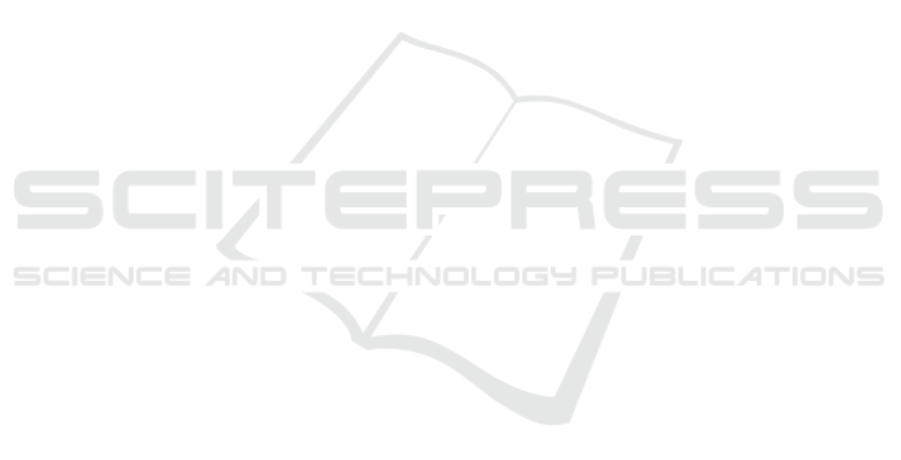
via multi-stage fully convolutional networks. IEEE
Transactions on Biomedical Engineering.
Codella, N. C., Gutman, D., Celebi, M. E., Helba, B.,
Marchetti, M. A., Dusza, S. W., Kalloo, A., Li-
opyris, K., Mishra, N., Kittler, H., et al. (2017).
Skin lesion analysis toward melanoma detection: A
challenge at the 2017 international symposium on
biomedical imaging (isbi), hosted by the international
skin imaging collaboration (isic). arXiv preprint
arXiv:1710.05006.
Dvo
ˇ
r
´
ankov
´
a, B., Szabo, P., Kodet, O., Strnad, H., Kol
´
a
ˇ
r,
M., Lacina, L., Krej
ˇ
c
´
ı, E., Na
ˇ
nka, O.,
ˇ
Sedo, A., and
Smetana, K. (2017). Intercellular crosstalk in human
malignant melanoma. Protoplasma, pages 1–8.
Everingham, M., Eslami, S. M. A., Van Gool, L., Williams,
C. K. I., Winn, J., and Zisserman, A. (2015). The pas-
cal visual object classes challenge: A retrospective.
International Journal of Computer Vision, 111(1):98–
136.
Garcia-Garcia, A., Orts-Escolano, S., Oprea, S., Villena-
Martinez, V., and Garcia-Rodriguez, J. (2017). A re-
view on deep learning techniques applied to semantic
segmentation. arXiv preprint arXiv:1704.06857.
Goyal, M., Oakley, A., Bansal, P., Dancey, D., and Yap,
M. H. (2019). Skin lesion segmentation in dermo-
scopic images with ensemble deep learning methods.
IEEE Access, pages 1–1.
Goyal, M., Reeves, N. D., Rajbhandari, S., Spragg, J., and
Yap, M. H. (2017). Fully convolutional networks
for diabetic foot ulcer segmentation. arXiv preprint
arXiv:1708.01928.
Jia, Y., Shelhamer, E., Donahue, J., Karayev, S., Long, J.,
Girshick, R., Guadarrama, S., and Darrell, T. (2014).
Caffe: Convolutional architecture for fast feature em-
bedding. In Proceedings of the 22nd ACM inter-
national conference on Multimedia, pages 675–678.
ACM.
Krizhevsky, A., Sutskever, I., and Hinton, G. E. (2012). Im-
agenet classification with deep convolutional neural
networks. In Advances in neural information process-
ing systems, pages 1097–1105.
LeCun, Y., Bengio, Y., and Hinton, G. (2015). Deep learn-
ing. Nature, 521(7553):436–444.
Long, J., Shelhamer, E., and Darrell, T. (2015). Fully con-
volutional networks for semantic segmentation. In
Proceedings of the IEEE Conference on Computer Vi-
sion and Pattern Recognition, pages 3431–3440.
Melanoma Foundation (AIM) (2017). Melanoma stats,
facts and figures. last access: 27/10/2017.
National Cancer Institute (2017). Cancer stat facts:
Melanoma of the skin. last access: 26/10/17.
Pathan, S., Prabhu, K. G., and Siddalingaswamy, P. (2018).
Techniques and algorithms for computer aided diag-
nosis of pigmented skin lesions—a review. Biomedi-
cal Signal Processing and Control, 39:237–262.
Russakovsky, O., Deng, J., Su, H., Krause, J., Satheesh,
S., Ma, S., Huang, Z., Karpathy, A., Khosla,
A., Bernstein, M., et al. (2014). Imagenet large
scale visual recognition challenge. arXiv preprint
arXiv:1409.0575.
Simonyan, K. and Zisserman, A. (2014). Very deep con-
volutional networks for large-scale image recognition.
arXiv preprint arXiv:1409.1556.
Yu, L., Chen, H., Dou, Q., Qin, J., and Heng, P.-A. (2017).
Automated melanoma recognition in dermoscopy im-
ages via very deep residual networks. IEEE transac-
tions on medical imaging, 36(4):994–1004.
Yuan, Y., Chao, M., and Lo, Y.-C. (2017). Automatic skin
lesion segmentation using deep fully convolutional
networks with jaccard distance. IEEE Transactions
on Medical Imaging.
Multi-class Semantic Segmentation of Skin Lesions via Fully Convolutional Networks
295