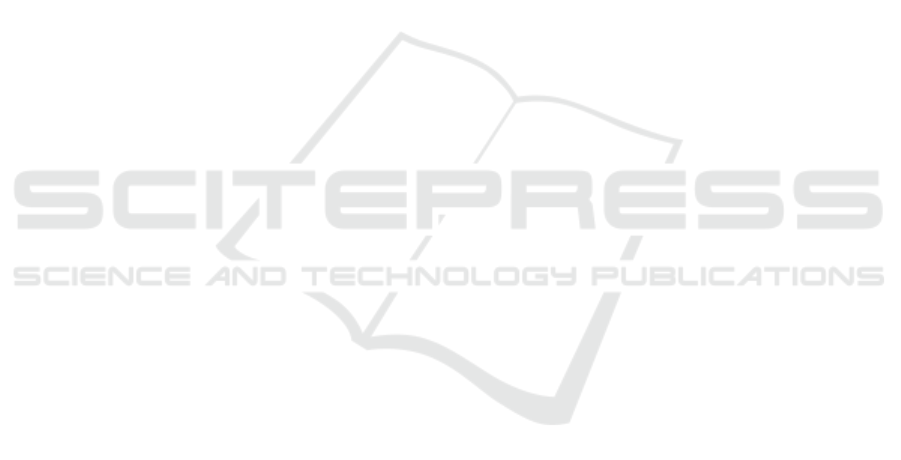
national Workshop on Crowd Simulation, volume 3,
pages 71–80.
Basak, B. and Gupta, S. (2017). Developing an agent-
based model for pilgrim evacuation using visual in-
telligence: A case study of ratha yatra at puri. Com-
puters, Environment and Urban Systems, 64:118–131.
Coffey, W. T. and Kalmykov, Y. P. (2004). The Langevin
equation: with applications to stochastic problems in
physics, chemistry and electrical engineering. World
Scientific.
Junior, J. C. S. J., Musse, S. R., and Jung, C. R. (2010).
Crowd analysis using computer vision techniques.
IEEE Signal Processing Magazine, 27(5):66–77.
Langevin, P. (1908). Sur la th
´
eorie du mouvement brown-
ien. CR Acad. Sci. Paris, 146:530–533.
Lim, M. K., Chan, C. S., Monekosso, D., and Remagnino,
P. (2014). Detection of salient regions in crowded
scenes. Electronics Letters, 50(5):363–365.
Lin, W., Chu, H., Wu, J., Sheng, B., and Chen, Z. (2013). A
heat-map-based algorithm for recognizing group ac-
tivities in videos. IEEE Transactions on Circuits and
Systems for Video Technology, 23(11):1980–1992.
Mehran, R., Moore, B. E., and Shah, M. (2010). A streak-
line representation of flow in crowded scenes. In Euro-
pean Conference on Computer Vision, pages 439–452.
Springer.
Monaghan, J. J. (1992). Smoothed particle hydrodynam-
ics. Annual review of astronomy and astrophysics,
30(1):543–574.
Schweitzer, F. (2007). Brownian agents and active par-
ticles: collective dynamics in the natural and social
sciences. Springer.
Shao, J., Change Loy, C., and Wang, X. (2014). Scene-
independent group profiling in crowd. In Proceedings
of the IEEE Conference on Computer Vision and Pat-
tern Recognition, pages 2219–2226.
Su, H., Yang, H., Zheng, S., Fan, Y., and Wei, S. (2013).
The large-scale crowd behavior perception based on
spatio-temporal viscous fluid field. IEEE Transactions
on Information Forensics and Security, 8(10):1575–
1589.
Ullah, H., Ullah, M., and Uzair, M. (2018). A hybrid social
influence model for pedestrian motion segmentation.
Neural Computing and Applications, pages 1–17.
Ullah, H., Uzair, M., Ullah, M., Khan, A., Ahmad, A., and
Khan, W. (2017). Density independent hydrodynam-
ics model for crowd coherency detection. Neurocom-
puting, 242:28–39.
Wu, S., Su, H., Yang, H., Zheng, S., Fan, Y., and Zhou, Q.
(2017). Bilinear dynamics for crowd video analysis.
Journal of Visual Communication and Image Repre-
sentation, 48:461–470.
Zhang, D., Xu, J., Sun, M., and Xiang, Z. (2017). High-
density crowd behaviors segmentation based on dy-
namical systems. Multimedia Systems, 23(5):599–
606.
Zhang, X., Yu, Q., and Yu, H. (2018). Physics inspired
methods for crowd video surveillance and analysis: a
survey. IEEE Access.
Zhang, Y., Qin, L., Ji, R., Yao, H., and Huang, Q. (2015).
Social attribute-aware force model: exploiting rich-
ness of interaction for abnormal crowd detection.
IEEE Transactions on Circuits and Systems for Video
Technology, 25(7):1231–1245.
Segmentation and Visualization of Crowd Flows in Videos using Hybrid Force Model
867