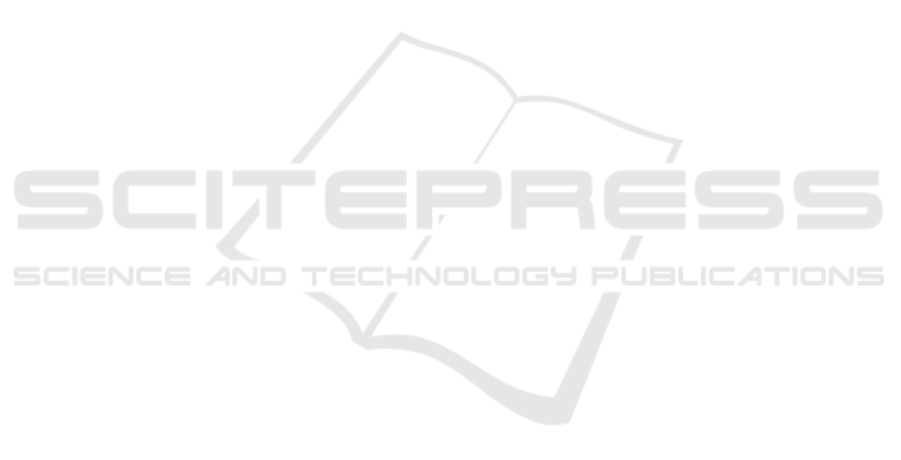
REFERENCES
Arik, S., Ibragimov, B., and Xing, L. (2017). Fully
automated quantitative cephalometry using convolu-
tional neural networks. Journal of Medical Imaging,
4:014501.
Bier, B., Unberath, M., Zaech, J.-N., Fotouhi, J., Armand,
M., Osgood, G., Navab, N., and Maier, A. (2018).
X-ray-transform invariant anatomical landmark de-
tection for pelvic trauma surgery. In Frangi, A. F.,
Schnabel, J. A., Davatzikos, C., Alberola-López, C.,
and Fichtinger, G., editors, Medical Image Computing
and Computer Assisted Intervention – MICCAI 2018,
pages 55–63, Cham. Springer International Publish-
ing.
Cardillo, J. and Sid-Ahmed, M. A. (1994). An image pro-
cessing system for locating craniofacial landmarks.
IEEE Transactions on Medical Imaging, 13(2):275–
289.
Cootes, T., Edwards, G., and Taylor, C. (2006). Active ap-
pearance models, volume 23, pages 484–498.
Cootes, T., Taylor, C., Cooper, D., and Graham, J.
(1995). Active shape models-their training and appli-
cation. Computer Vision and Image Understanding,
61:38–59.
Davis, D. N. and Taylor, C. (1991). A blackboard architec-
ture for automating cephalometric analysis. Medical
informatics = Médecine et informatique, 16:137–49.
Eaton-Rosen, Z., Bragman, F., Bisdas, S., Ourselin, S., and
Cardoso, M. J. (2018). Towards Safe Deep Learn-
ing: Accurately Quantifying Biomarker Uncertainty
in Neural Network Predictions: 21st International
Conference, Granada, Spain, September 16–20, 2018,
Proceedings, Part I, pages 691–699.
Gal, Y. (2016). Uncertainty in Deep Learning. PhD thesis,
University of Cambridge.
Gal, Y. and Ghahramani, Z. (2016). Dropout as a bayesian
approximation: Representing model uncertainty in
deep learning. In Proceedings of the 33rd Inter-
national Conference on International Conference on
Machine Learning - Volume 48, ICML’16, pages
1050–1059. JMLR.org.
Grau, V., Alcañiz Raya, M., Juan, M.-C., Monserrat, C., and
Knoll, C. (2001). Automatic localization of cephalo-
metric landmarks. Journal of biomedical informatics,
34:146–56.
Guha Roy, A., Conjeti, S., Navab, N., and Wachinger, C.
(2018). Inherent Brain Segmentation Quality Con-
trol from Fully ConvNet Monte Carlo Sampling, pages
664–672.
Hinton, G. E., Srivastava, N., Krizhevsky, A., Sutskever,
I., and Salakhutdinov, R. (2012). Improving neural
networks by preventing co-adaptation of feature de-
tectors. CoRR, abs/1207.0580.
Lakshminarayanan, B., Pritzel, A., and Blundell, C. (2017).
Simple and scalable predictive uncertainty estimation
using deep ensembles. In Guyon, I., Luxburg, U. V.,
Bengio, S., Wallach, H., Fergus, R., Vishwanathan, S.,
and Garnett, R., editors, Advances in Neural Informa-
tion Processing Systems 30, pages 6402–6413. Curran
Associates, Inc.
Leibig, C., Allken, V., Ayhan, M. S., Berens, P., and Wahl,
S. (2017). Leveraging uncertainty information from
deep neural networks for disease detection. Scientific
Reports, 7.
Levy-Mandel, A. D., Tsotsos, J. K., and Venetsanopou-
los, A. N. (1985). Knowledge-based landmarking of
cephalograms. In Lemke, H., Rhodes, M. L., Jaffee,
C. C., and Felix, R., editors, Computer Assisted Ra-
diology / Computergestützte Radiologie, pages 473–
478, Berlin, Heidelberg. Springer Berlin Heidelberg.
Litjens, G. J. S., Kooi, T., Bejnordi, B. E., Setio, A. A. A.,
Ciompi, F., Ghafoorian, M., van der Laak, J., van Gin-
neken, B., and Sánchez, C. I. (2017). A survey on
deep learning in medical image analysis. Medical im-
age analysis, 42:60–88.
Paszke, A., Gross, S., Chintala, S., Chanan, G., Yang, E.,
DeVito, Z., Lin, Z., Desmaison, A., Antiga, L., and
Lerer, A. (2017). Automatic differentiation in pytorch.
In NIPS-W.
Payer, C., Stern, D., Bischof, H., and Urschler, M. (2016).
Regressing Heatmaps for Multiple Landmark Local-
ization Using CNNs, volume 9901 of Lecture Notes in
Computer Science, pages 230–238. Springer Interna-
tional Publishing AG, Switzerland.
Pei, Y., Liu, B., Zha, H., Han, B., and Xu, T. (2006).
Anatomical structure sketcher for cephalograms by bi-
modal deep learning. Trans. Biomed. Eng., 53:1615–
1623.
Ronneberger, O., Fischer, P., and Brox, T. (2015). U-
net: Convolutional networks for biomedical image
segmentation. In Navab, N., Hornegger, J., Wells,
W. M., and Frangi, A. F., editors, Medical Image Com-
puting and Computer-Assisted Intervention – MICCAI
2015, pages 234–241, Cham. Springer International
Publishing.
Wang, C.-W., Huang, C.-T., Lee, J.-H., Li, C.-H., Chang,
S.-W., Siao, M.-J., Lai, T.-M., Ibragimov, B., Vrtovec,
T., Ronneberger, O., Fischer, P., Cootes, T. F., and
Lindner, C. (2016). A benchmark for comparison of
dental radiography analysis algorithms. Medical Im-
age Analysis, 31:63 – 76.
Widdowson, S. and Taylor, D. (2016). The management of
grading quality: good practice in the quality assurance
of grading. Tech Report.
BIOIMAGING 2020 - 7th International Conference on Bioimaging
220