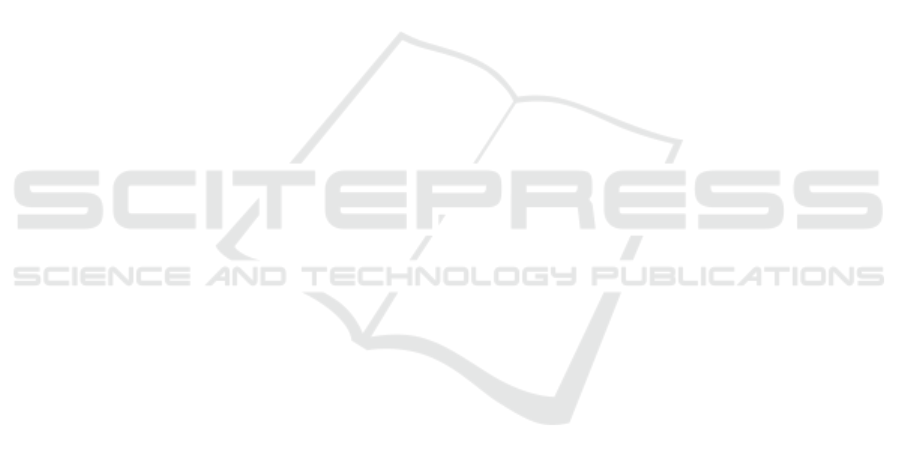
Guvenc, I., Koohifar, F., Singh, S., Sichitiu, M. L., and Ma-
tolak, D. (2018). Detection, tracking, and interdiction
for amateur drones. IEEE Communications Magazine,
56(4):75–81.
Han, J., Pei, J., and Kamber, M. (2011). Data mining: con-
cepts and techniques. Elsevier.
Hartmann, K. and Steup, C. (2013). The vulnerability of
uavs to cyber attacks-an approach to the risk assess-
ment. In 2013 5th international conference on cyber
conflict (CYCON 2013), pages 1–23. IEEE.
He, K., Gkioxari, G., Doll
´
ar, P., and Girshick, R. (2017).
Mask r-cnn. In Proceedings of the IEEE international
conference on computer vision, pages 2961–2969.
Jovanoska, S., Br
¨
otje, M., and Koch, W. (2018). Multisen-
sor data fusion for uav detection and tracking. In 2018
19th International Radar Symposium (IRS), pages 1–
10. IEEE.
Kerns, A. J., Shepard, D. P., Bhatti, J. A., and Humphreys,
T. E. (2014). Unmanned aircraft capture and con-
trol via gps spoofing. Journal of Field Robotics,
31(4):617–636.
Kim, I. S., Choi, H. S., Yi, K. M., Choi, J. Y., and Kong,
S. G. (2010). Intelligent visual surveillancea survey.
International Journal of Control, Automation and Sys-
tems, 8(5):926–939.
Korobiichuk, I., Danik, Y., Samchyshyn, O., Dupelich, S.,
and Kachniarz, M. (2019). The estimation algorithm
of operative capabilities of complex countermeasures
to resist uavs. Simulation, 95(6):569–573.
Kyrkou, C., Plastiras, G., Theocharides, T., Venieris, S. I.,
and Bouganis, C.-S. (2018). Dronet: Efficient con-
volutional neural network detector for real-time uav
applications. In 2018 Design, Automation & Test in
Europe Conference & Exhibition (DATE), pages 967–
972. IEEE.
Lin, T.-Y., Maire, M., Belongie, S., Hays, J., Perona, P.,
Ramanan, D., Doll
´
ar, P., and Zitnick, C. L. (2014).
Microsoft coco: Common objects in context. In Euro-
pean conference on computer vision, pages 740–755.
Springer.
Liu, J., Shahroudy, A., Perez, M. L., Wang, G., Duan, L.-Y.,
and Chichung, A. K. (2019). Ntu rgb+ d 120: A large-
scale benchmark for 3d human activity understanding.
IEEE transactions on pattern analysis and machine
intelligence.
May, R., Steinheim, Y., Kvaløy, P., Vang, R., and Hanssen,
F. (2017). Performance test and verification of an
off-the-shelf automated avian radar tracking system.
Ecology and evolution, 7(15):5930–5938.
Mazur, M., Wisniewski, A., and McMillan, J. (2016). Clar-
ity from above: Pwc global report on the commer-
cial applications of drone technology. Warsaw: Drone
Powered Solutions, PriceWater house Coopers.
Morris, B. T. and Trivedi, M. M. (2008). A survey of vision-
based trajectory learning and analysis for surveillance.
IEEE transactions on circuits and systems for video
technology, 18(8):1114–1127.
Pedregosa, F., Varoquaux, G., Gramfort, A., Michel, V.,
Thirion, B., Grisel, O., Blondel, M., Prettenhofer,
P., Weiss, R., Dubourg, V., Vanderplas, J., Passos,
A., Cournapeau, D., Brucher, M., Perrot, M., and
Duchesnay, E. (2011). Scikit-learn: Machine learning
in Python. Journal of Machine Learning Research,
12:2825–2830.
Platt, J. et al. (1999). Probabilistic outputs for support vec-
tor machines and comparisons to regularized likeli-
hood methods. Advances in large margin classifiers,
10(3):61–74.
Poppe, R. (2010). A survey on vision-based human action
recognition. Image and vision computing, 28(6):976–
990.
Ren, S., He, K., Girshick, R., and Sun, J. (2015). Faster
r-cnn: Towards real-time object detection with region
proposal networks. In Advances in neural information
processing systems, pages 91–99.
Shakhatreh, H., Sawalmeh, A. H., Al-Fuqaha, A., Dou, Z.,
Almaita, E., Khalil, I., Othman, N. S., Khreishah, A.,
and Guizani, M. (2019). Unmanned aerial vehicles
(uavs): A survey on civil applications and key re-
search challenges. IEEE Access, 7:48572–48634.
Shoufan, A., Al-Angari, H. M., Sheikh, M. F. A., and Dami-
ani, E. (2018). Drone pilot identification by classify-
ing radio-control signals. IEEE Transactions on Infor-
mation Forensics and Security, 13(10):2439–2447.
Soomro, K., Zamir, A. R., and Shah, M. (2012). Ucf101:
A dataset of 101 human actions classes from videos in
the wild. arXiv preprint arXiv:1212.0402.
Su, J., He, J., Cheng, P., and Chen, J. (2016). A stealthy
gps spoofing strategy for manipulating the trajectory
of an unmanned aerial vehicle. IFAC-PapersOnLine,
49(22):291–296.
Unlu, E., Zenou, E., and Riviere, N. (2018). Using shape
descriptors for uav detection. Electronic Imaging,
2018(9):1–5.
Unlu, E., Zenou, E., Riviere, N., and Dupouy, P.-E. (2019).
Deep learning-based strategies for the detection and
tracking of drones using several cameras. IPSJ
Transactions on Computer Vision and Applications,
11(1):7.
Wagoner, A. R., Schrader, D. K., and Matson, E. T. (2017).
Survey on detection and tracking of uavs using com-
puter vision. In 2017 First IEEE International Con-
ference on Robotic Computing (IRC), pages 320–325.
IEEE.
Zhang, H., Cao, C., Xu, L., and Gulliver, T. A. (2018). A
uav detection algorithm based on an artificial neural
network. IEEE Access, 6:24720–24728.
Zhang, T. and Zhu, Q. (2017). Strategic defense against de-
ceptive civilian gps spoofing of unmanned aerial ve-
hicles. In International Conference on Decision and
Game Theory for Security, pages 213–233. Springer.
A Preliminary Study on the Automatic Visual based Identification of UAV Pilots from Counter UAVs
589