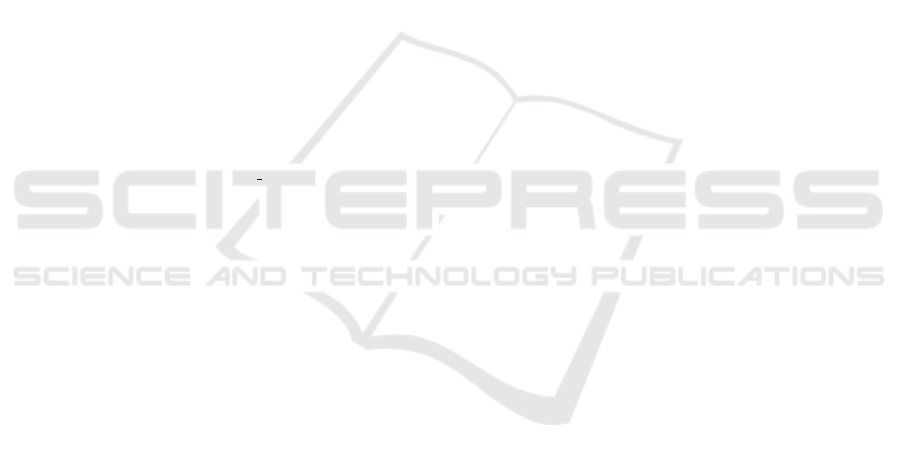
During our data analysis we have also identified
the need for differentiation between vague and am-
biguous terms, which we believe has implications for
the use of test corpora – such as MeSH – for WSD
research, even beyond the medical domain.
REFERENCES
Agirre, E. and Soroa, A. (2009). Personalizing pagerank
for word sense disambiguation. In Proceedings of the
EACL 2009, pages 33–41.
Antunes, R. and Matos, S. (2017a). Biomedical word sense
disambiguation with word embeddings. In Interna-
tional Conference on Practical Applications of Com-
putational Biology & Bioinformatics, pages 273–279.
Springer.
Antunes, R. and Matos, S. (2017b). Supervised learning
and knowledge-based approaches applied to biomedi-
cal word sense disambiguation. Journal of integrative
bioinformatics, 14(4).
Biber, D. (2006). University language: A corpus-based
study of spoken and written discourse. Amsterdam:
John Benjamin.
Bodenreider, O. (2004). The unified medical language sys-
tem (umls): integrating biomedical terminology. Nu-
cleic acids research, 32(suppl 1):D267–D270.
Geeraerts, D. (2010). Theories of lexical semantics. Oxford
University Press.
Gui, L., Leng, J., Pergola, G., Xu, R., He, Y., et al. (2019).
Neural topic model with reinforcement learning. In
Proceedings of the 2019 Conference on EMNLP-
IJCNLP, pages 3469–3474.
Humphrey, S. M., Rogers, W. J., Kilicoglu, H., Demner-
Fushman, D., and Rindflesch, T. C. (2006). Word
sense disambiguation by selecting the best semantic
type based on journal descriptor indexing: Prelimi-
nary experiment. JASIST, 57(1):96–113.
Jiang, J. J. and Conrath, D. W. (1997). Semantic similarity
based on corpus statistics and lexical taxonomy. arXiv
preprint cmp-lg/9709008.
Jimeno-Yepes, A. J. and Aronson, A. R. (2010).
Knowledge-based biomedical word sense disam-
biguation: comparison of approaches. BMC bioinfor-
matics, 11(1):569.
Jimeno-Yepes, A. J., McInnes, B. T., and Aronson, A. R.
(2011). Exploiting mesh indexing in medline to gen-
erate a data set for word sense disambiguation. BMC
bioinformatics, 12(1):223.
Jurafsky, D. and Martin, J. H. (2020). Speech
& language processing [Book in preparation].
https://web.stanford.edu/ jurafsky/slp3/.
Koren
ˇ
ci
´
c, D., Ristov, S., and
ˇ
Snajder, J. (2018). Document-
based topic coherence measures for news media text.
Expert Systems with Applications, 114:357–373.
Lesk, M. (1986). Automatic sense disambiguation using
machine readable dictionaries: how to tell a pine cone
from an ice cream cone. In Proceedings of the 5th an-
nual international conference on Systems documenta-
tion, pages 24–26.
Lin, D. et al. (1998). An information-theoretic definition of
similarity. In Icml, volume 98, pages 296–304.
McInnes, B. (2008). An unsupervised vector approach to
biomedical term disambiguation: integrating umls and
medline. In Proceedings of the ACL-08: HLT Student
Research Workshop, pages 49–54.
Miller, G. A. (1995). Wordnet: a lexical database for en-
glish. Communications of the ACM, 38(11):39–41.
Navigli, R. (2009). Word sense disambiguation: A survey.
ACM computing surveys (CSUR), 41(2):1–69.
Neumann, M., King, D., Beltagy, I., and Ammar, W.
(2019). Scispacy: Fast and robust models for biomed-
ical natural language processing. arXiv preprint
arXiv:1902.07669.
Pesaranghader, A., Matwin, S., Sokolova, M., and Pe-
saranghader, A. (2019). deepbiowsd: effective deep
neural word sense disambiguation of biomedical text
data. JAMIA, 26(5):438–446.
Pustejovsky, J. and Stubbs, A. (2012). Natural Language
Annotation for Machine Learning: A guide to corpus-
building for applications. ” O’Reilly Media, Inc.”.
Pyysalo, S., Ginter, F., Tapio, S., and Sophia, A. (2013).
Distributional semantics resources for biomedical text
processing. Proceedings of LBM, pages 39–44.
Saeed, J. I. (2008). Semantics. Wiley-Blackwell.
Tversky, A. (1977). Features of similarity. Psychological
review, 84(4):327.
Velazquez, E., Ratt
´
e, S., and de Jong, F. (2016). Analyz-
ing students’ knowledge building skills by compar-
ing their written production to syllabus. In Interna-
tional Conference on Interactive Collaborative Learn-
ing, pages 345–352. Springer.
Wang, Y., Zheng, K., Xu, H., and Mei, Q. (2018). Inter-
active medical word sense disambiguation through in-
formed learning. JAMIA, 25(7):800–808.
Weeber, M., Mork, J. G., and Aronson, A. R. (2001). Devel-
oping a test collection for biomedical word sense dis-
ambiguation. In Proceedings of the AMIA Symposium,
page 746. American Medical Informatics Association.
Zhang, C., Bi
´
s, D., Liu, X., and He, Z. (2019). Biomedi-
cal word sense disambiguation with bidirectional long
short-term memory and attention-based neural net-
works. BMC bioinformatics, 20(16):502.
HEALTHINF 2021 - 14th International Conference on Health Informatics
280