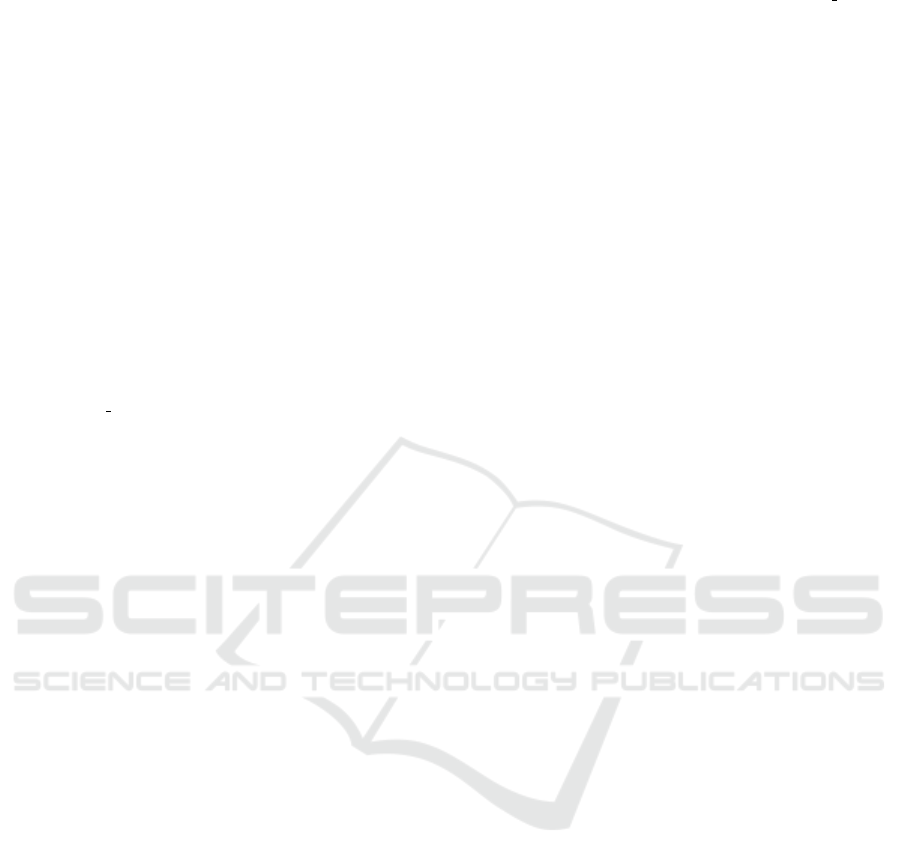
ics of complex networks, Rev. Mod. Phys, 74(1):47–
97.
Bidkhori, G., Narimani, Z., Ashtiani, S. H., Moeini, A.,
Nowzari-Dalini, A., and Masoudi-Nejad, A. (2013).
Reconstruction of an integrated genome-scale co-
expression network reveals key modules involved in
lung adenocarcinoma. PloS one, 8(7):e67552.
Bullinger, L., D
¨
ohner, K., Bair, E., Fr
¨
ohling, S., Schlenk,
R. F., Tibshirani, R., D
¨
ohner, H., and Pollack, J. R.
(2004). Use of gene-expression profiling to iden-
tify prognostic subclasses in adult acute myeloid
leukemia. New England Journal of Medicine,
350(16):1605–1616.
Canchi, S., Raao, B., Masliah, D., Rosenthal, S. B., Sasik,
R., Fisch, K. M., De Jager, P. L., Bennett, D. A., and
Rissman, R. A. (2019). Integrating gene and protein
expression reveals perturbed functional networks in
alzheimer’s disease. Cell reports, 28(4):1103–1116.
Consortium, G. O. (2004). The gene ontology (go) database
and informatics resource. Nucleic acids research,
32(suppl 1):D258–D261.
Edgar, R., Domrachev, M., and Lash, A. E. (2002). Gene
expression omnibus: Ncbi gene expression and hy-
bridization array data repository. Nucleic acids re-
search, 30(1):207–210.
Eisen, M. B., Spellman, P. T., Brown, P. O., and Botstein,
D. (1998). Cluster analysis and display of genome-
wide expression patterns. Proceedings of the National
Academy of Sciences, 95(25):14863–14868.
Franz, M., Rodriguez, H., Lopes, C., Zuberi, K., Montojo,
J., Bader, G. D., and Morris, Q. (2018). Genemania
update 2018. Nucleic acids research, 46(W1):W60–
W64.
Iba, H. and Noman, N. (2016). Evolutionary computation
in gene regulatory network research. John Wiley &
Sons.
Langfelder, P. and Horvath, S. (2007). Eigengene networks
for studying the relationships between co-expression
modules. BMC systems biology, 1(1):54.
Langfelder, P. and Horvath, S. (2008). Wgcna: an r pack-
age for weighted correlation network analysis. BMC
bioinformatics, 9(1):559.
Maere, S., Heymans, K., and Kuiper, M. (2005). Bingo: a
cytoscape plugin to assess overrepresentation of gene
ontology categories in biological networks. Bioinfor-
matics, 21(16):3448–3449.
Montazeri, H., Coto-Llerena, M., Bianco, G., Zangeneh, E.,
Taha-Mehlitz, S., Paradiso, V., Srivatsa, S., de Weck,
A., Roma, G., Lanzafame, M., et al. (2019). Apsic:
Analysis of perturbation screens for the identification
of novel cancer genes. bioRxiv, page 807248.
Panov (2014). Basics of Molecular Biology and Genetics.
University ”St. Cyril and Methodius”, Skopje.
Rueda, L. (2018). Microarray image and data analysis:
theory and practice. CRC Press.
Sanguinetti, G. and Huynh-Thu, V. (2018). Gene Regula-
tory Networks: Methods and Protocols. Methods in
Molecular Biology. Springer New York.
Segal, E., Wang, H., and Koller, D. (2003). Discovering
molecular pathways from protein interaction and gene
expression data. Bioinformatics, 19(suppl 1):i264–
i272.
Simjanoska, M., Bogdanova, A. M., and Panov, S. (2013).
Gene ontology analysis of colorectal cancer biomark-
ers probed with affymetrix and illumina microarrays.
In IJCCI, pages 396–406.
Slizhikova, D., Vinogradova, T., and Sverdlov, E. (2005).
The nola2 and rps3a genes as highly informative
markers of human squamous cell carcinoma of lung.
Russian Journal of Bioorganic Chemistry, 31(2):178–
182.
Sotiriou, C., Wirapati, P., Loi, S., Harris, A., Fox, S.,
Smeds, J., Nordgren, H., Farmer, P., Praz, V., Haibe-
Kains, B., et al. (2006). Gene expression profiling in
breast cancer: understanding the molecular basis of
histologic grade to improve prognosis. Journal of the
National Cancer Institute, 98(4):262–272.
Sun, W., Zhang, K., Zhang, X., Lei, W., Xiao, T., Ma, J.,
Guo, S., Shao, S., Zhang, H., Liu, Y., et al. (2004).
Identification of differentially expressed genes in hu-
man lung squamous cell carcinoma using suppression
subtractive hybridization. Cancer letters, 212(1):83–
93.
The Gene Ontology Consortium (2018). The Gene Ontol-
ogy Resource: 20 years and still GOing strong. Nu-
cleic Acids Research, 47(D1):D330–D338.
van Dam, S., Vosa, U., van der Graaf, A., Franke, L., and
de Magalhaes, J. P. (2017). Gene co-expression analy-
sis for functional classification and gene–disease pre-
dictions. Briefings in bioinformatics, 19(4):575–592.
Weinberg, R. A. (2013). The Biology of Cancer: Second
International Student Edition. WW Norton & Com-
pany.
Yang, Y., Han, L., Yuan, Y., Li, J., Hei, N., and Liang, H.
(2014). Gene co-expression network analysis reveals
common system-level properties of prognostic genes
across cancer types. Nature communications, 5:3231.
Yim, W. C., Min, K., Jung, D., Lee, B.-M., and Kwon,
Y. (2011). Cross experimental analysis of microar-
ray gene expression data from volatile organic com-
pounds treated targets. Molecular & Cellular Toxicol-
ogy, 7(3):233.
Yin, L., Cai, Z., Zhu, B., and Xu, C. (2018). Identification
of key pathways and genes in the dynamic progression
of hcc based on wgcna. Genes, 9(2):92.
Yip, A. M. and Horvath, S. (2007). Gene network inter-
connectedness and the generalized topological overlap
measure. BMC bioinformatics, 8(1):22.
Zhang, B. and Horvath, S. (2005). A general framework for
weighted gene co-expression network analysis. Statis-
tical applications in genetics and molecular biology,
4(1).
Zhang, J., Baran, J., Cros, A., Guberman, J. M., Haider,
S., Hsu, J., Liang, Y., Rivkin, E., Wang, J., Whitty,
B., et al. (2011). International cancer genome con-
sortium data portal—a one-stop shop for cancer ge-
nomics data. Database, 2011.
Zhu, Z., Jin, Z., Deng, Y., Wei, L., Yuan, X., Zhang, M.,
and Sun, D. (2019). Co-expression network analysis
identifies four hub genes associated with prognosis in
soft tissue sarcoma. Frontiers in genetics, 10:37.
Gene Co-expression Analysis for Lung Cancer Biomarkers Detection
67