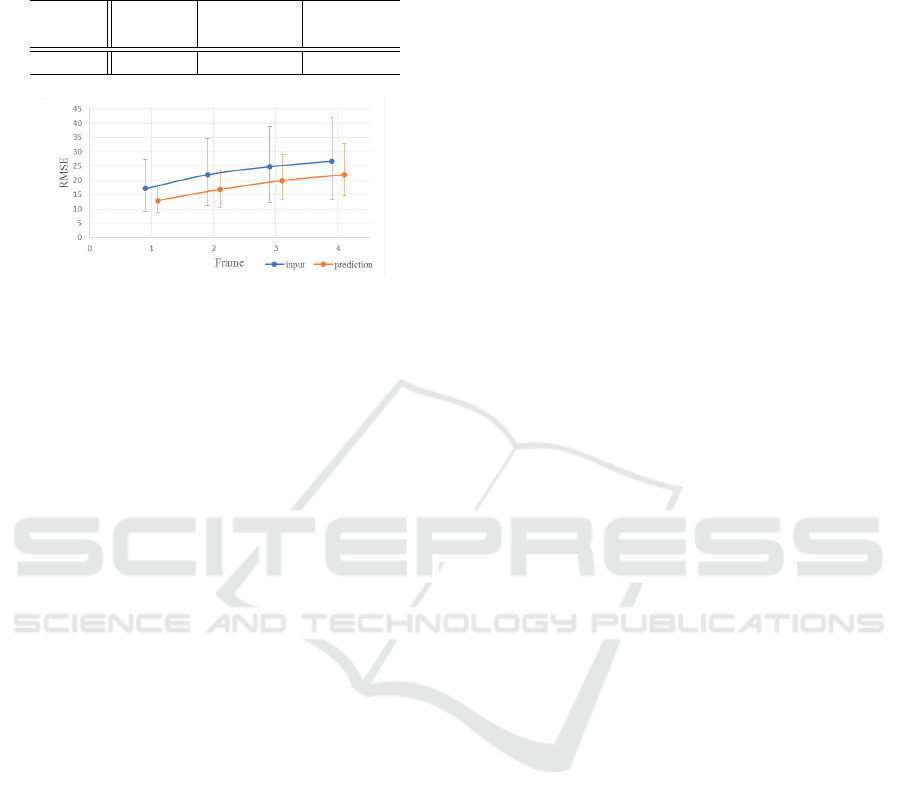
Table 1: RMSEs from the ground-truth for original input
image, predicted images without inpainting and predicted
images with inpainting.
original without predicted
inpainting image
RMSE 16.04 11.57 10.77
Figure 8: RMSE between ground-truth and predicted/input
images.
images were computed. Table 1 shows computed
RMSEs for original input images, predicted images
without image inpainting, and predicted images with
inpainting. The table shows that the RMSE decreases
with object motion prediction and image inpainting.
The results indicate that the image inpainting tech-
nique provides better results for video prediction.
We next synthesized predicted images from 1∼4
ago images, respectively. We computed RMSEs be-
tween ground-truth and the predicted images. Fig-
ure 8 shows average RMSE for each result. In this
figure, error bars for each point show the minimum
and the maximum error. For comparison, RMSE be-
tween the input images and ground-truth are shown by
an orange line. In this result, RMSEs of predicted re-
sults always are lower than ones of input images. The
results show that our proposed method can predict fu-
ture images for various images. Note that the min-
imum errors for input images and predicted images
are mostly the same since the data includes mostly
static sequences. This fact indicates that our proposed
method can predict future images for static sequences
as well as dynamic sequences.
5 CONCLUSION
In this paper, we proposed a future image prediction
method from a stereo image in a driving scene. In this
method, 3D shapes in the scene are reconstructed us-
ing the stereo method, and the reconstructed shapes
are separated into multiple objects by semantic image
segmentation. In the motion estimation of each sep-
arated object, the Kalman filter is used, and the filter
predicts the future condition of the objects. From the
conditions of the predicted objects, future images are
rendered. Furthermore, the deep image prior is ap-
plied to the predicted images to interpolate the miss-
ing areas of the images caused by occlusion, and we
finally predict the future natural images without miss-
ing areas. Several experimental results of a public
dataset show that our proposed method can predict fu-
ture images even when the input scene includes mul-
tiple objects moving independently.
REFERENCES
Cordts, M., Omran, M., Ramos, S., Rehfeld, T., Enzweiler,
M., Benenson, R., Franke, U., Roth, S., and Schiele,
B. (2016). The cityscapes dataset for semantic urban
scene understanding. In Proceedings of the IEEE con-
ference on computer vision and pattern recognition,
pages 3213–3223.
Finn, C., Goodfellow, I., and Levine, S. (2016). Unsuper-
vised learning for physical interaction through video
prediction. In Advances in Neural Information Pro-
cessing Systems (NIPS), pages 64—-72.
Hirschmuller, H. (2005). Accurate and efficient stereo pro-
cessing by semi-global matching and mutual informa-
tion. In 2005 IEEE Computer Society Conference on
Computer Vision and Pattern Recognition (CVPR’05),
volume 2, pages 807–814 vol. 2.
Isola, P., Zhu, J.-Y., Zhou, T., and Efros, A. A. (2016).
Image-to-image translation with conditional adversar-
ial networks. arxiv.
Kalman, R. E. (1960). A new approach to linear filtering
and prediction problems. ASME Journal of Basic En-
gineering.
Lowe, D. G. (2004). Distinctive image features from scale-
invariant keypoints. International journal of computer
vision, 60(2):91–110.
Sharma, S., Ansari, J. A., Murthy, J. K., and Krishna, K. M.
(2018). Beyond pixels: Leveraging geometry and
shape cues for online multi-object tracking. In 2018
IEEE International Conference on Robotics and Au-
tomation (ICRA), pages 3508–3515. IEEE.
Ulyanov, D., Vedaldi, A., and Lempitsky, V. (2018). Deep
image prior. In Proceedings of the IEEE Conference
on Computer Vision and Pattern Recognition, pages
9446–9454.
Vondrick, C., Pirsiavash, H., and Torralba, A. (2016). Gen-
erating videos with scene dynamics. In In Advances In
Neural Information Processing Systems (NIPS), pages
613––621.
Vondrick, C. and Torralba, A. (2017). Generating the future
with adversarial transformers. In Proc. IEEE Con-
ference on Computer Vision and Pattern Recognition
(CVPR).
Yu, J., Lin, Z., Yang, J., Shen, X., Lu, X., and Huang, T.
(2018). Generative image inpainting with contextual
attention. In Proc. IEEE Conference on Computer Vi-
sion and Pattern Recognition (CVPR), pages 5505–
5514.
VISAPP 2020 - 15th International Conference on Computer Vision Theory and Applications
866