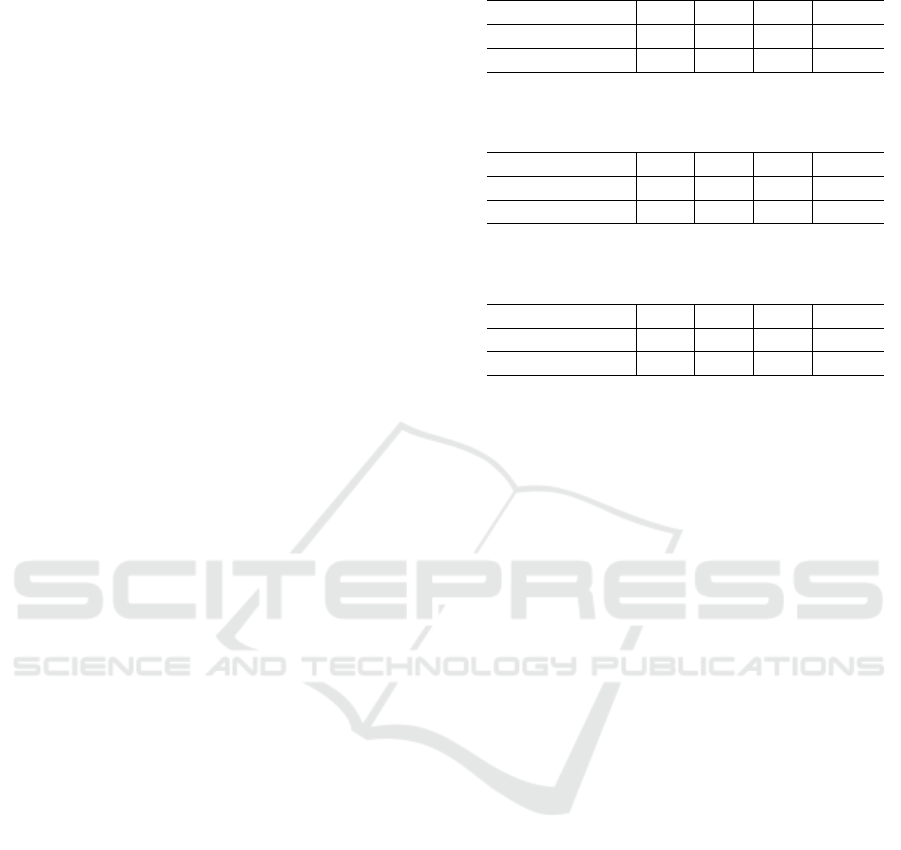
significantly with time and depend on the neonate’s
state but we assume that the anomalies are estimated
from the empirical pdf distributions given in 7.
In order to make the final decision, we need to uti-
lize the information available to us: the local binary
decisions u
n
. Note that in the presence of the training
set (annotations) the initial guesses for unknown pa-
rameters can be obtained from the training set but they
are non-stationary and change with time. The details
of the implementation are given in our previous work,
(Liu et al., 2014), (Jeremic and Nikolic, 2019).
3 RESULTS
We evaluate the performance of the proposed algo-
rithms on the data set consisting of preterm infants
(GA less than 32 weeks) admitted to the Neonatal In-
tensive Care Unit at McMaster Hospital. Due to phys-
ical limitations we were able to obtain prior expect
knowledge on a very limited time length and limited
set of patients. We selected only patients with seizure
epochs and obtained expert annotations on a limited
length (2 hours per patient).
For illustrational purposes in Figures 4-6 , we plot
the detection performance as a scatter diagram of win-
dows selected from testing data. Note that in the pres-
ence of motion artifacts the actual performance will
actually vary significantly. Furthermore because the
original system design was based on no-seizures the
system was calibrated so that the probability of false
alarm is controlled. Due to motion artifacts and re-
action to pain stimuli during medical procedures in
NICU it is quite likely that local detectors will iden-
tify these manifestation in EEG as false seizure. In
Table 1 we present the results of our previously pro-
posed blind system (Jeremic and Nikolic, 2019) with-
out any training in which the detector anomalies and
priors are estimated and the local detectors are based
on (Rankine et al., 2007), (Gotman, 1997) and (Celka
and Colditz, 2002). In Tables 2 and 3 we illustrate our
two proposed algorithms with average probability of
error averaged of 1000 randomized training set runs.
As expected the proposed system performs better due
to the fact that expert annotations are available.
For comparison purposes we also implemented a
support vector machine (SVM) classifier which at-
tempts to find an optimal hyperplane in the feature
(or reduced dimension) space which minimizes over-
all probability of classification error. To this purpose
we use PSD images and reduce their dimensional-
ity using principal component analysis (PCA) as fea-
ture reduction preprocessing technique. The overall
average accuracy of SVM was 82% for seizure-free
Table 1: Average seizure detection performance - blind.
d
R1
d
R2
d
R3
Fused
false seizures 0.14 0.15 0.16 0.11
missed seizures 0.17 0.14 0.16 0.15
Table 2: Average seizure detection performance - training
set maximum a posteriori.
d
R1
d
R2
d
R3
Fused
false seizures 0.07 0.09 0.12 0.05
missed seizures 0.09 0.08 0.11 0.07
Table 3: Average seizure detection performance - training
set based maximum likelihood.
d
R1
d
R2
d
R3
Fused
false seizures 0.09 0.11 0.15 0.09
missed seizures 0.11 0.10 0.14 0.12
epochs and 78% for seizure epochs. The number of
features selected was set to 20 in order to capture 85%
of the variance (arbitrarily set). Note that the number
of features can be selected optimally and it will be
addressed in future work.
4 CONCLUSIONS
Automatic systems for seizure detection have been
subject of considerable research interest in the past.
One of main advantages lies in the fact that expert
time is potentially required only during the training
session. Furthermore, for newborn patients admitted
to NICU such systems enable continuous monitoring
of seizure events and hence can provide better insight
into neurological development. In recent years signif-
icant effort has been placed on developing systems
that predict seizures in order to potentially counter
them with appropriately generated electrical stimuli.
To this purpose in this paper we examined possibility
of detecting seizures by measuring different distances
using STFT. To achieve this goal we define local de-
tectors using empirically determined parameters and
fuse their local decisions using our previously devel-
oped information fusion algorithm for seizure detec-
tion. We demonstrated the applicability of the pro-
posed algorithms using a real data set consisting of
multiple NICU patients and expert annotations.
Our results indicate that training techniques of-
fer better performance if adequate expert annotations
are available. An effort should be placed on ex-
amining possibility of using machine learning tech-
niques which would enable efficient management of
resources. Due to patient-to-patient variability we
BIOSIGNALS 2020 - 13th International Conference on Bio-inspired Systems and Signal Processing
346