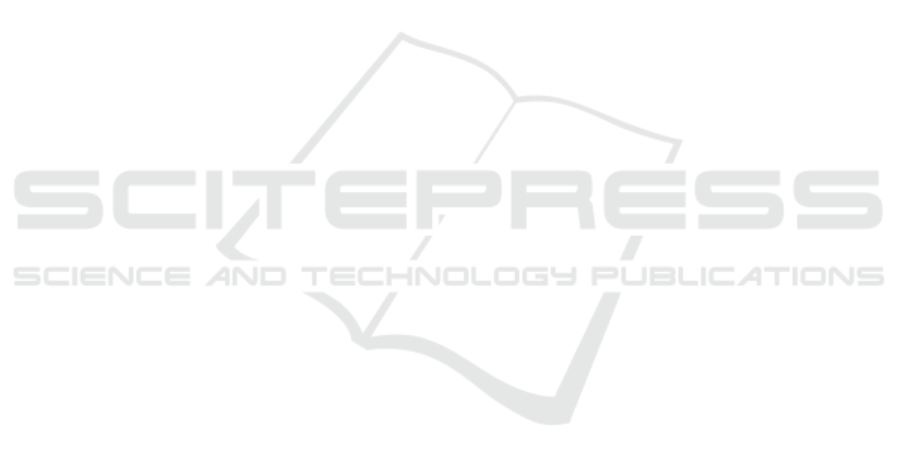
sign guidelines, techniques and applications. In Euro-
graphics (STARs), pages 39–63.
Del Coso, C., Fustes, D., Dafonte, C., N
´
ovoa, F. J.,
Rodr
´
ıguez-Pedreira, J. M., and Arcay, B. (2015). Mix-
ing numerical and categorical data in a self-organizing
map by means of frequency neurons. Applied Soft
Computing, 36:246–254.
Dunne, C. and Shneiderman, B. (2013). Motif simplifica-
tion: improving network visualization readability with
fan, connector, and clique glyphs. In Proceedings of
the SIGCHI Conference on Human Factors in Com-
puting Systems, pages 3247–3256. ACM.
Fuchs, J., Isenberg, P., Bezerianos, A., Fischer, F., and
Bertini, E. (2014). The influence of contour on simi-
larity perception of star glyphs. IEEE transactions on
visualization and computer graphics, 20(12).
Fuchs, J., Isenberg, P., Bezerianos, A., and Keim, D. (2017).
A systematic review of experimental studies on data
glyphs. IEEE Trans. on Visualization and Computer
Graphics, 23(7).
Furletti, B., Gabrielli, L., Renso, C., and Rinzivillo, S.
(2012). Identifying users profiles from mobile calls
habits. In Proceedings of the ACM SIGKDD int. work-
shop on urban computing, pages 17–24. ACM.
Gorricha, J. M. and Lobo, V. J. (2011). On the use of
three-dimensional self-organizing maps for visualiz-
ing clusters in georeferenced data. In Information Fu-
sion and Geographic Information Systems, pages 61–
75. Springer.
Hsu, C.-C. (2006). Generalizing self-organizing map for
categorical data. IEEE transactions on Neural Net-
works, 17(2):294–304.
Hsu, C.-C. and Kung, C.-H. (2013). Incorporating unsu-
pervised learning with self-organizing map for visual-
izing mixed data. In 2013 Ninth International Con-
ference on Natural Computation (ICNC), pages 146–
151. IEEE.
Hsu, C.-C. and Lin, S.-H. (2011). Visualized analysis of
mixed numeric and categorical data via extended self-
organizing map. IEEE transactions on neural net-
works and learning systems, 23(1):72–86.
Jacomy, M., Venturini, T., Heymann, S., and Bastian, M.
(2014). Forceatlas2, a continuous graph layout algo-
rithm for handy network visualization designed for the
gephi software. PloS one, 9(6).
Kameoka, Y., Yagi, K., Munakata, S., and Yamamoto, Y.
(2015). Customer segmentation and visualization by
combination of self-organizing map and cluster anal-
ysis. In 2015 13th International Conference on ICT
and Knowledge Engineering (ICT & Knowledge En-
gineering 2015), pages 19–23. IEEE.
Kohonen, T. (1990). The self-organizing map. Proceedings
of the IEEE, 78(9):1464–1480.
Koua, E. (2003). Using self-organizing maps for informa-
tion visualization and knowledge discovery in com-
plex geospatial datasets. Proceedings of 21st int. car-
tographic renaissance (ICC), pages 1694–1702.
Mackinlay, J. (1986). Automating the design of graphical
presentations of relational information. Acm Transac-
tions On Graphics (Tog), 5(2):110–141.
Milosevic, M., McConville, K. M. V., Sejdic, E., Masani,
K., Kyan, M. J., and Popovic, M. R. (2012). Visualiza-
tion of trunk muscle synergies during sitting perturba-
tions using self-organizing maps (som). IEEE Trans-
actions on Biomedical Engineering, 59(9):2516–
2523.
Morais, A. M. M., Quiles, M. G., and Santos, R. D. (2014).
Icon and geometric data visualization with a self-
organizing map grid. In International Conference on
Computational Science and Its Applications, pages
562–575. Springer.
Olszewski, D. (2014). Fraud detection using self-organizing
map visualizing the user profiles. Knowledge-Based
Systems, 70:324–334.
Peng, W., Ward, M. O., and Rundensteiner, E. A. (2004).
Clutter reduction in multi-dimensional data visualiza-
tion using dimension reordering. In Information Visu-
alization, 2004. INFOVIS 2004. IEEE Symposium on,
pages 89–96. IEEE.
Rajagopal, D. et al. (2011). Customer data cluster-
ing using data mining technique. arXiv preprint
arXiv:1112.2663.
Rogovschi, N., Lebbah, M., and Bennani, Y. (2011). A self-
organizing map for mixed continuous and categorical
data. Int. Journal of Computing, 10(1):24–32.
Schreck, T., Bernard, J., Von Landesberger, T., and
Kohlhammer, J. (2009). Visual cluster analysis of tra-
jectory data with interactive kohonen maps. Informa-
tion Visualization, 8(1):14–29.
Shen, Z., Ogawa, M., Teoh, S. T., and Ma, K.-L. (2006).
Biblioviz: a system for visualizing bibliography in-
formation. In Proceedings of the 2006 Asia-Pacific
Symposium on Information Visualisation-Volume 60,
pages 93–102. Australian Computer Society, Inc.
Siegel, J. H., Farrell, E. J., Goldwyn, R. M., and Friedman,
H. P. (1972). The surgical implications of physio-
logic patterns in myocardial infarction shock. Surgery,
72(1):126–141.
Tai, W.-S. and Hsu, C.-C. (2012). Growing self-organizing
map with cross insert for mixed-type data clustering.
Applied Soft Computing, 12(9):2856–2866.
Tufte, E. R. (2001). The visual display of quantitative in-
formation, volume 2. Graphics press Cheshire, CT.
Ward, M. O. (2002). A taxonomy of glyph placement strate-
gies for multidimensional data visualization. Informa-
tion Visualization, 1(3-4):194–210.
Wehrens, R., Buydens, L. M., et al. (2007). Self-and super-
organizing maps in r: the kohonen package. Journal
of Statistical Software, 21(5):1–19.
Wittenbrink, C. M., Pang, A. T., and Lodha, S. K. (1996).
Glyphs for visualizing uncertainty in vector fields.
IEEE Trans. on Visualization and Computer Graph-
ics, 2(3):266–279.
Yang, J., Peng, W., Ward, M. O., and Rundensteiner, E. A.
(2003). Interactive hierarchical dimension ordering,
spacing and filtering for exploration of high dimen-
sional datasets. In Information Visualization, 2003.
INFOVIS 2003. IEEE Symposium on, pages 105–112.
IEEE.
IVAPP 2020 - 11th International Conference on Information Visualization Theory and Applications
308