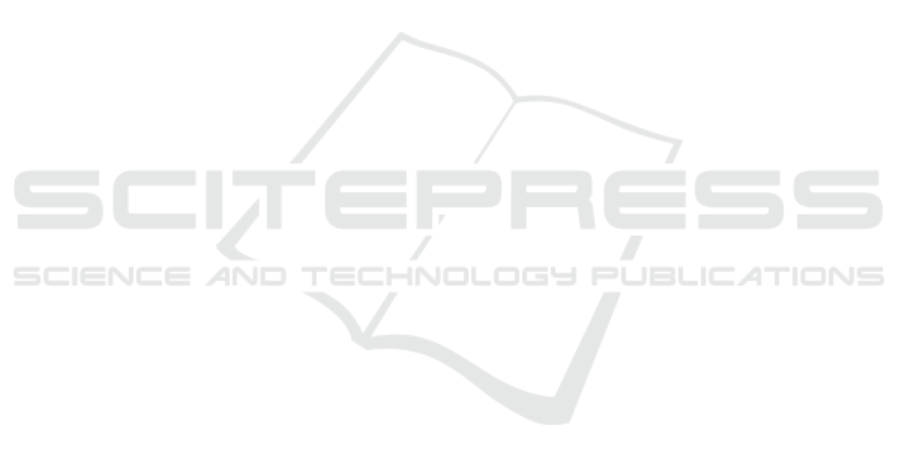
rived from them. The best results have been obtained
using Mash based on the recomputed distances after
excluding the self-similarities (Figure 3(h)). Also, the
operation of balancing the samples by reducing the
size of the databases allows for obtaining similar re-
sults with the CoMeta program (Figure 3(d)). It is
worth noting here that such an operation is indirectly
performed by Mash, as it builds sketches of a constant
size, independently on the sample size.
4 CONCLUSIONS AND FUTURE
WORK
In this paper, we proposed a new approach toward
clustering metagenomic reads in search of the sam-
ples that have common origin. The results of our ex-
perimental study indicate that the presented method
allows for separating the samples based on their mu-
tual similarity.
An important advantage of the reported approach
lies in determining the sample similarity at the reads
level without the necessity to understand the contents
of these samples. Therefore, our methodology does
not require large databases (taxonomical and func-
tional) of annotated reads. Here, we used two pro-
grams (CoMeta and Mash) for comparing the sam-
ples prior to clustering, and the results obtained for
the best variants of both programs were similar. Im-
portantly, we show that clustering of the metagenomic
samples can be automated, which may be extremely
important when a larger number of samples is to be
processed.
In the presented preliminary research, we used the
samples from two large cities located relatively close
to each other—Boston and New York. While based on
that limited dataset it is difficult to indicate which pro-
gram is more suitable for clustering, we have demon-
strated how important it is to deal with the problem
of imbalanced data as well as to preprocess the sim-
ilarity scores. In our future work, we will extend the
database used for evaluation to verify this approach
for a larger number of clusters (i.e., ground-truth lo-
cations) and increase their diversity.
ACKNOWLEDGEMENTS
This work was supported by the Polish Na-
tional Science Centre under the project DEC-
2015/19/D/ST6/03252. This research was supported
in part by PL-Grid Infrastructure.
REFERENCES
Afshinnekoo, E., Meydan, C., Chowdhury, S., Jaroudi, D.,
Boyer, C., Bernstein, N., Maritz, J. M., Reeves, D.,
Gandara, J., Chhangawala, S., et al. (2015). Geospa-
tial resolution of human and bacterial diversity with
city-scale metagenomics. Cell systems, 1(1):72–87.
Bengtsson-Palme, J. (2018). Strategies for taxonomic and
functional annotation of metagenomes. In Metage-
nomics, pages 55–79. Elsevier.
Breitwieser, F. P., Lu, J., and Salzberg, S. L. (2017). A re-
view of methods and databases for metagenomic clas-
sification and assembly. Briefings in bioinformatics.
Casimiro-Soriguer, C. S., Loucera, C., Perez Florido, J.,
L
´
opez-L
´
opez, D., and Dopazo, J. (2019). Antibi-
otic resistance and metabolic profiles as functional
biomarkers that accurately predict the geographic ori-
gin of city metagenomics samples. Biology Direct,
14(1):15.
Deorowicz, S., Kokot, M., Grabowski, S., and Debudaj-
Grabysz, A. (2015). KMC 2: fast and resource-frugal
k-mer counting. Bioinformatics, 31(10):1569–1576.
Handelsman, J. (2004). Metagenomics: application of ge-
nomics to uncultured microorganisms. Microbiol Mol
Biol Rev., 68(4).
Harris, Z. N., Dhungel, E., Mosior, M., and Ahn, T.-H.
(2019). Massive metagenomic data analysis using
abundance-based machine learning. Biology Direct,
14(1):12.
Hsu, T., Joice, R., Vallarino, J., Abu-Ali, G., Hartmann,
E. M., Shafquat, A., DuLong, C., Baranowski, C.,
Gevers, D., Green, J. L., et al. (2016). Urban tran-
sit system microbial communities differ by surface
type and interaction with humans and the environ-
ment. Msystems, 1(3):e00018–16.
Kawulok, J. and Deorowicz, S. (2015). CoMeta: Clas-
sication of metagenomes using k-mers. PLoS ONE,
10(4):e0121453.
Kawulok, J. and Kawulok, M. (2018). Environmen-
tal metagenome classification for soil-based forensic
analysis. In BIOINFORMATICS, pages 182–187.
Kawulok, J., Kawulok, M., and Deorowicz, S. (2019). Envi-
ronmental metagenome classification for constructing
a microbiome fingerprint. Biology Direct, 14(1).
Li, W., Fu, L., Niu, B., Wu, S., and Wooley, J. (2012). Ultra-
fast clustering algorithms for metagenomic sequence
analysis. Briefings in bioinformatics, 13(6):656–668.
Ondov, B. D., Starrett, G. J., Sappington, A., Kostic,
A., Koren, S., Buck, C. B., and Phillippy, A. M.
(2019). Mash screen: High-throughput sequence con-
tainment estimation for genome discovery. BioRxiv,
page 557314.
Oulas, A., Pavloudi, C., Polymenakou, P., Pavlopoulos,
G. A., Papanikolaou, N., Kotoulas, G., Arvanitidis,
C., and Iliopoulos, l. (2015). Metagenomics: tools
and insights for analyzing next-generation sequencing
data derived from biodiversity studies. Bioinformatics
and biology insights, 9:BBI–S12462.
Qiao, Y., Jia, B., Hu, Z., Sun, C., Xiang, Y., and Wei, C.
(2018). MetaBinG2: a fast and accurate metagenomic
BIOINFORMATICS 2020 - 11th International Conference on Bioinformatics Models, Methods and Algorithms
224