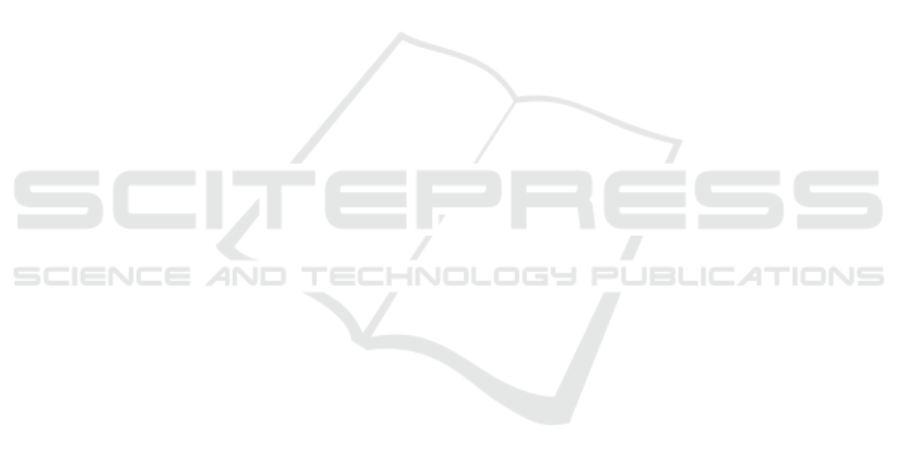
Ballinger, C. and Payne, S. (2002). The construction of the
risk of falling among and by older people. Ageing &
Society, 22(3):305–324.
Banerjee, T., Enayati, M., Keller, J. M., Skubic, M.,
Popescu, M., and Rantz, M. (2014). Monitoring pa-
tients in hospital beds using unobtrusive depth sen-
sors. In 2014 36th Annual International Conf. of the
IEEE Engineering in Medicine and Biology Society,
pages 5904–5907. IEEE.
Baptista-R
´
ıos, M., Mart
´
ınez-Garc
´
ıa, C., Losada-Guti
´
errez,
C., and Marr
´
on-Romera, M. (2016). Human activity
monitoring for falling detection. a realistic framework.
In 2016 International Conf. on Indoor Positioning and
Indoor Navigation (IPIN), pages 1–7.
Blamire, P. A. (1996). The influence of relative sample size
in training artificial neural networks. International
Journal of Remote Sensing, 17(1):223–230.
Chou, E., Tan, M., Zou, C., Guo, M., Haque, A., Milstein,
A., and Fei-Fei, L. (2018). Privacy-preserving action
recognition for smart hospitals using low-resolution
depth images. arXiv preprint arXiv:1811.09950.
De Miguel, K., Brunete, A., Hernando, M., and Gambao, E.
(2017). Home camera-based fall detection system for
the elderly. Sensors, 17(12).
dipakkr (2018). 3d-cnn for action recogni-
tion. https://github.com/dipakkr/3d-cnn-action-
recognition.
Gia, T. N., Sarker, V. K., Tcarenko, I., Rahmani, A. M.,
Westerlund, T., Liljeberg, P., and Tenhunen, H.
(2018). Energy efficient wearable sensor node for iot-
based fall detection systems. Microprocessors and Mi-
crosystems, 56:34–46.
Ji, S., Xu, W., Yang, M., and Yu, K. (2012). 3d convolu-
tional neural networks for human action recognition.
IEEE transactions on pattern analysis and machine
intelligence, 35(1):221–231.
Kingma, D. P. and Ba, J. (2014). Adam: A method for
stochastic optimization. CoRR, abs/1412.6980.
Lapierre, N., Neubauer, N., Miguel-Cruz, A., Rincon, A. R.,
Liu, L., and Rousseau, J. (2018). The state of knowl-
edge on technologies and their use for fall detection:
A scoping review. International journal of medical
informatics, 111:58–71.
Liang, B. and Zheng, L. (2015). A survey on human action
recognition using depth sensors. In 2015 International
Conf. on Digital Image Computing: Techniques and
Applications (DICTA), pages 1–8.
Lin, S., Liu, A., Hsu, T., and Fu, L. (2015). Representative
body points on top-view depth sequences for daily ac-
tivity recognition. In 2015 IEEE International Conf.
on Systems, Man, and Cybernetics, pages 2968–2973.
Luna, C. A., Losada-Gutierrez, C., Fuentes-Jimenez,
D., Fernandez-Rincon, A., Mazo, M., and Macias-
Guarasa, J. (2017). Robust people detection using
depth information from an overhead time-of-flight
camera. Expert Systems with Applications, 71:240–
256.
Macias-Guarasa, J., Losada-Gutierrez, C., and Fuentes-
Jimenez, D. (2018). GEINTRA Overhead ToF Peo-
ple Detection 3 (GOTPD3) database: Human activ-
ity detection. Available online: http:// www.geintra-
uah.org/datasets/gotpd3 (Last accessed: 09-Oct-2019.
Mathon, C., Beaucamp, F., Roca, F., Chassagne, P.,
Thevenon, A., and Puisieux, F. (2017). Post-fall syn-
drome: Profile and outcomes. Annals of Physical and
Rehabilitation Medicine, 60:e50–e51.
Megavannan, V., Agarwal, B., and Babu, R. V. (2012). Hu-
man action recognition using depth maps. In 2012
International Conf. on Signal Processing and Com-
munications (SPCOM), pages 1–5.
Mubashir, M., Shao, L., and Seed, L. (2013). A survey on
fall detection: Principles and approaches. Neurocom-
puting, 100:144–152.
Organization, W. H. (2012). Good health adds life to years.
global brief for world health day 2012. 2012.
Ozcan, K. and Velipasalar, S. (2016). Wearable camera-
and accelerometer-based fall detection on portable de-
vices. IEEE Embedded Systems Letters, 8(1):6–9.
Pierleoni, P., Belli, A., Palma, L., Pellegrini, M., Pernini, L.,
and Valenti, S. (2015). A high reliability wearable de-
vice for elderly fall detection. IEEE Sensors Journal,
15(8):4544–4553.
Rougier, C., Auvinet, E., Rousseau, J., Mignotte, M.,
and Meunier, J. (2011). Fall detection from depth
map video sequences. In International Conf. on
smart homes and health telematics, pages 121–128.
Springer.
Sundelin, T., Karshikoff, B., Axelsson, E., H
¨
oglund, C. O.,
Lekander, M., and Axelsson, J. (2015). Sick man
walking: Perception of health status from body mo-
tion. Brain, Behavior, and Immunity, 48:53 – 56.
Tang-Wei Hsu, Yu-Huan Yang, Tso-Hsin Yeh, An-Sheng
Liu, Li-Chen Fu, and Yi-Chong Zeng (2016). Pri-
vacy free indoor action detection system using top-
view depth camera based on key-poses. In 2016 IEEE
International Conf. on Systems, Man, and Cybernetics
(SMC), pages 004058–004063.
Wang, P., Li, W., Gao, Z., Tang, C., and Ogunbona, P. O.
(2018). Depth pooling based large-scale 3-d action
recognition with convolutional neural networks. IEEE
Transactions on Multimedia, 20(5):1051–1061.
Wang, P., Wang, S., Gao, Z., Hou, Y., and Li, W. (2017).
Structured images for rgb-d action recognition. In
Proceedings of the IEEE International Conf. on Com-
puter Vision, pages 1005–1014.
Wu, F., Zhao, H., Zhao, Y., and Zhong, H. (2015). De-
velopment of a wearable-sensor-based fall detection
system. Int. J. Telemedicine Appl., 2015:2:2–2:2.
Yang, X., Zhang, C., and Tian, Y. (2012). Recognizing ac-
tions using depth motion maps-based histograms of
oriented gradients. In Proceedings of the 20th ACM
international Conf. on Multimedia, pages 1057–1060.
ACM.
Zerrouki, N., Harrou, F., Sun, Y., and Houacine, A.
(2016). Accelerometer and camera-based strategy for
improved human fall detection. Journal of Medical
Systems, 40(12):284.
3D Convolutional Neural Network for Falling Detection using Only Depth Information
597