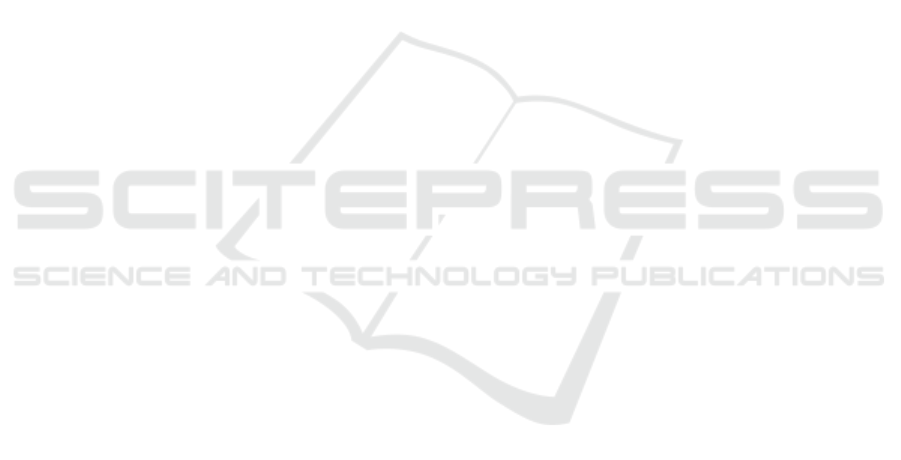
Armato III, S. G., McLennan, G., Bidaut, L., McNitt-Gray,
M. F., Meyer, C. R., Reeves, A. P., Clarke, L. P.,
et al. (2015). Data from lidc-idri. the cancer imaging
archive. DOI http://doi. org/10.7937 K, 9:7.
Armato III, S. G., McLennan, G., Bidaut, L., McNitt-Gray,
M. F., Meyer, C. R., Reeves, A. P., Zhao, B., Aberle,
D. R., Henschke, C. I., Hoffman, E. A., et al. (2011).
The lung image database consortium (lidc) and image
database resource initiative (idri): a completed refer-
ence database of lung nodules on ct scans. Medical
physics, 38(2):915–931.
Bi, L., Kim, J., Ahn, E., Kumar, A., Fulham, M., and Feng,
D. (2017). Dermoscopic image segmentation via mul-
tistage fully convolutional networks. IEEE Transac-
tions on Biomedical Engineering, 64(9):2065–2074.
Clark, K., Vendt, B., Smith, K., Freymann, J., Kirby, J.,
Koppel, P., Moore, S., Phillips, S., Maffitt, D., Pringle,
M., et al. (2013). The cancer imaging archive (tcia):
maintaining and operating a public information repos-
itory. Journal of digital imaging, 26(6):1045–1057.
Drozdzal, M., Chartrand, G., Vorontsov, E., Shakeri, M.,
Di Jorio, L., Tang, A., Romero, A., Bengio, Y., Pal,
C., and Kadoury, S. (2018). Learning normalized in-
puts for iterative estimation in medical image segmen-
tation. Medical image analysis, 44:1–13.
Gupta, D. and Anand, R. (2017). A hybrid edge-based seg-
mentation approach for ultrasound medical images.
Biomedical Signal Processing and Control, 31:116–
126.
J
´
egou, S., Drozdzal, M., Vazquez, D., Romero, A., and Ben-
gio, Y. (2017). The one hundred layers tiramisu: Fully
convolutional densenets for semantic segmentation. In
Proceedings of the IEEE Conference on Computer Vi-
sion and Pattern Recognition Workshops, pages 11–
19.
Lin, B. S., Michael, K., Kalra, S., and Tizhoosh, H. R.
(2017). Skin lesion segmentation: U-nets versus clus-
tering. In 2017 IEEE Symposium Series on Computa-
tional Intelligence (SSCI), pages 1–7. IEEE.
Nisbet, R., Miner, G., and Yale, K. (2018). Chapter 11
- model evaluation and enhancement. In Nisbet, R.,
Miner, G., and Yale, K., editors, Handbook of Statis-
tical Analysis and Data Mining Applications (Second
Edition), pages 215 – 233. Academic Press, Boston,
second edition edition.
Ronneberger, O., Fischer, P., and Brox, T. (2015). U-net:
Convolutional networks for biomedical image seg-
mentation. In International Conference on Medical
image computing and computer-assisted intervention,
pages 234–241. Springer.
Saraiva, A., Ferreira, N., Sousa, L., Carvalho da Costa, N.,
Sousa, J., Santos, D., and Soares, S. (2019a). Classi-
fication of images of childhood pneumonia using con-
volutional neural networks. In 6th International Con-
ference on Bioimaging, pages 112–119.
Saraiva, A., Melo, R., Filipe, V., Sousa, J., Ferreira, N. F.,
and Valente, A. (2018). Mobile multirobot manipula-
tion by image recognition.
Saraiva, A. A., Santos, D. B. S., Costa, N. J. C., Sousa, J.
V. M., Ferreira, N. M. F., Valente, A., and Soares, S.
F. S. P. (2019b). Models of learning to classify x-ray
images for the detection of pneumonia using neural
networks. In BIOIMAGING.
Shamir, R. R., Duchin, Y., Kim, J., Sapiro, G., and Harel,
N. (2019). Continuous dice coefficient: a method for
evaluating probabilistic segmentations. arXiv preprint
arXiv:1906.11031.
Siegel, R. L., Miller, K. D., and Jemal, A. (2019). Cancer
statistics, 2019. CA: a cancer journal for clinicians,
69(1):7–34.
Vardhana, M., Arunkumar, N., Lasrado, S., Abdulhay, E.,
and Ramirez-Gonzalez, G. (2018). Convolutional
neural network for bio-medical image segmentation
with hardware acceleration. Cognitive Systems Re-
search, 50:10–14.
Wang, G., Li, W., Zuluaga, M. A., Pratt, R., Patel, P. A.,
Aertsen, M., Doel, T., David, A. L., Deprest, J.,
Ourselin, S., et al. (2018). Interactive medical image
segmentation using deep learning with image-specific
fine tuning. IEEE transactions on medical imaging,
37(7):1562–1573.
Xue, Y., Xu, T., Zhang, H., Long, L. R., and Huang, X.
(2018). Segan: Adversarial network with multi-scale
l 1 loss for medical image segmentation. Neuroinfor-
matics, 16(3-4):383–392.
Use of Convolutional Neural Networks for Detection and Segmentation of Pulmonary Nodules in Computed Tomography Images
297