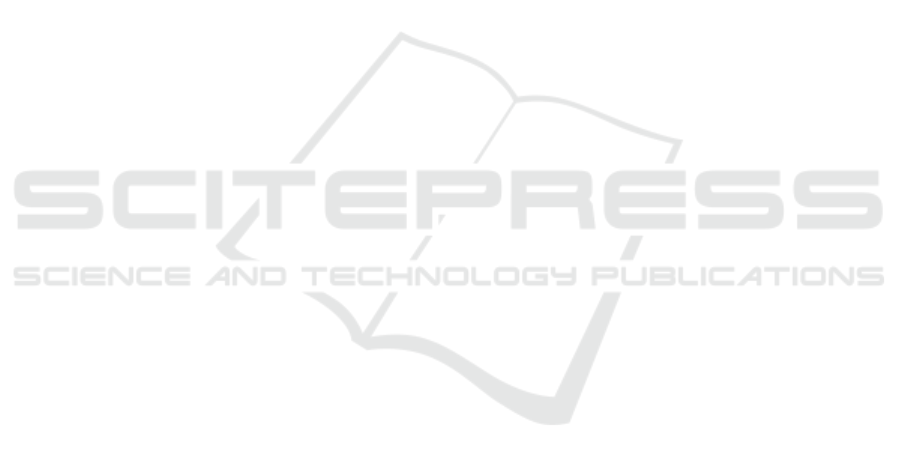
REFERENCES
Andriluka, M., Pishchulin, L., Gehler, P., and Schiele, B.
(2014). 2D human pose estimation: New benchmark
and state of the art analysis. In Proceedings of the
IEEE Computer Society Conference on Computer Vi-
sion and Pattern Recognition.
Bastioni, M. (2001). Makehuman, Open Source tool for
making 3D characters.
Borges, J., Queir
´
os, S., Oliveira, B., Torres, H., Rodrigues,
N., Coelho, V., Pallauf, J., Henrique Brito, J., Mendes,
J., and C. Fonseca, J. (2019). MoLa R10k InCar
Dataset.
Borghi, G., Venturelli, M., Vezzani, R., and Cucchiara,
R. (2017). POSEidon: Face-from-Depth for driver
pose estimation. Proceedings - 30th IEEE Conference
on Computer Vision and Pattern Recognition, CVPR
2017, 2017-Janua:5494–5503.
Cao, Z., Simon, T., Wei, S. E., and Sheikh, Y. (2017).
Realtime multi-person 2D pose estimation using part
affinity fields. Proceedings - 30th IEEE Conference
on Computer Vision and Pattern Recognition, CVPR
2017, 2017-Janua:1302–1310.
Carlucci, F. M., Russo, P., and Caputo, B. (2017). A
deep representation for depth images from synthetic
data. Proceedings - IEEE International Conference
on Robotics and Automation, pages 1362–1369.
CMU (2016). CMU Dataset @ mocap.cs.cmu.edu.
Gatys, L. A., Ecker, A. S., Bethge, M., Hertzmann, A., and
Shechtman, E. (2017). Controlling perceptual factors
in neural style transfer. In Proceedings - 30th IEEE
Conference on Computer Vision and Pattern Recog-
nition, CVPR 2017, volume 2017-Janua, pages 3730–
3738.
Ionescu, C., Papava, D., Olaru, V., and Sminchisescu, C.
(2014). Human3.6M: Large scale datasets and pre-
dictive methods for 3D human sensing in natural en-
vironments. IEEE Transactions on Pattern Analysis
and Machine Intelligence, 36(7):1325–1339.
Iversen, T. and Kraft, D. (2017). Generation of synthetic
Kinect depth images based on empirical noise model.
Electronics Letters, 53(13).
Liu, X., Liang, W., Wang, Y., Li, S., and Pei, M. (2016).
3D head pose estimation with convolutional neural
network trained on synthetic images. Proceedings -
International Conference on Image Processing, ICIP,
2016-Augus:1289–1293.
Loper, M., Mahmood, N., Romero, J., Pons-moll, G., and
Black, M. J. (2015). SMPL : A Skinned Multi-Person
Linear Model. ACM Trans. Graphics (Proc. SIG-
GRAPH Asia), 34(6):248:1—-248:16.
Martinez, J., Hossain, R., Romero, J., and Little, J. J.
(2017). A Simple Yet Effective Baseline for 3d Hu-
man Pose Estimation. In Proceedings of the IEEE In-
ternational Conference on Computer Vision.
National Aeronautics and Space Administration (2000).
Anthropometry and Biomechanics. Man-Systems In-
tegration Standards NASA-STD-3000, 1(1):16–45.
Pini, S., Grazioli, F., Borghi, G., Vezzani, R., and Cuc-
chiara, R. (2018). Learning to generate facial depth
maps. Proceedings - 2018 International Conference
on 3D Vision, 3DV 2018, pages 634–642.
Rodrigues, N., Torres, H., Oliveira, B., Borges, J., Queir
´
os,
S., Mendes, J., Fonseca, J., Coelho, V., and Brito, J. H.
(2019). Top-down human pose estimation with depth
images and domain adaptation. In VISIGRAPP 2019
- Proceedings of the 14th International Joint Con-
ference on Computer Vision, Imaging and Computer
Graphics Theory and Applications, volume 5, pages
281–288.
Ronneberger, O., Fischer, P., and Brox, T. (2015). U-
net: Convolutional networks for biomedical im-
age segmentation. Lecture Notes in Computer Sci-
ence (including subseries Lecture Notes in Artificial
Intelligence and Lecture Notes in Bioinformatics),
9351:234–241.
Ros, G., Sellart, L., Materzynska, J., Vazquez, D., and
Lopez, A. M. (2016). The SYNTHIA Dataset: A
Large Collection of Synthetic Images for Semantic
Segmentation of Urban Scenes. 2016 IEEE Con-
ference on Computer Vision and Pattern Recognition
(CVPR), (600388):3234–3243.
Shotton, J., Fitzgibbon, A., Cook, M., Sharp, T., Finocchio,
M., Moore, R., Kipman, A., and Blake, A. (2013).
Real-time human pose recognition in parts from single
depth images. Studies in Computational Intelligence,
411:119–135.
Sigal, L., Balan, A. O., and Black, M. J. (2010). HumanEva:
Synchronized video and motion capture dataset and
baseline algorithm for evaluation of articulated human
motion. International Journal of Computer Vision,
87(1-2):4–27.
Torres, H. R., Oliveira, B., Fonseca, J., Queirós,
S., Borges, J., Rodrigues, N., Coelho, V., Pallauf, J.,
Brito, J., and Mendes, J. (2019). Real-Time Human
Body Pose Estimation for In-Car Depth Images. In
IFIP Advances in Information and Communication
Technology, volume 553, pages 169–182. Springer
New York LLC.
Varol, G., Romero, J., Martin, X., Mahmood, N., Black,
M. J., Laptev, I., and Schmid, C. (2017). Learn-
ing from synthetic humans. Proceedings - 30th IEEE
Conference on Computer Vision and Pattern Recogni-
tion, CVPR 2017, 2017-Janua:4627–4635.
Automated Generation of Synthetic in-Car Dataset for Human Body Pose Detection
557