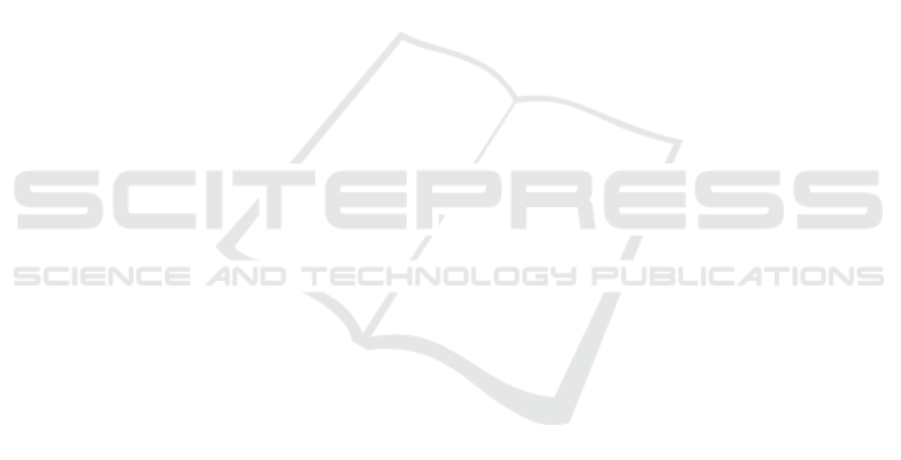
ness, consistency and locality), but also provides in-
formation that can be used to decide about the actions
of an agent.
The advantage of reflexive reinforcement learn-
ing is that an agent can learn even in the ab-
sence of an evaluative signal (reward and punish-
ment), it can bootstrap elementary actions (as in
homeokinesis (Der and Martius, 2012)) or can learn
about options in the environment (as in empower-
ment (Klyubin et al., 2005b)), and obtain more mean-
ingful and generalisable representations (see (Smith
and Herrmann, 2019)).
The unavoidable difficulty in reflexive reinforce-
ment learning consists in the fact that the use of quan-
tities that are eventually based on the reward as a re-
ward, introduces a feedback loop which can lead to
instabilities or divergences. This is not unknown in
RL, where e.g., an often visited source of low re-
ward can dominate a better source of reward that is
rarely found, or in cases where correlations among
basis functions lead to divergences as notice already
in Ref. (Baird, 1995).
In RRL such feedback is even more typical, but
can also be used to introduce structure the state space
by self-organised pattern formation or to identify hi-
erarchical relationships as will be studied in future.
In order to keep the effects of self-referentiality under
control and to make use of their potential a dynamical
systems theory of reinforcement learning is required
that does not only consider the agent as a dynamical
system, but the full interactive system formed by the
agent, its environment and its internal representations.
ACKNOWLEDGEMENTS
This research was funded by EPSRC through the CDT
RAS at Edinburgh Centre for Robotics. Discussions
with Calum Imrie and Simon Smith are gratefully ac-
knowledged.
REFERENCES
Baird, L. (1995). Residual algorithms: Reinforcement
learning with function approximation. In Machine
Learning Proceedings 1995, pages 30–37. Elsevier.
Der, R. and Martius, G. (2012). The playful machine: The-
oretical foundation and practical realization of self-
organizing robots, volume 15. Springer Science &
Business Media.
Friston, K., Kilner, J., and Harrison, L. (2006). A free en-
ergy principle for the brain. Journal of Physiology-
Paris, 100(1-3):70–87.
Friston, K. J., Daunizeau, J., and Kiebel, S. J. (2009). Re-
inforcement learning or active inference? PloS one,
4(7):e6421.
Herrmann, M. and Der, R. (1995). Efficient q-learning by
division of labour. In Proceedings ICANN, volume 95,
pages 129–134.
Klyubin, A. S., Polani, D., and Nehaniv, C. L. (2005a). All
else being equal be empowered. In European Confer-
ence on Artificial Life, pages 744–753. Springer.
Klyubin, A. S., Polani, D., and Nehaniv, C. L. (2005b).
Empowerment: A universal agent-centric measure of
control. In 2005 IEEE Congress on Evolutionary
Computation, volume 1, pages 128–135. IEEE.
Laureiro-Mart
´
ınez, D., Brusoni, S., and Zollo, M. (2010).
The neuroscientific foundations of the exploration-
exploitation dilemma. Journal of Neuroscience, Psy-
chology, and Economics, 3(2):95.
March, J. G. (1991). Exploration and exploitation in organi-
zational learning. Organization science, 2(1):71–87.
Ng, A. Y. and Russell, S. J. (2000). Algorithms for inverse
reinforcement learning. In IMCL, pages 663–670.
Pathak, D., Agrawal, P., Efros, A. A., and Darrell, T. (2017).
Curiosity-driven exploration by self-supervised pre-
diction. In Proceedings of the IEEE Conference on
Computer Vision and Pattern Recognition Workshops,
pages 16–17.
Prange, C. and Schlegelmilch, B. B. (2009). The role of am-
bidexterity in marketing strategy implementation: Re-
solving the exploration-exploitation dilemma. Busi-
ness Research, 2(2):215–240.
Salge, C., Glackin, C., and Polani, D. (2014). Empower-
ment – an introduction. In Guided Self-Organization:
Inception, pages 67–114. Springer.
Salge, C. and Polani, D. (2017). Empowerment as re-
placement for the three laws of robotics. Frontiers in
Robotics and AI, 4:25.
Smith, S. C., Dharmadi, R., Imrie, C., Si, B., and Herrmann,
J. M. (2020). The DIAMOnD model: Deep recur-
rent neural networks for self-organising robot control.
Frontiers in Neurorobotics, 14:62.
Smith, S. C. and Herrmann, J. M. (2019). Evaluation
of internal models in autonomous learning. IEEE
Transactions on Cognitive and Developmental Sys-
tems, 11(4):463–472.
Sutton, R. S. and Barto, A. G. (1999). Reinforcement learn-
ing. Journal of Cognitive Neuroscience, 11(1):126–
134.
Sutton, R. S. and Barto, A. G. (2018). Reinforcement learn-
ing: An Introduction. MIT Press.
Thrun, S. (2002). Probabilistic robotics. Communications
of the ACM, 45(3):52–57.
Tschantz, A., Millidge, B., Seth, A. K., and Buckley, C. L.
(2020). Reinforcement learning through active infer-
ence. arXiv preprint arXiv:2002.12636.
Walter, E. (2008). Cambridge advanced learner’s dictio-
nary. Cambridge University Press.
Zhao, R., Tiomkin, S., and Abbeel, P. (2019). Learning
efficient representation for intrinsic motivation. arXiv
preprint arXiv:1912.02624.
NCTA 2020 - 12th International Conference on Neural Computation Theory and Applications
388