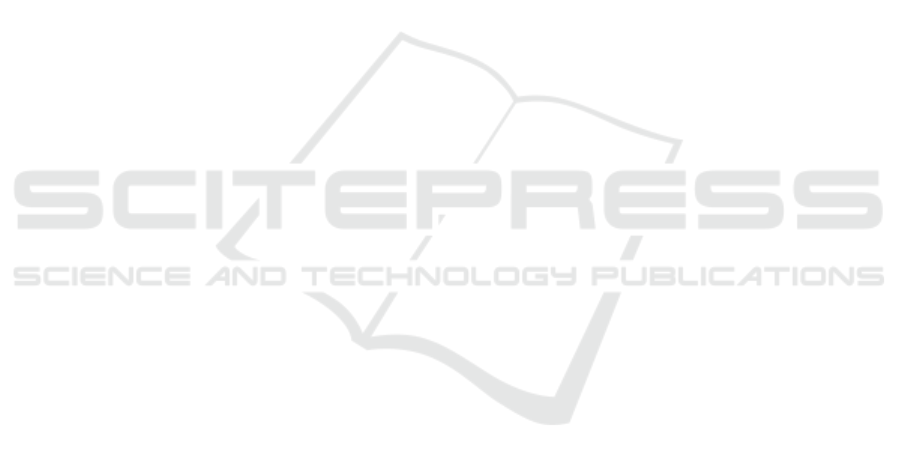
Anderson, J. P. (1980). Computer security threat monitoring
and surveillance.
Campbell, T. (2016). Protection of systems. In Prac-
tical Information Security Management, pages 155–
177. Springer.
Carta, S., Fenu, G., Recupero, D. R., and Saia, R. (2019).
Fraud detection for e-commerce transactions by em-
ploying a prudential multiple consensus model. J. Inf.
Secur. Appl., 46:13–22.
Castiello, C., Castellano, G., and Fanelli, A. M. (2005).
Meta-data: Characterization of input features for
meta-learning. In International Conference on Mod-
eling Decisions for Artificial Intelligence, pages 457–
468. Springer.
Chopra, A. and Bhilare, P. (2018). Application of ensemble
models in credit scoring models. Business Perspec-
tives and Research, 6(2):129–141.
Damrongsakmethee, T. and Neagoe, V.-E. (2019). Principal
component analysis and relieff cascaded with decision
tree for credit scoring. In Computer Science On-line
Conference, pages 85–95. Springer.
Denning, D. E. (1987). An intrusion-detection model. IEEE
Transactions on software engineering, (2):222–232.
Falc
˜
ao, F., Zoppi, T., Silva, C. B. V., Santos, A., Fonseca,
B., Ceccarelli, A., and Bondavalli, A. (2019). Quanti-
tative comparison of unsupervised anomaly detection
algorithms for intrusion detection. In Proceedings of
the 34th ACM/SIGAPP Symposium on Applied Com-
puting, pages 318–327.
Fawcett, T. (2004). Roc graphs: Notes and practical consid-
erations for researchers. Machine learning, 31(1):1–
38.
Gilmore, C. and Haydaman, J. (2016). Anomaly detection
and machine learning methods for network intrusion
detection: An industrially focused literature review. In
Proceedings of the International Conference on Secu-
rity and Management (SAM), page 292. The Steering
Committee of The World Congress in Computer Sci-
ence, Computer . . . .
Jose, S., Malathi, D., Reddy, B., and Jayaseeli, D. (2018). A
survey on anomaly based host intrusion detection sys-
tem. In Journal of Physics: Conference Series, vol-
ume 1000, page 012049. IOP Publishing.
Kumar, G. (2014). Evaluation metrics for intrusion detec-
tion systems-a study. Evaluation, 2(11):11–7.
Liao, H.-J., Lin, C.-H. R., Lin, Y.-C., and Tung, K.-Y.
(2013). Intrusion detection system: A comprehensive
review. Journal of Network and Computer Applica-
tions, 36(1):16–24.
Liu, H., Hussain, F., Tan, C. L., and Dash, M. (2002). Dis-
cretization: An enabling technique. Data mining and
knowledge discovery, 6(4):393–423.
Luo, C., Wu, D., and Wu, D. (2017). A deep learn-
ing approach for credit scoring using credit default
swaps. Engineering Applications of Artificial Intel-
ligence, 65:465–470.
Luque, A., Carrasco, A., Mart
´
ın, A., and de las Heras, A.
(2019). The impact of class imbalance in classifica-
tion performance metrics based on the binary confu-
sion matrix. Pattern Recognition, 91:216–231.
Malekipirbazari, M. and Aksakalli, V. (2015). Risk assess-
ment in social lending via random forests. Expert Sys-
tems with Applications, 42(10):4621–4631.
Mazini, M., Shirazi, B., and Mahdavi, I. (2019). Anomaly
network-based intrusion detection system using a re-
liable hybrid artificial bee colony and adaboost algo-
rithms. Journal of King Saud University-Computer
and Information Sciences, 31(4):541–553.
McHugh, J., Christie, A., and Allen, J. (2000). Defend-
ing yourself: The role of intrusion detection systems.
IEEE software, 17(5):42–51.
Munaiah, N., Meneely, A., Wilson, R., and Short, B. (2016).
Are intrusion detection studies evaluated consistently?
a systematic literature review.
Pfleeger, C. P. and Pfleeger, S. L. (2012). Security in Com-
puting, 4th Edition. Prentice Hall.
Potluri, S. and Diedrich, C. (2016). High performance in-
trusion detection and prevention systems: A survey. In
ECCWS2016-Proceedings fo the 15th European Con-
ference on Cyber Warfare and Security, page 260.
Academic Conferences and publishing limited.
Rodda, S. and Erothi, U. S. R. (2016). Class imbal-
ance problem in the network intrusion detection sys-
tems. In 2016 International Conference on Electrical,
Electronics, and Optimization Techniques (ICEEOT),
pages 2685–2688. IEEE.
Saia, R. and Carta, S. (2016). A linear-dependence-based
approach to design proactive credit scoring models. In
KDIR, pages 111–120. SciTePress.
Saia, R. and Carta, S. (2017). Evaluating credit card trans-
actions in the frequency domain for a proactive fraud
detection approach. In SECRYPT, pages 335–342.
SciTePress.
Saia, R., Carta, S., and Fenu, G. (2018). A wavelet-based
data analysis to credit scoring. In ICDSP, pages 176–
180. ACM.
Saia, R., Carta, S., Recupero, D. R., Fenu, G., and Saia, M.
(2019a). A discretized enriched technique to enhance
machine learning performance in credit scoring. In
KDIR, pages 202–213. ScitePress.
Saia, R., Carta, S., Recupero, D. R., Fenu, G., and Stan-
ciu, M. (2019b). A discretized extended feature space
(DEFS) model to improve the anomaly detection per-
formance in network intrusion detection systems. In
KDIR, pages 322–329. ScitePress.
Sundaram, A. (1996). An introduction to intrusion detec-
tion. Crossroads, 2(4):3–7.
Wang, W., Guyet, T., Quiniou, R., Cordier, M.-O.,
Masseglia, F., and Zhang, X. (2014a). Autonomic
intrusion detection: Adaptively detecting anomalies
over unlabeled audit data streams in computer net-
works. Knowledge-Based Systems, 70:103–117.
Wang, Y., Yang, K., Jing, X., and Jin, H. L. (2014b). Prob-
lems of kdd cup 99 dataset existed and data prepro-
cessing. In Applied Mechanics and Materials, volume
667, pages 218–225. Trans Tech Publ.
Xia, Y., Liu, C., Li, Y., and Liu, N. (2017). A boosted de-
cision tree approach using bayesian hyper-parameter
optimization for credit scoring. Expert Systems with
Applications, 78:225–241.
KDIR 2020 - 12th International Conference on Knowledge Discovery and Information Retrieval
144