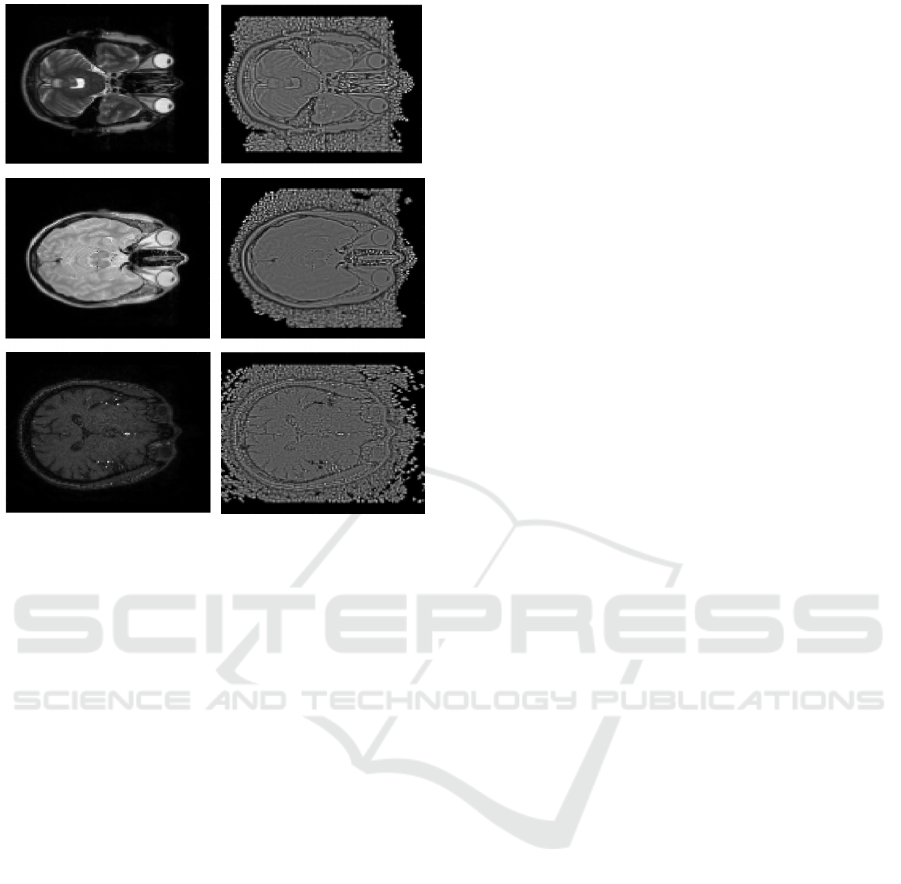
Figure 7: Top Row: T 2 image(left) and the respective quo-
tient image(right), Middle Row: PD image(left) and the
respective quotient image(right), Bottom Row: MRA im-
age(left) and the respective quotient image(right).
5 CONCLUSIONS
In this work a recently introduced Least-Squares
based groupwise image registration method was im-
proved in terms of its computational cost. This was
achieved by optimally defining a sequence of “cen-
troid” images whose limit was the desired but un-
known “mean” image for solving the groupwise prob-
lem. In addition, the proposed technique was prop-
erly adapted for its use in the groupwise registration
of multimodal images. The performance of the pro-
posed technique, from its application on a number
of experiments, was very good. The extensive eval-
uation of its performance against other state of the
art groupwise registration techniques and its exten-
sion for solving the corresponding groupwise volume
problem are currently under investigation.
ACKNOWLEDGEMENTS
This research is implemented through the Operational
Program ”Human Resources Development, Educa-
tion and Lifelong Learning” and is co-financed by the
European Union (European Social Fund) and Greek
national funds.
REFERENCES
Baker, S. and Matthews, I. (2004). Lucas-kanade 20 years
on: A unifying framework. International Journal of
Computer Vision 56(3), 221–255.
Cox, M. (2010). Unsupervised alignment of thousands of
images. PhD thesis, Queensland University of Tech-
nology, Brisbane, Queensland.
Cox, M., Sridharan, S., Lucey, S., and Cohn, J. (2008).
Least squares congealign for unsupervised alignment
of images. CVPR.
Cox, M., Sridharan, S., Lucey, S., and Cohn, J. (2009).
Least squares congealign for large number of images.
CVPR.
Guyader, Jean-Marie, Huizinga, Wyke, Poot, J., D. H., van
Kranenburg, Matthijs, Uitterdijk, A., Niessen, W. J.,
and Klein, S. (2018). Groupwise image registration
based on a total correlation dissimilarity measure for
quantitative mri and dynamic imaging data. Scientific
Reports, 8(1):13112.
Huang, G., Jain, V., and Learned-Miller, E. (2007). Unsu-
pervised joint alignment of complex images. In ICCV-
2007.
Huang, G. B., Mattar, M.and Lee, H., and Learned-Miller,
E. (2012). Learning to align from scratch. In Neural
Information Processing Systems.
Huizinga, W., Poot, D. H. J., Guyader, J.-M., Klaassen,
R., Coolen, B. F., van Kranenburg, M., van Geuns,
R. J. M., Uitterdijk, A., Polfliet, M., and et al., J. V.
(2016). Pca-based groupwise image registration for
quantitative mri. Med Image Anal., pages 65–78.
Learned-Miller, E. (2006). Data driven image models
through continuous joint alignment. IEEE T-PAMI,
28(2):236–250.
Liu, Q. and Wang, Q. (2014). Groupwise registra-
tion of brain magnetic resonance images: A review.
Journal of Shanghai Jiaotong University (Science),
19(6):755–762.
Nikolikos, N., Lamprinou, N., Boile, A., and Psarakis, E.
(2019). Multi-contrast mri volume alignment via ecc
maximization. In BioInformatics and BioEngineering
(BIBE). IEEE.
Nikolikos, N., Psarakis, E., and Lamprinou, N. (2017). A
new least squares based congealing technique. Else-
vier Pattern Recognition Letters, 95:58–64.
Storer, M. and Urschler, M. (2010). Intensity-based con-
gealing for unsupervised joint image alignment. In
ICPR, 2010.
Tong, C., Liu, X., Willer, F., and Tu, P. (2009). Automatic
facial landmark labelin with minimal supervision. In
CVPR, 2009.
Vedaldi, A. and Soatto, S. (2006). A complexity-distortion
approach to joint pattern alignment. In NIPS.
Wang, H., Li, S. Z., Wang, Y., and jun Zhang, J. (2004). Self
quotient image for face recognition. In International
Conference on Image Processing (ICIP), pages 1397–
1400. IEEE.
Xue, Y. and Liu, X. (2012). Image congealign via efficient
feature selection. Applications of Computer Vision
(WACV).
Zollei, L. (2006). A Unified Information Theoretic Frame-
work for Pair- and Group-wise Registration of Medi-
cal Images. PhD thesis, MIT Computer Science and
Artificial Intelligence Laboratory.
A Least Squares based Groupwise Image Registration Technique
127