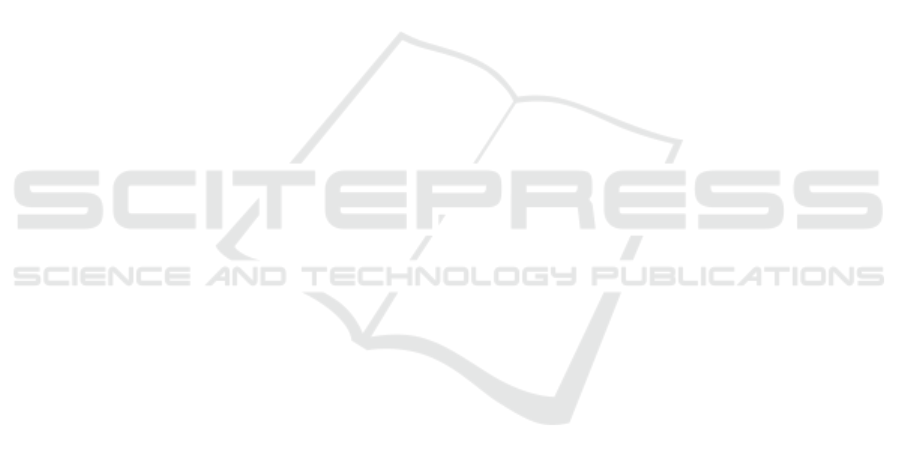
though being able to recognize eating behavior with
accuracy exceeding 90% automatically, cannot assess
specific meal-related activities such as the number of
chewing, drinking, or swallowing as our method does
(Bi et al., 2018; Zhang and Amft, 2018).
Further validation of the proposed classification
method may be validated further by comparing with
more types of classifier such as neural networks,
Bayesian models, and random forest. Indeed, SVM
requires normalization to deal correctly with individ-
ual differences, which may be an issue to guarantee
reliability to new users. Moreover, real-time perfor-
mances when running the model on a smartphone,
for example, should be verified. Finally, the robust-
ness of the model generalization to other types of eat-
ing sounds should be verified. For example, the level
of environmental noise from the smartphone record-
ing may affect classification capability, though in our
former study we shown that noise (tongue mixing
the food, etc.) could be accurately classified (Kondo
et al., 2019a).
As a prospect, we plan to use the proposed clas-
sification model to classify mastication, swallowing
food, swallowing drink, and utterance in real time
using bone conduction microphone and smartphone.
In realizing this, it is necessary to design a system
that automatically extracts sound data segments that
can be considered to be whether chewing, swallow-
ing, drinking or utterance in real-time. Besides, it is
also necessary to add the other sounds such as noises
in the model so that it is more robust to natural meal
environment.
ACKNOWLEDGEMENTS
This research was supported by Lotte Research Pro-
motion Grant. Entire experimental protocols were ap-
proved by the ethics committee of Aoyama Gakuin
University.
REFERENCES
Amft, O., St
¨
ager, M., Lukowicz, P., and Tr
¨
oster, G. (2005).
Analysis of chewing sounds for dietary monitoring. In
UbiComp 2005, 7th Int. Conf. on Ubiquitous Comput-
ing, pages 52–72.
Bi, S., Wang, T., Tobias, N., Nordrum, J., Wang, S.,
Halvorsen, G., Sen, S., Peterson, R., Odame, K.,
Caine, K., et al. (2018). Auracle: Detecting eating
episodes with an ear-mounted sensor. Proceedings of
the ACM on Interact., Mob., Wearable and Ubiquitous
Technol., 2(3):1–27.
Boersma, P. (2001). Praat, a system for doing phonetics by
computer. Glot International, 5(9/10):341–345.
Denney-Wilson, E. and Campbell, K. J. (2008). Eating be-
haviour and obesity. BMJ, 337:73–75.
Faudot, T., Lopez, G., and Yamada, I. (2010). Information
system for mapping wearable sensors into healthcare
services: Application to dietary habits monitoring. In
WIVE 2010, 2nd International Workshop on Web In-
tellgence and Virtual Enterprises, pages 1–9.
Fontana, J. and Sazonov, E. (2013). Evaluation of chew-
ing and swallowing sensors for monitoring ingestive
behavior. Sensor letters, 11(3):560–565.
Kao (2007). The effect of chewing well, tasting and eating -
preventive measures against metabolic syndrome and
obesity. In Kao Health Care Report, number 19, pages
4–5. (in Japanese).
Keum, S. C., Sarnab, B., and Edison, T. (2018). Detecting
eating episodes by tracking jawbone movements with
a non-contact wearable sensor. Proc. ACM Interact.
Mob. Wearable Ubiquitous Technol., 2(1):1–21.
Kishida, N. and Kamimura, Y. (1993). Relationship of con-
versation during meal and health and dietary life of
school children. The Japanese Journal of Nutririon
and Dietetics, 51(1):23–30.
Kohyama, K., Mioche, L., and Bourdio, P. (2003). Influence
of age and dental status on chewing behavior stud-
ied by emg recordings during consumption of various
food samples. Gerontology, 20(1):15–23.
Kondo, T., Haruka, K., Shun, I., Yokokubo, A., and
Lopez, G. (2019a). Robust classification of eating
sound collected in natural meal environment. In Ubi-
Comp/ISWC ’19, pages 105–108.
Kondo, T., Shiro, H., Yokokubo, A., and Lopez, G. (2019b).
Optimized classification model for efficient recogni-
tion of meal-related activities in daily life meal envi-
ronment. In 3rd IVPR, pages 146–151.
Li, J., Zhang, N., Hu, L., Ki, Z., Li, R., Li, C., and Wang,
S. (2011). Improvement in chewing activity reduces
energy intake in one meal and modulates plasma gut
hormone concentrations in obese and lean young chi-
nese men. American Journal of Clinical Nutrition,
94(3):709–716.
Logemann, J. (2014). Critical factors in the oral control
needed for chewing and swallowing. Journal of tex-
ture studies, 45(3):173–179.
Lopez, G., Mitsui, H., Ohara, J., and Yokokubo, A. (2019).
Effect of feedback medium for real-time awareness in-
crease using wearable sensors. In HEALTHINF 2019.
MHLW (2016). The national health and nutrition survey
in japan, 2014. Japan Ministry of Health Labor and
Welfare. (in Japanese).
Mizuno, H., Nagai, H., Sasaki, K., Hosaka, H., Sugimoto,
C., and Tatsuta, S. (2007). Wearable sensor sys-
tem for human behavior recognition -first report: Ba-
sic architecture and behavior prediction method. In
Transducers 2007, The 14th International Conference
on Solid-State Sensors, Actuators and Microsystems,
pages 435–438.
Nicklas, T., Baranowski, T., Cullen, K., and Berenson,
G. (2001). Eating patterns, dietary quality and obe-
HEALTHINF 2020 - 13th International Conference on Health Informatics
290