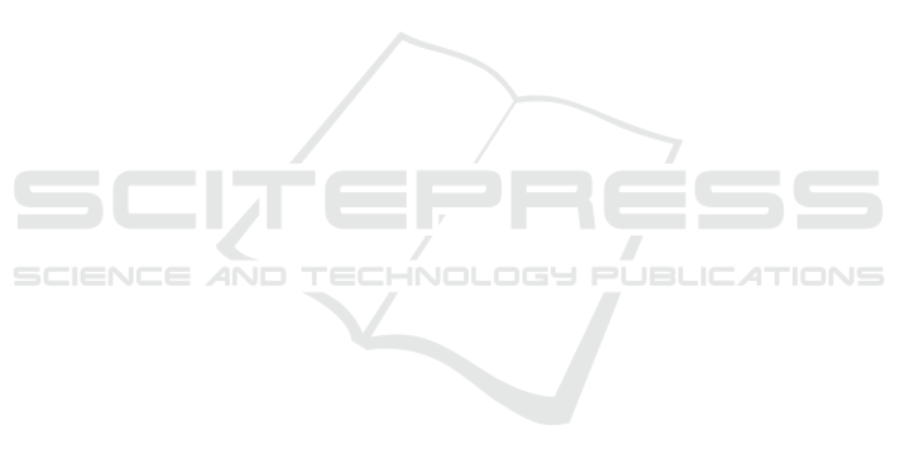
3D sensing technologies for that purpose and com-
pared 3D and 2D variants of state-of-the-art neural
networks trained on the data collected from a straw-
berry growing farm. These results show encouraging
performance but also allow us to highlight the limita-
tions of current technologies and algorithms. Time-
of-Flight technology, despite its superior quality of
point clouds and shape information, struggles with re-
flective surfaces resulting in a large number of false
detections, while stereo technology, lacking detail in
acquired depth, fails to detect numerous fruits. Tradi-
tional 2D image-based convolutional neural networks
still outperform the 3D networks for the task of fruit
segmentation and therefore are more suited for this
task. This work can be treated as a baseline for fu-
ture work on 3D information for outdoor applications
such as robotic fruit picking and should encourage re-
searchers to pursue more experimentation in such dif-
ficult to counteract limitations found in the paper and
bridge the gap with state-of-the-art techniques in per-
ception for 2D information.
REFERENCES
Armeni, I., Sax, A., Zamir, A. R., and Savarese, S. (2017).
Joint 2D-3D-Semantic Data for Indoor Scene Under-
standing. ArXiv e-prints.
Badrinarayanan, V., Kendall, A., and Cipolla, R. (2015).
SegNet: A Deep Convolutional Encoder-Decoder Ar-
chitecture for Image Segmentation. arXiv e-prints.
Bargoti, S. and Underwood, J. (2016). Deep Fruit Detection
in Orchards. arXiv e-prints.
Barnea, E., Mairon, R., and Ben-Shahar, O. (2016). Colour-
agnostic shape-based 3d fruit detection for crop har-
vesting robots. Biosystems Engineering, 146:57 – 70.
Special Issue: Advances in Robotic Agriculture for
Crops.
Cohen, J. (1960). A coefficient of agreement for nominal
scales. Educational and Psychological Measurement,
20(1):37–46.
Dai, A., Chang, A. X., Savva, M., Halber, M., Funkhouser,
T., and Nießner, M. (2017). ScanNet: Richly-
annotated 3D Reconstructions of Indoor Scenes.
In Proc. Computer Vision and Pattern Recognition
(CVPR), IEEE.
Everingham, M., Gool, L., Williams, C. K., Winn, J.,
and Zisserman, A. (2010). The Pascal Visual Ob-
ject Classes (VOC) Challenge. Int. J. Comput. Vision,
88(2):303–338.
Geiger, A., Lenz, P., and Urtasun, R. (2012). Are we ready
for Autonomous Driving? The KITTI Vision Bench-
mark Suite. In Conference on Computer Vision and
Pattern Recognition (CVPR).
Georg Halmetschlager-Funek, Markus Suchi, M. K. and
Vincze, M. (2018). An empirical evaluation of ten
depth cameras. IEEE Robotics and automation maga-
zine.
Grimstad, L. and From, P. J. (2017). The Thorvald II Agri-
cultural Robotic System. Robotics, 6(4).
Hackel, T., Savinov, N., Ladicky, L., Wegner, J. D.,
Schindler, K., and Pollefeys, M. (2017). SEMAN-
TIC3D.NET: A new large-scale point cloud classifica-
tion benchmark. In ISPRS Annals of the Photogram-
metry, Remote Sensing and Spatial Information Sci-
ences, volume IV-1-W1, pages 91–98.
Jiang, M., Wu, Y., Zhao, T., Zhao, Z., and Lu, C. (2018).
PointSIFT: A SIFT-like Network Module for 3D Point
Cloud Semantic Segmentation. arXiv e-prints.
Kazmi, W., Foix, S., Aleny, G., and Andersen, H. J. (2014).
Indoor and outdoor depth imaging of leaves with time-
of-flight and stereo vision sensors: Analysis and com-
parison. ISPRS Journal of Photogrammetry and Re-
mote Sensing, 88:128–146.
Lehnert, C., English, A., McCool, C., Tow, A., and Perez,
T. (2017). Autonomous Sweet Pepper Harvesting for
Protected Cropping Systems. arXiv e-prints.
Lehnert, C., McCool, C., Sa, I., and Perez, T. (2018). A
Sweet Pepper Harvesting Robot for Protected Crop-
ping Environments. arXiv e-prints.
Li, Y., Bu, R., Sun, M., and Chen, B. (2018). PointCNN:
Convolution On X-Transformed Points. arXiv
preprint arXiv:1801.07791.
Noraky, J. and Sze, V. (2018). Low Power Depth Estimation
of Rigid Objects for Time-of-Flight Imaging. arXiv e-
prints.
Pham, Q.-H., Thanh Nguyen, D., Hua, B.-S., Roig, G.,
and Yeung, S.-K. (2019). JSIS3D: Joint Semantic-
Instance Segmentation of 3D Point Clouds with Multi-
Task Pointwise Networks and Multi-Value Condi-
tional Random Fields. arXiv e-prints.
Qi, C. R., Su, H., Mo, K., and Guibas, L. J. (2017a). Point-
Net: Deep learning on point sets for 3d classification
and segmentation. Proc. Computer Vision and Pattern
Recognition (CVPR), IEEE, 1(2):4.
Qi, C. R., Yi, L., Su, H., and Guibas, L. J. (2017b). Point-
Net++: Deep hierarchical feature learning on point
sets in a metric space. In Advances in Neural Infor-
mation Processing Systems, pages 5099–5108.
Wang, W., Yu, R., Huang, Q., and Neumann, U. (2017).
SGPN: Similarity Group Proposal Network for 3D
Point Cloud Instance Segmentation. arXiv e-prints.
Wang, X., Liu, S., Shen, X., Shen, C., and Jia, J. (2019).
Associatively Segmenting Instances and Semantics in
Point Clouds. arXiv e-prints.
Yang, B., Wang, J., Clark, R., Hu, Q., Wang, S., Markham,
A., and Trigoni, N. (2019). Learning Object Bounding
Boxes for 3D Instance Segmentation on Point Clouds.
arXiv e-prints.
Yoshida, T., Fukao, T., , and Hasegawa, T. (2018). Fast De-
tection of Tomato Peduncle Using Point Cloud with
a Harvesting Robot. Journal of Robotics and Mecha-
tronics, 30(2):180–186.
Evaluation of 3D Vision Systems for Detection of Small Objects in Agricultural Environments
689