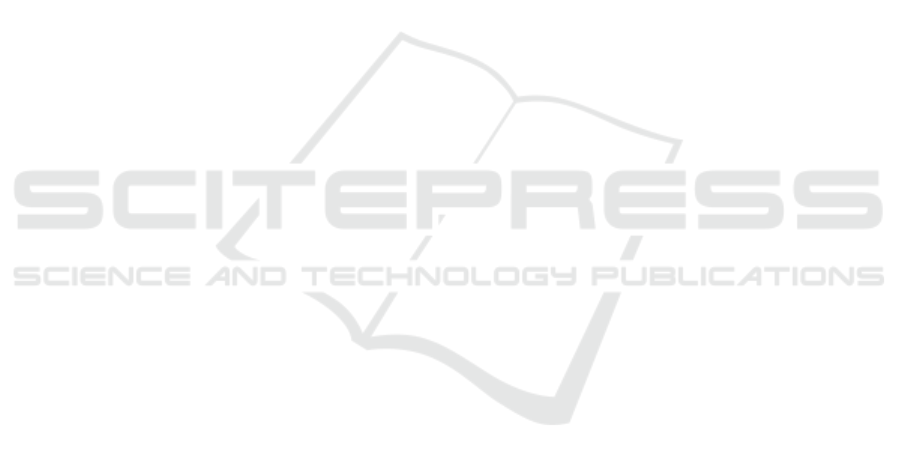
users our system outperformed the iView SMI RED
250 eye tracker.
We also evaluated the results for the users wearing
glasses and without glasses as shown in Figure 11 and
Figure 12. It is evident that both systems work better
with the user without glasses compared to users with
glasses. Table 3 details the accuracy for the compared
systems for users with and without glasses.
6 CONCLUSION
We worked on the eye gaze location detection on the
screen for team meetings to help BVIP to get im-
mersed in the conversation. We built a prototype of
an automatic eye gaze tracking system which can be
available at low cost using an open source software
’OpenFace’. We geometrically converted the eye gaze
vectors and eye position coordinates to screen coor-
dinates and manipulated those coordinates using an
SVM regression algorithm to work in a similar man-
ner to the commercially available SMI 250 RED eye
tracker. We used a small desktop screen with 2 × 3
box matrix to calibrate our proposed system for eye
gaze tracking.
In our user study, we evaluated our automatic sys-
tem with 28 users. We found out that our system
works quite comparable to the SMI RED 250 eye
tracker for the numbers which are inside the box ma-
trix on the screen but inversely for the corner boxes
on the screen which led to the accuracy difference be-
tween the proposed system and the SMI RED 250.
We compared the performance of users with spec-
tacles and without spectacles which showed that the
users with spectacles had less accuracy than without
spectacles which might be due to the extra reflection
due to presence glasses.
In future work, we will convert the output in such
a way that it can be made accessible to BVIP by au-
dio or haptic feedback. We will also work on improv-
ing the accuracy of our system using neural networks
which have proven to perform better than the classical
computer vision techniques in other problems.
ACKNOWLEDGEMENTS
This work has been supported by the Swiss Na-
tional Science Foundation (SNF) under the grant no.
200021E 177542 / 1. It is part of a joint project be-
tween TU Darmstadt, ETH Zurich, and JKU Linz
with the respective funding organizations DFG (Ger-
man Research Foundation), SNF and FWF (Austrian
Science Fund). We also thank Dr. Quentin Lohmeyer
and Product Development Group Zurich for lending
us the SMI RED 250 eye tracker.
REFERENCES
Agarwal, A., JeevithaShree, D., Saluja, K. S., Sahay, A.,
Mounika, P., Sahu, A., Bhaumik, R., Rajendran, V. K.,
and Biswas, P. (2019a). Comparing two webcam-
based eye gaze trackers for users with severe speech
and motor impairment. In Research into Design for a
Connected World, pages 641–652. Springer.
Agarwal, A., JeevithaShree, D., Saluja, K. S., Sahay, A.,
Mounika, P., Sahu, A., Bhaumik, R., Rajendran, V. K.,
and Biswas, P. (2019b). Comparing two webcam-
based eye gaze trackers for users with severe speech
and motor impairment. In Chakrabarti, A., editor, Re-
search into Design for a Connected World, pages 641–
652, Singapore. Springer Singapore.
Amos, B., Ludwiczuk, B., Satyanarayanan, M., et al.
(2016). Openface: A general-purpose face recogni-
tion library with mobile applications. CMU School of
Computer Science, 6.
Bal, E., Harden, E., Lamb, D., Van Hecke, A. V., Denver,
J. W., and Porges, S. W. (2010). Emotion recognition
in children with autism spectrum disorders: Relations
to eye gaze and autonomic state. Journal of autism
and developmental disorders, 40(3):358–370.
Baltru
ˇ
saitis, T., Robinson, P., and Morency, L.-P. (2016).
Openface: an open source facial behavior analysis
toolkit. In 2016 IEEE Winter Conference on Applica-
tions of Computer Vision (WACV), pages 1–10. IEEE.
Baltru
ˇ
saitis, T., Zadeh, A., Lim, Y. C., and Morency, L.-
P. (2018). Openface 2.0: Facial behavior analysis
toolkit. In 2018 13th IEEE International Conference
on Automatic Face & Gesture Recognition (FG 2018),
pages 59–66. IEEE.
Dhingra, N. and Kunz, A. (2019). Res3atn-deep 3D resid-
ual attention network for hand gesture recognition in
videos. In 2019 International Conference on 3D Vi-
sion (3DV), pages 491–501. IEEE.
Dhingra, N., Valli, E., and Kunz, A. (2020). Recognition
and localisation of pointing gestures using a RGB-D
camera. arXiv e-prints, page arXiv:2001.03687.
Dong, X., Wang, H., Chen, Z., and Shi, B. E. (2015).
Hybrid brain computer interface via bayesian inte-
gration of eeg and eye gaze. In 2015 7th Interna-
tional IEEE/EMBS Conference on Neural Engineer-
ing (NER), pages 150–153. IEEE.
Dostal, J., Kristensson, P. O., and Quigley, A. (2013). Sub-
tle gaze-dependent techniques for visualising display
changes in multi-display environments. In Proceed-
ings of the 2013 international conference on Intelli-
gent user interfaces, pages 137–148. ACM.
Drewes, H. and Schmidt, A. (2007). Interacting with
the computer using gaze gestures. In IFIP Confer-
ence on Human-Computer Interaction, pages 475–
488. Springer.
Duchowski, A. T. (2007). Eye tracking methodology. The-
ory and practice, 328(614):2–3.
Eivazi, S., K
¨
ubler, T. C., Santini, T., and Kasneci, E.
(2018). An inconspicuous and modular head-mounted
Eye Gaze Tracking for Detecting Non-verbal Communication in Meeting Environments
245