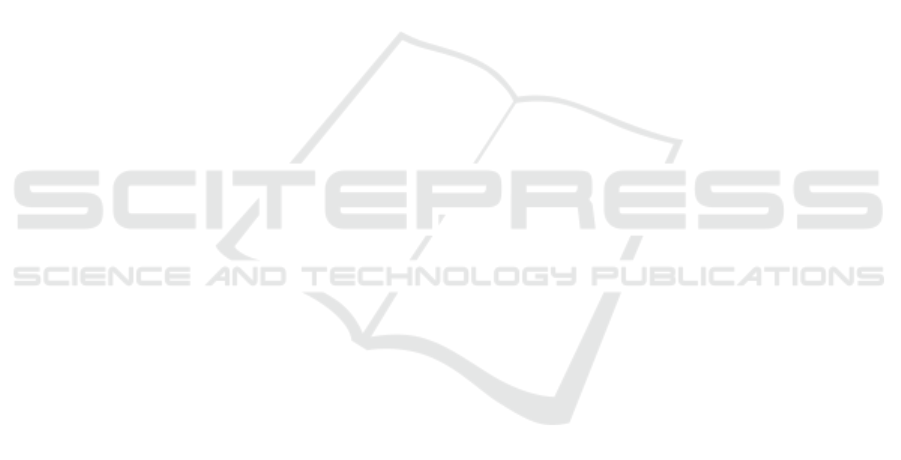
REFERENCES
Bojani
´
c, D., Bartol, K., Pribani
´
c, T., Petkovi
´
c, T., Donoso,
Y. D., and Mas, J. S. (2019). On the comparison
of classic and deep keypoint detector and descriptor
methods. 11th Int’l Symposium on Image and Signal
Processing and Analysis.
Cordts, M., Omran, M., Ramos, S., Rehfeld, T., Enzweiler,
M., Benenson, R., Franke, U., Roth, S., and Schiele,
B. (2016). The cityscapes dataset for semantic urban
scene understanding. CoRR, abs/1604.01685.
Furukawa, Y. and Hern
´
andez, C. (2015). Multi-view stereo:
A tutorial. Foundations and Trends in Computer
Graphics and Vision.
Garg, R., G, V. K. B., and Reid, I. D. (2016). Unsupervised
CNN for single view depth estimation: Geometry to
the rescue. CoRR, abs/1603.04992.
Geiger, A., Lenz, P., Stiller, C., and Urtasun, R. (2013).
Vision meets robotics: The kitti dataset. I. J. Robotic
Res., 32(11):1231–1237.
Godard, C., Aodha, O. M., Firman, M., and Brostow, G.
(2018). Digging into self-supervised monocular depth
estimation. The International Conference on Com-
puter Vision (ICCV).
Godard, C., Mac Aodha, O., and Brostow, G. J. (2016). Un-
supervised monocular depth estimation with left-right
consistency. CoRR, abs/1609.03677.
Hartley, R. and Zisserman, A. (2003). Multiple View Geom-
etry in Computer Vision. Cambridge University Press,
USA, 2 edition.
Li, W., Saeedi, S., McCormac, J., Clark, R., Tzoumanikas,
D., Ye, Q., Huang, Y., Tang, R., and Leutenegger, S.
(2018). Interiornet: Mega-scale multi-sensor photo-
realistic indoor scenes dataset. In British Machine Vi-
sion Conference (BMVC).
Lowe, D. G. (2004). Distinctive image features from scale-
invariant keypoints. International Journal of Com-
puter Vision.
Mahjourian, R., Wicke, M., and Jun, C. (2017). Unsuper-
vised learning of depth and ego-motion from video.
CVPR.
Ono, Y., Trulls, E., Fua, P., and Yi, K. M. (2018). Lf-
net: Learning local features from images. CoRR,
abs/1805.09662.
Paszke, A., Gross, S., Massa, F., Lerer, A., Bradbury, J.,
Chanan, G., Killeen, T., Lin, Z., Gimelshein, N.,
Antiga, L., Desmaison, A., Kopf, A., Yang, E., De-
Vito, Z., Raison, M., Tejani, A., Chilamkurthy, S.,
Steiner, B., Fang, L., Bai, J., and Chintala, S. (2019).
Pytorch: An imperative style, high-performance deep
learning library. In Wallach, H., Larochelle, H.,
Beygelzimer, A., d'Alch
´
e-Buc, F., Fox, E., and Gar-
nett, R., editors, Advances in Neural Information Pro-
cessing Systems 32, pages 8024–8035. Curran Asso-
ciates, Inc.
Sch
¨
onberger, J. L. and Frahm, J.-M. (2016). Structure-
from-motion revisited. 2016 IEEE Conference on
Computer Vision and Pattern Recognition (CVPR),
pages 4104–4113.
Towards Keypoint Guided Self-Supervised Depth Estimation
589