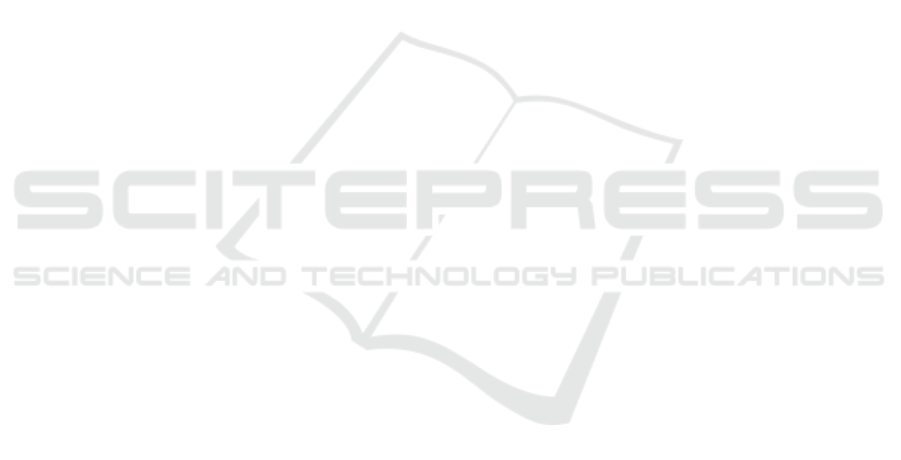
ACKNOWLEDGEMENTS
The authors acknowledge the generous support of the
Carinthian Government and the City of Klagenfurt
within the innovation center KI4Life.
REFERENCES
Alom, M. Z., Taha, T. M., Yakopcic, C., Westberg, S.,
Sidike, P., Nasrin, M. S., Hasan, M., Van Essen, B. C.,
Awwal, A. A. S., and Asari, V. K. (2019). A State-of-
the-art Survey on Deep Learning Theory and Archi-
tectures. Electronics, 8:292ff.
Brock, A., Lim, T., Ritchie, J. M., and Weston, N. (2016).
Generative and Discriminative Voxel Modeling with
Convolutional Neural Networks. International Con-
ference on Neural Information Processing Systems /
3D Deep Learning Workshop, 30:1–9.
Chatfield, K., Simonyan, K., Vedaldi, A., and Zisserman,
A. (2014). Return of the Devil in the Details: Delving
Deep into Convolutional Nets. British Machine Vision
Conference, 6:1–12.
Deng, J., Dong, W., Socher, R., Li, L.-J., Li, K., and Fei-Fei,
L. (2009). Imagenet: A Large-scale Hierarchical Im-
age Database. IEEE Conference on Computer Vision
and Pattern Recognition (CVPR), 12:248–255.
Elhoseiny, M., El-Gaaly, T., Bakry, A., and Elgammal,
A. M. (2016). A Comparative Analysis and Study of
Multiview CNN Models for Joint Object Categoriza-
tion and Pose Estimation. International Conference
on Machine Learning (ICML), 33:888–897.
Evans, G., Miller, J., Pena, M. I., MacAllister, A., and
Winer, E. (2017). Evaluating the Microsoft Hololens
through an Augmented Reality Assembly Applica-
tion. Degraded Environments: Sensing, Processing,
and Display (Proceedings of SPIE Defense and Secu-
rity), 10197:1–16.
H
¨
ane, C., Zach, C., Cohen, A., and Pollefeys, M. (2017).
Dense Semantic 3d Reconstruction. IEEE Transac-
tions on Pattern Analysis and Machine Intelligence,
39:1730–1743.
Hartley, R. and Zisserman, A. (2004). Multiple View Geom-
etry in Computer Vision. Cambridge University Press.
He, K., Zhang, X., Ren, S., and Sun, J. (2016). Deep
Residual Learning for Image Recognition. IEEE Con-
ference on Computer Vision and Pattern Recognition
(CVPR), 19:770–778.
Kanezaki, A., Matsushita, Y., and Nishida, Y. (2018). Ro-
tationNet: Joint Object Categorization and Pose Es-
timation Using Multiviews from Unsupervised View-
points. IEEE Conference on Computer Vision and Pat-
tern Recognition (CVPR), 21:5010–5019.
Kress, B. C. and Cummings, W. J. (2017). Towards the Ul-
timate Mixed Reality Experience: Hololens Display
Architecture Choices. Digest of Technical Papers, So-
ciety for Information Display, 48:127–131.
Krizhevsky, A., Sutskever, I., and Hinton, G. E. (2012). Im-
ageNet Classification with Deep Convolutional Neu-
ral Networks. International Conference on Neural In-
formation Processing Systems, 25:1097–1105.
Kuznietsov, Y., St
¨
uckler, J., and Leibe, B. (2017). Semi-
supervised Deep Learning for Monocular Depth Map
Prediction. IEEE Conference on Computer Vision and
Pattern Recognition (CVPR), 20:2215–2223.
Mahjourian, R., Wicke, M., and Angelova, A. (2018). Un-
supervised Learning of Depth and Ego-motion from
Monocular Video Using 3d Geometric Constraints.
IEEE Conference on Computer Vision and Pattern
Recognition (CVPR), 21:5667–5675.
Oliphant, T. E. (2006). A Bayesian perspective on esti-
mating mean, variance, and standard-deviation from
data. Brigham Young University (BYU) Faculty Pub-
lications, 1877-438:http://hdl.lib.byu.edu/1877/438.
Qi, C. R., Su, H., Nießner, M., Dai, A., Yan, M., and
Guibas, L. (2016). Volumetric and Multi-view CNNs
for Object Classification on 3D Data. IEEE Con-
ference on Computer Vision and Pattern Recognition
(CVPR), 19:5648–5656.
Russakovsky, O., Deng, J., Su, H., Krause, J., Satheesh,
S., Ma, S., Huang, Z., Karpathy, A., Khosla, A.,
Bernstein, M., Berg, A. C., and Fei-Fei, L. (2015).
ImageNet Large Scale Visual Recognition Challenge.
International Journal of Computer Vision (IJCV),
115:211–252.
Saxena, A., Chung, S. H., and Ng, A. Y. (2006). Learn-
ing Depth from Single Monocular Images. Advances
in Neural Information Processing Systems, 18:1161–
1168.
Schinko, C., Ullrich, T., and Fellner, D. W. (2011). Simple
and Efficient Normal Encoding with Error Bounds.
Theory and Practice of Computer Graphics, 29:63–
66.
Sfikas, K., Pratikakis, I., and Theoharis, T. (2018). Ensem-
ble of PANORAMA-based convolutional neural net-
works for 3D model classification and retrieval. Com-
puters & Graphics, 71:208–218.
Su, H., Maji, S., Kalogerakis, E., and Learned-Miller, E.
(2015). Multi-view Convolutional Neural Networks
for 3d Shape Recognition. IEEE International Con-
ference on Computer Vision (ICCV), 11:945–953.
Tao, F., Zhang, M., and Nee, A. Y. C. (2019). Digital Twin
Driven Smart Manufacturing. Academic Press.
Wu, Z., Song, S., Khosla, A., Yu, F., Zhang, L., Tang, X.,
and Xiao, J. (2015). 3d ShapeNets: A Deep Repre-
sentation for Volumetric Shapes. IEEE Conference
on Computer Vision and Pattern Recognition (CVPR),
18:1912–1920.
Zhou, T., Brown, M., Snavely, N., and Lowe, D. G. (2017).
Unsupervised Learning of Depth and Ego-Motion
from Video. IEEE Conference on Computer Vision
and Pattern Recognition (CVPR), 20:1851–1860.
HUCAPP 2020 - 4th International Conference on Human Computer Interaction Theory and Applications
238