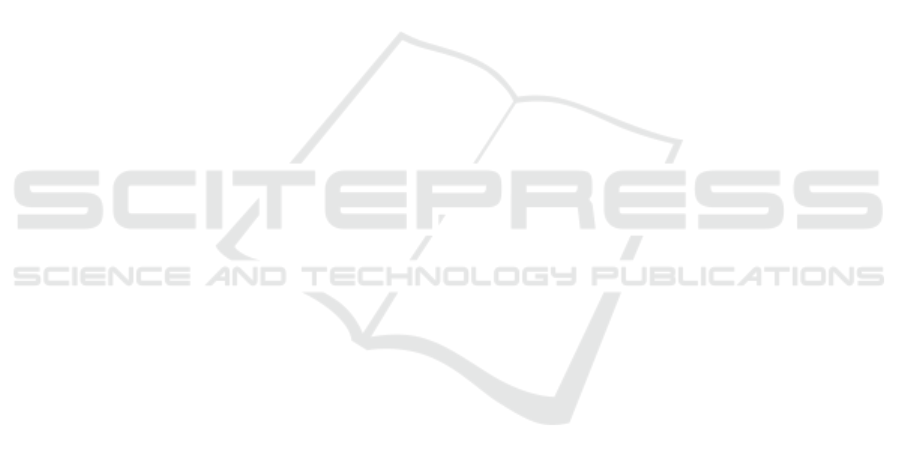
IEEE Conference on Computer Vision and Pattern
Recognition, CVPR, USA, July 21-26, pages 2492–
2500.
Buonamici, F. and Carfagni, M. (2016). Reverse engineer-
ing of mechanical parts: A brief overview of existing
approaches and possible new strategies. International
Design Engineering Technical Conferences and Com-
puters and Information in Engineering Conference.
Chum, O. and Matas, J. (2005). Matching with PROSAC -
progressive sample consensus. In 2005 IEEE Com-
puter Society Conference on Computer Vision and
Pattern Recognition CVPR, 20-26 June, USA, pages
220–226.
Dahnert, M., Dai, A., Guibas, L., and Nießner, M. (2019).
Joint embedding of 3d scan and cad objects.
Du, T., Inala, J. P., Pu, Y., Spielberg, A., Schulz, A., Rus,
D., Solar-Lezama, A., and Matusik, W. (2018). In-
versecsg: automatic conversion of 3d models to CSG
trees. ACM Trans. Graph., 37(6):213:1–213:16.
Fischler, M. A. and Bolles, R. C. (1981). Random sample
consensus: A paradigm for model fitting with appli-
cations to image analysis and automated cartography.
Commun. ACM, 24(6):381–395.
Gauthier, S., Puech, W., B
´
eni
`
ere, R., and Subsol, G. (2019).
Cad-driven pattern recognition in reverse engineered
models. In Proceedings of the 14th International Joint
Conference on Computer Vision, Imaging and Com-
puter Graphics Theory and Applications, VISIGRAPP
2019, Volume 1: GRAPP, Prague, Czech Republic,
February 25-27, 2019., pages 244–254.
Jia, P. (2017). Fitting a parametric model to a cloud of
points via optimization methods.
Kaiser, A., Zepeda, J. A. Y., and Boubekeur, T. (2019). A
survey of simple geometric primitives detection meth-
ods for captured 3d data. Computer Graphics Forum,
38(1):167–196.
Kang, Z. and Li, Z. (2015). Primitive fitting based on the
efficient multibaysac algorithm. PLOS ONE, 10(3):1–
21.
Kov
´
acs, I., V
´
arady, T., and Salvi, P. (2015). Applying ge-
ometric constraints for perfecting CAD models in re-
verse engineering. Graphical Models, 82:44–57.
Li, L., Sung, M., Dubrovina, A., Yi, L., and Guibas, L. J.
(2019). Supervised fitting of geometric primitives to
3d point clouds. In IEEE Conference on Computer
Vision and Pattern Recognition, CVPR, USA, June 16-
20, pages 2652–2660.
Li, Y., Wu, X., Chrysanthou, Y., Sharf, A., Cohen-Or, D.,
and Mitra, N. J. (2011). Globfit: consistently fit-
ting primitives by discovering global relations. ACM
Trans. Graph., 30(4):52.
Mo, K., Zhu, S., Chang, A. X., Yi, L., Tripathi, S., Guibas,
L. J., and Su, H. (2019). PartNet: A large-scale bench-
mark for fine-grained and hierarchical part-level 3D
object understanding. In The IEEE Conference on
Computer Vision and Pattern Recognition (CVPR).
Musy, M., Dalmasso, G., Sharpe, J., and Sime, N. (2019).
vtkplotter: plotting in fenics with python. In FEn-
iCS’2019 Conference.
Patil, A. K., Holi, P., Lee, S. K., and Chai, Y. H. (2017). An
adaptive approach for the reconstruction and modeling
of as-built 3d pipelines from point clouds. Automation
in Construction, 75:65 – 78.
Ranftl, R. and Koltun, V. (2018). Deep fundamental matrix
estimation. In Computer Vision - ECCV 2018 - 15th
European Conference, Munich, Germany, September
8-14, 2018, Proceedings, Part I, pages 292–309.
Ribes, A. and Caremoli, C. (2007). Salom
´
e platform com-
ponent model for numerical simulation. COMPSAC
07: Proceeding of the 31st Annual International Com-
puter Software and Applications Conference, pages
553–564.
Schnabel, R., Wahl, R., and Klein, R. (2007). Efficient
ransac for point-cloud shape detection. Computer
Graphics Forum, 26(2):214–226.
Sharma, G., Goyal, R., Liu, D., Kalogerakis, E., and Maji,
S. (2018). Csgnet: Neural shape parser for con-
structive solid geometry. In 2018 IEEE Conference
on Computer Vision and Pattern Recognition, CVPR,
USA, June 18-22, pages 5515–5523.
TraceParts S.A.S. (2019). TraceParts.
Trimesh (2019). [Computer software]. Retrieved from
https://github.com/mikedh/trimesh.
Tulsiani, S., Su, H., Guibas, L. J., Efros, A. A., and Ma-
lik, J. (2017). Learning shape abstractions by assem-
bling volumetric primitives. In 2017 IEEE Conference
on Computer Vision and Pattern Recognition, CVPR,
USA, July 21-26, pages 1466–1474.
V
´
arady, T., Martin, R. R., and Cox, J. (1997). Reverse
engineering of geometric models - an introduction.
Computer-Aided Design, 29(4):255–268.
Werghi, N., Fisher, R. B., Robertson, C., and Ashbrook, A.
(1999). Object reconstruction by incorporating geo-
metric constraints in reverse engineering. Computer-
Aided Design, 31(6):363–399.
Wu, Q., Xu, K., and Wang, J. (2018). Constructing 3d CSG
models from 3d raw point clouds. Comput. Graph.
Forum, 37(5):221–232.
Yi, C., Zhang, Y., Wu, Q., Xu, Y., Remil, O., Wei, M., and
Wang, J. (2017). Urban building reconstruction from
raw lidar point data. Computer-Aided Design, 93:1–
14.
Zou, C., Yumer, E., Yang, J., Ceylan, D., and Hoiem, D.
(2017). 3d-prnn: Generating shape primitives with re-
current neural networks. In IEEE International Con-
ference on Computer Vision, ICCV 2017, Venice, Italy,
October 22-29, 2017, pages 900–909.
GRAPP 2020 - 15th International Conference on Computer Graphics Theory and Applications
398