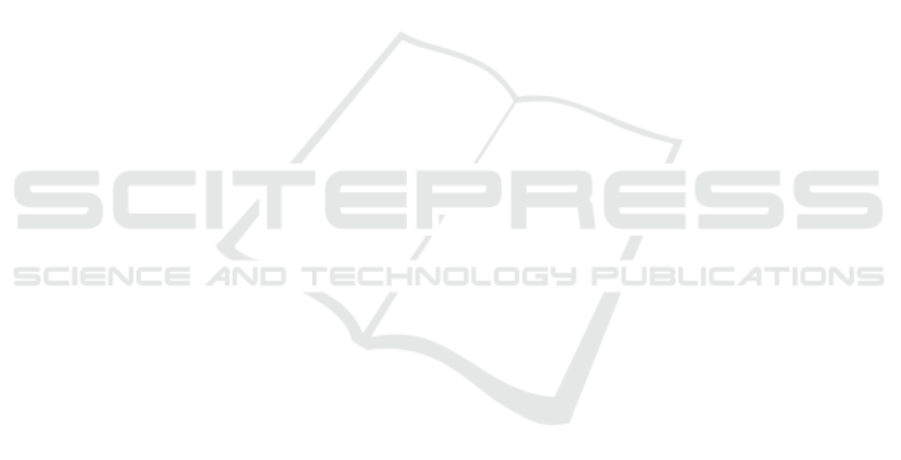
REFERENCES
Aramaki, E., Maskawa, S., and Morita, M. (2011). Twitter
Catches The Flu: Detecting Influenza Epidemics us-
ing Twitter. In Proceedings of the 2011 Conference on
Empirical Methods in Natural Language Processing,
pages 1568–1576, Edinburgh, Scotland, UK. Associ-
ation for Computational Linguistics.
Blei, D. M., Ng, A. Y., and Jordan, M. I. (2003). Latent
Dirichlet Allocation. Journal of Machine Learning
Research, 3(Jan):993–1022.
Brownstein, J. S., Freifeld, C. C., Chan, E. H., Keller, M.,
Sonricker, A. L., Mekaru, S. R., and Buckeridge, D. L.
(2010). Information Technology and Global Surveil-
lance of Cases of 2009 H1n1 Influenza. New England
Journal of Medicine, 362(18):1731–1735.
Collier, N. and Doan, S. (2012). Syndromic Classification
of Twitter Messages. In Kostkova, P., Szomszor, M.,
and Fowler, D., editors, Electronic Healthcare, vol-
ume 91, pages 186–195. Springer Berlin Heidelberg,
Berlin, Heidelberg.
Cooper, G. F., Villamarin, R., Tsui, F.-C. R., Millett, N.,
Espino, J. U., and Wagner, M. M. (2015). A Method
for Detecting and Characterizing Outbreaks of Infec-
tious Disease from Clinical Reports. J Biomed Inform,
53:15–26.
Ji, X., Chun, S. A., and Geller, J. (2013). Monitoring public
health concerns using twitter sentiment classifications.
In 2013 IEEE International Conference on Healthcare
Informatics, pages 335–344.
Ji, X., Chun, S. A., and Geller, J. (2013). Monitoring Pub-
lic Health Concerns Using Twitter Sentiment Classi-
fications. In 2013 IEEE International Conference on
Healthcare Informatics, pages 335–344, Philadelphia,
PA, USA. IEEE.
Keshtkar, F. and Inkpen, D. (2009). Using sentiment ori-
entation features for mood classification in blogs. In
2009 International Conference on Natural Language
Processing and Knowledge Engineering, pages 1–6.
Lamb, A., Paul, M. J., and Dredze, M. (2015). Separating
Fact from Fear: Tracking Flu Infections on Twitter.
page 7.
Lampos, V. and Cristianini, N. (2010). Tracking the flu pan-
demic by monitoring the social web. In 2010 2nd In-
ternational Workshop on Cognitive Information Pro-
cessing, pages 411–416, Elba Island, Italy. IEEE.
Long-Hay, P., Yamamoto, E., Bun, S., Savuth, T., Buntha,
S., Sokdaro, S., Kariya, T., Saw, Y. M., Sengdoeurn,
Y., and Hamajima, N. (2019). Outbreak detection of
influenza-like illness in Prey Veng Province, Cambo-
dia: a community-based surveillance. Nagoya J Med
Sci, 81(2):269–280.
Mikolov, T., Yih, W.-t., and Zweig, G. (2014). Linguistic
Regularities in Continuous Space Word Representa-
tions. page 6.
Paul, M. J., Dredze, M., and University, J. H. (2017). You
Are What You Tweet: Analyzing Twitter for Public
Health. page 8.
Saeed, Z., Abbasi, R. A., Maqbool, O., Sadaf, A., Raz-
zak, I., Daud, A., Aljohani, N. R., and Xu, G. (2019).
What’s Happening Around the World? A Survey and
Framework on Event Detection Techniques on Twit-
ter. J Grid Computing, 17(2):279–312.
Shah, M. (2017). Disease Propagation in Social Networks:
A Novel Study of Infection Genesis and Spread on
Twitter. page 17.
Wang, C.-K., Singh, O., Tang, Z.-L., and Dai, H.-J. (2017).
Using a Recurrent Neural Network Model for Classi-
fication of Tweets Conveyed Influenza-related Infor-
mation. In Proceedings of the International Workshop
on Digital Disease Detection using Social Media 2017
(DDDSM-2017), pages 33–38, Taipei, Taiwan. Asso-
ciation for Computational Linguistics.
Using BERT and Semantic Patterns to Analyze Disease Outbreak Context over Social Network Data
863