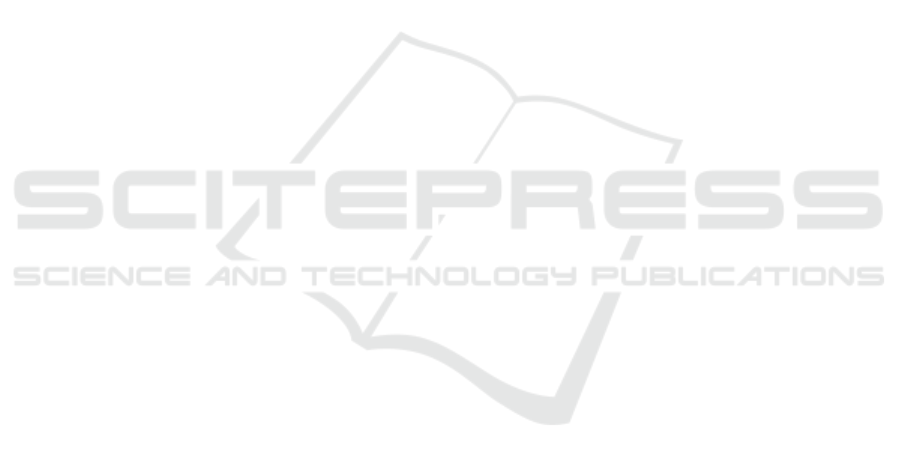
in layers or other topologies to enable unsupervised
analysis of more complex motion patterns.
ACKNOWLEDGEMENTS
This work has been partly funded by IRCICA (Univ.
Lille, CNRS, USR 3380 IRCICA, Lille, France).
REFERENCES
Amir, A., Taba, B., Berg, D. J., Melano, T., McKinstry,
J. L., Di Nolfo, C., Nayak, T. K., Andreopoulos, A.,
Garreau, G., Mendoza, M., et al. (2017). A low
power, fully event-based gesture recognition system.
In CVPR, pages 7388–7397.
Bichler, O., Querlioz, D., Thorpe, S. J., Bourgoin, J.-P.,
and Gamrat, C. (2012). Extraction of temporally
correlated features from dynamic vision sensors with
spike-timing-dependent plasticity. Neural Networks,
32:339–348.
Cao, Y., Chen, Y., and Khosla, D. (2015). Spiking deep
convolutional neural networks for energy-efficient ob-
ject recognition. International Journal of Computer
Vision, 113(1):54–66.
Goodman, D. F. and Brette, R. (2009). The brian simulator.
Frontiers in neuroscience, 3:26.
Hopkins, M., Pineda-Garc
´
ıa, G., Bogdan, P. A., and Furber,
S. B. (2018). Spiking neural networks for computer
vision. Interface Focus, 8(4):20180007.
Ilg, E., Mayer, N., Saikia, T., Keuper, M., Dosovitskiy, A.,
and Brox, T. (2017). Flownet 2.0: Evolution of optical
flow estimation with deep networks. In CVPR 2017,
pages 1647–1655. IEEE.
Kreiser, R., Moraitis, T., Sandamirskaya, Y., and Indiveri,
G. (2017). On-chip unsupervised learning in winner-
take-all networks of spiking neurons. In Biomedi-
cal Circuits and Systems Conference (BioCAS), 2017
IEEE, pages 1–4. IEEE.
Lee, J. H., Delbruck, T., and Pfeiffer, M. (2016). Training
deep spiking neural networks using backpropagation.
Frontiers in neuroscience, 10:508.
Li, H., Liu, H., Ji, X., Li, G., and Shi, L. (2017). Cifar10-
dvs: an event-stream dataset for object classification.
Frontiers in neuroscience, 11:309.
Lichtsteiner, P., Posch, C., and Delbruck, T. (2008). A
128×128 120 db 15µ s latency asynchronous tempo-
ral contrast vision sensor. IEEE journal of solid-state
circuits, 43(2):566–576.
Maass, W. (1997). Networks of spiking neurons: the third
generation of neural network models. Neural net-
works, 10(9):1659–1671.
Masquelier, T. and Thorpe, S. J. (2007). Unsupervised
learning of visual features through spike timing de-
pendent plasticity. PLoS computational biology,
3(2):e31.
Merolla, P. A., Arthur, J. V., Alvarez-Icaza, R., Cassidy,
A. S., Sawada, J., Akopyan, F., Jackson, B. L., Imam,
N., Guo, C., Nakamura, Y., et al. (2014). A mil-
lion spiking-neuron integrated circuit with a scal-
able communication network and interface. Science,
345(6197):668–673.
Orchard, G., Benosman, R., Etienne-Cummings, R., and
Thakor, N. V. (2013). A spiking neural network ar-
chitecture for visual motion estimation. In Biomedi-
cal Circuits and Systems Conference (BioCAS), 2013
IEEE, pages 298–301. IEEE.
Orchard, G., Jayawant, A., Cohen, G. K., and Thakor, N.
(2015). Converting static image datasets to spiking
neuromorphic datasets using saccades. Frontiers in
neuroscience, 9:437.
Oudjail, V. and Martinet, J. (2019). Bio-inspired event-
based motion analysis with spiking neural networks.
In VISAPP 2019, pages 389–394.
Paugam-Moisy, H. and Bohte, S. (2012). Computing with
spiking neuron networks. In Handbook of natural
computing, pages 335–376. Springer.
Ponulak, F. and Kasinski, A. (2011). Introduction to spik-
ing neural networks: Information processing, learning
and applications. Acta neurobiologiae experimentalis,
71(4):409–433.
Serrano-Gotarredona, T. and Linares-Barranco, B. (2015).
Poker-dvs and mnist-dvs. their history, how they were
made, and other details. Frontiers in neuroscience,
9:481.
Sourikopoulos, I., Hedayat, S., Loyez, C., Danneville, F.,
Hoel, V., Mercier, E., and Cappy, A. (2017). A 4-
fj/spike artificial neuron in 65 nm cmos technology.
Frontiers in neuroscience, 11:123.
Tavanaei, A. and Maida, A. S. (2017). Multi-layer unsuper-
vised learning in a spiking convolutional neural net-
work. In IJCNN 2017, pages 2023–2030. IEEE.
Zhao, B., Ding, R., Chen, S., Linares-Barranco, B., and
Tang, H. (2015). Feedforward categorization on aer
motion events using cortex-like features in a spiking
neural network. IEEE Trans. Neural Netw. Learning
Syst., 26(9):1963–1978.
VISAPP 2020 - 15th International Conference on Computer Vision Theory and Applications
860