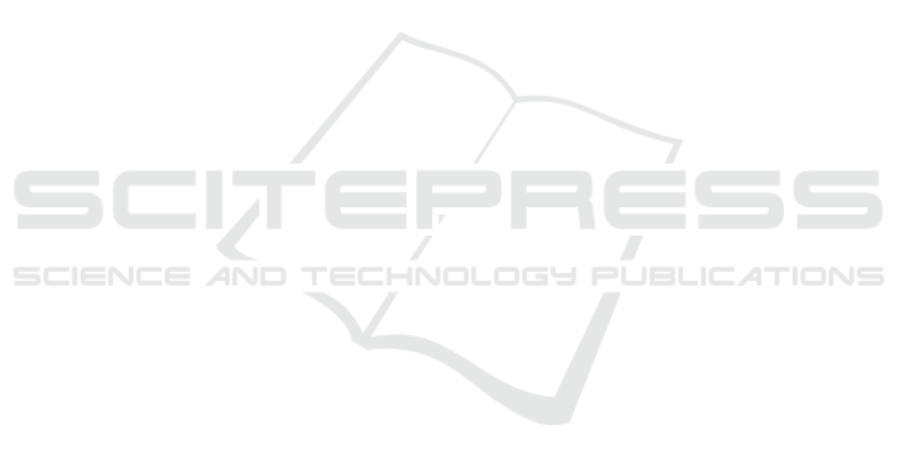
include evaluation on other datasets as well as stud-
ies on temporal consistency to apply our methods to
videos.
REFERENCES
Bertozzi, M. and Broggi, A. (1998). GOLD: A parallel real-
time stereo vision system for generic obstacle and lane
detection. IEEE Transactions on Image Processing,
7(1):62–81.
Billy, A., Pouteau, S., Desbarats, P., Chaumette, S., and
Domenger, J. P. (2019). Adaptive slam with synthetic
stereo dataset generation for real-time dense 3d re-
construction. In VISIGRAPP 2019 - Proceedings of
the 14th International Joint Conference on Computer
Vision, Imaging and Computer Graphics Theory and
Applications, volume 5, pages 840–848.
Cunha, J., Pedrosa, E., Cruz, C., Neves, A., and Lau, N.
(2011). Using a depth camera for indoor robot local-
ization and navigation. In DETI/IEETA-University of
Aveiro.
Delage, E., Lee, H., and Ng, A. Y. (2007). Automatic
single-image 3d reconstructions of indoor Manhattan
world scenes. Springer Tracts in Advanced Robotics,
28.
D
´
ıaz, R. Soft Labels for Ordinal Regression. Technical
report.
Eigen, D., Puhrsch, C., and Fergus, R. (2014). Depth map
prediction from a single image using a multi-scale
deep network. In Advances in Neural Information
Processing Systems, volume 3, pages 2366–2374.
Fu, H., Gong, M., Wang, C., Batmanghelich, K., and Tao,
D. Deep Ordinal Regression Network for Monocular
Depth Estimation. Technical report.
Garg, R., Vijay Kumar, B. G., Carneiro, G., and Reid, I.
(2016). Unsupervised CNN for single view depth es-
timation: Geometry to the rescue. In Lecture Notes in
Computer Science (including subseries Lecture Notes
in Artificial Intelligence and Lecture Notes in Bioin-
formatics), volume 9912 LNCS, pages 740–756.
Geiger, A., Lenz, P., and Urtasun, R. (2012). Are we ready
for autonomous driving? the KITTI vision benchmark
suite. In Proceedings of the IEEE Computer Society
Conference on Computer Vision and Pattern Recogni-
tion, pages 3354–3361.
Godard, C., Mac Aodha, O., and Brostow, G. J. (2017). Un-
supervised monocular depth estimation with left-right
consistency. In Proceedings - 30th IEEE Conference
on Computer Vision and Pattern Recognition, CVPR
2017, volume 2017-Janua, pages 6602–6611.
Henry, P., Krainin, M., Herbst, E., Ren, X., and Fox,
D. (2014). RGB-D mapping: Using depth cameras
for dense 3D modeling of indoor environments. In
Springer Tracts in Advanced Robotics, volume 79,
pages 477–491. Springer Verlag.
Hirschm
¨
uller, H. (2008). Stereo processing by semiglobal
matching and mutual information. IEEE Transac-
tions on Pattern Analysis and Machine Intelligence,
30(2):328–341.
Koch, T., Liebel, L., Fraundorfer, F., and K
¨
orner, M. Eval-
uation of CNN-based Single-Image Depth Estimation
Methods. Technical report.
Mayer, N., Ilg, E., Hausser, P., Fischer, P., Cremers, D.,
Dosovitskiy, A., and Brox, T. (2016). A Large Dataset
to Train Convolutional Networks for Disparity, Opti-
cal Flow, and Scene Flow Estimation. In Proceedings
of the IEEE Computer Society Conference on Com-
puter Vision and Pattern Recognition, volume 2016-
Decem, pages 4040–4048.
Mutimbu, L. and Robles-Kelly, A. (2013). A relaxed fac-
torial Markov random field for colour and depth es-
timation from a single foggy image. In 2013 IEEE
International Conference on Image Processing, ICIP
2013 - Proceedings, pages 355–359.
Ong, S. and Nee, A. (2013). Virtual and augmented reality
applications in manufacturing.
Pillai, S., Ambrus¸, R., and Gaidon, A. (2019). SuperDepth:
Self-supervised, super-resolved monocular depth esti-
mation. In Proceedings - IEEE International Confer-
ence on Robotics and Automation, volume 2019-May,
pages 9250–9256.
Ren, H., El-khamy, M., and Lee, J. (2019). Deep Robust
Single Image Depth Estimation Neural Network Us-
ing Scene Understanding.
Ronneberger, O., Fischer, P., and Brox, T. (2015). U-
net: Convolutional networks for biomedical image
segmentation. In Lecture Notes in Computer Science
(including subseries Lecture Notes in Artificial Intel-
ligence and Lecture Notes in Bioinformatics), volume
9351, pages 234–241. Springer Verlag.
Saxena, A., Sun, M., and Ng, A. Y. (2007). Learning 3-D
scene structure from a single still image. In Proceed-
ings of the IEEE International Conference on Com-
puter Vision.
Scharstein, D., Szeliski, R., and Zabih, R. (2001). A
taxonomy and evaluation of dense two-frame stereo
correspondence algorithms. In Proceedings - IEEE
Workshop on Stereo and Multi-Baseline Vision, SMBV
2001, pages 131–140.
Schwarz, B. (2010). industry perspective technology focus
lIDAR Mapping the world in 3D. Technical report.
Silberman, N., Hoiem, D., Kohli, P., and Fergus, R.
(2012). Indoor segmentation and support inference
from RGBD images. In Lecture Notes in Computer
Science (including subseries Lecture Notes in Artifi-
cial Intelligence and Lecture Notes in Bioinformatics),
volume 7576 LNCS, pages 746–760.
Thrun, S. (2008). Simultaneous localization and mapping.
Ullman, S. (1979). The interpretation of structure from mo-
tion. Proceedings of the Royal Society of London. Se-
ries B, Containing papers of a Biological character.
Royal Society (Great Britain), 203(1153):405–426.
Urmson, C., Anhalt, J., Bagnell, D., Baker, C., Bittner,
R., Clark, M. N., Dolan, J., Duggins, D., Galatali,
T., Geyer, C., Gittleman, M., Harbaugh, S., Hebert,
M., Howard, T. M., Kolski, S., Kelly, A., Likhachev,
M., McNaughton, M., Miller, N., Peterson, K., Pil-
nick, B., Rajkumar, R., Rybski, P., Salesky, B., Seo,
Y.-W., Singh, S., Snider, J., Stentz, A., Whittaker,
VISAPP 2020 - 15th International Conference on Computer Vision Theory and Applications
534