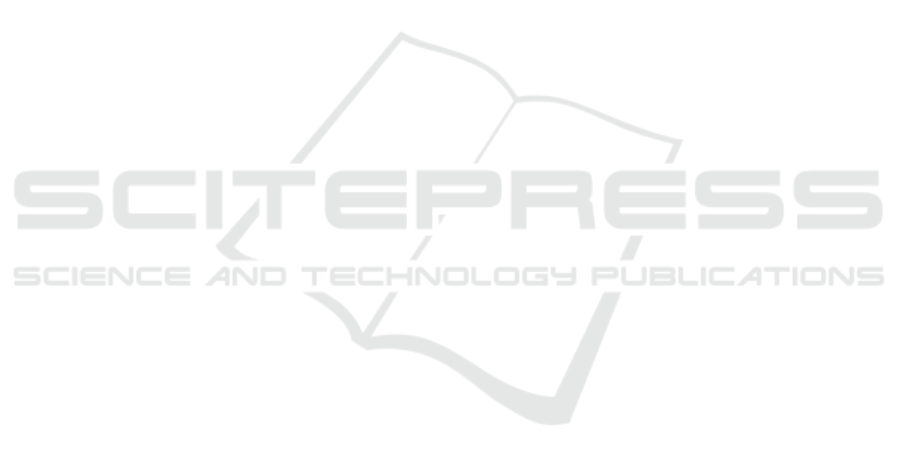
CT (systematized nomenclature of medicine – clinical
terms), and MeSH (medical subject headings).
5 CONCLUSIONS
In this study, we investigated the use of prototype em-
beddings for terminology expansion, specifically for
extracting symptoms of urinary tract infections from
clinical text corpora. Four word embedding methods
were used for deriving the higher-level prototype em-
beddings; it was observed that FastText yielded the
best results. We also explored two statistical phrase
detection methods and, while there was little differ-
ence between them, we also studied the trade-off be-
tween the number and quality of identified phrases
and its impact on the downstream terminology expan-
sion task. We also observed that using a somewhat
smaller but high-quality, relevant corpus generally
gave better results than using a larger yet less precise
corpus; however, this seems to depend on the target
concept’s abstraction level. Indeed, two levels of ab-
straction were compared and contrasted: both yielded
good results, but using prototype embeddings for spe-
cific symptoms overall outperformed the use of pro-
totype embeddings for urinary tract infection symp-
toms in general. Ultimately, we were able to identify
an additional 142 symptoms for inclusion in the ter-
minology with very little manual effort required.
ACKNOWLEDGEMENTS
This research has been approved by the Regional Eth-
ical Review Board in Stockholm under permission no.
2016/2309-32.
REFERENCES
Artetxe, M., Labaka, G., and Agirre, E. (2018). Unsuper-
vised statistical machine translation. arXiv preprint
arXiv:1809.01272.
Bojanowski, P., Grave, E., Joulin, A., and Mikolov, T.
(2017). Enriching word vectors with subword infor-
mation. Transactions of the Association for Computa-
tional Linguistics, 5:135–146.
Bouma, G. (2009). Normalized (pointwise) mutual in-
formation in collocation extraction. Proceedings of
GSCL, pages 31–40.
Dalianis, H. (2018). Clinical text mining: Secondary use of
electronic patient records. Springer, Open Access.
Dalianis, H., Henriksson, A., Kvist, M., Velupillai, S., and
Weegar, R. (2015). Health bank-a workbench for data
science applications in healthcare. In CAiSE Industry
Track, pages 1–18.
Devlin, J., Chang, M.-W., Lee, K., and Toutanova, K.
(2018). Bert: Pre-training of deep bidirectional trans-
formers for language understanding. arXiv preprint
arXiv:1810.04805.
ECDC (2016). Point prevalence survey of healthcare-
associated infections and antimicrobial use in Eu-
ropean acute care hospitals protocol version 5.3 :
ECDC PPS 2016–2017. ECDC, Stockholm.
Fan, Y., Pakhomov, S., McEwan, R., Zhao, W., Lindemann,
E., and Zhang, R. (2019). Using word embeddings to
expand terminology of dietary supplements on clinical
notes. JAMIA open, 2(2):246–253.
Foxman, B. (2010). The epidemiology of urinary tract in-
fection. Nature Reviews Urology, 7(12):653.
Harris, Z. S. (1954). Distributional structure. Word.
Henriksson, A. (2015). Learning multiple distributed proto-
types of semantic categories for named entity recogni-
tion. International journal of data mining and bioin-
formatics, 13(4):395–411.
Henriksson, A., Dalianis, H., and Kowalski, S. (2014a).
Generating features for named entity recognition by
learning prototypes in semantic space: The case of
de-identifying health records. In 2014 IEEE Interna-
tional Conference on Bioinformatics and Biomedicine
(BIBM), pages 450–457. IEEE.
Henriksson, A., Moen, H., Skeppstedt, M., Daudaravicius,
V., and Duneld, M. (2014b). Synonym extraction and
abbreviation expansion with ensembles of semantic
spaces. Journal of Biomedical Semantics, 5(6).
Herzog, K., Dusel, J. E., Hugentobler, M., Beutin, L.,
S
¨
agesser, G., Stephan, R., H
¨
achler, H., and N
¨
uesch-
Inderbinen, M. (2014). Diarrheagenic enteroag-
gregative escherichia coli causing urinary tract in-
fection and bacteremia leading to sepsis. Infection,
42(2):441–444.
Khattak, F. K., Jeblee, S., Pou-Prom, C., Abdalla, M.,
Meaney, C., and Rudzicz, F. (2019). A survey of word
embeddings for clinical text. Journal of Biomedical
Informatics: X, 4:100057.
Landers, T., Apte, M., Hyman, S., Furuya, Y., Glied, S.,
and Larson, E. (2010). A comparison of methods to
detect urinary tract infections using electronic data.
The Joint Commission Journal on Quality and Patient
Safety, 36(9):411–417.
Mikolov, T., Sutskever, I., Chen, K., Corrado, G. S., and
Dean, J. (2013). Distributed representations of words
and phrases and their compositionality. In Advances in
neural information processing systems, pages 3111–
3119.
NHSN (2017). National Healthcare Safety Network
(NHSN) Patient Safety Component Manual, Centers
for Disease Control and Prevention; 2017. NHSN,
U.S. Department of Health & Human Services.
Pennington, J., Socher, R., and Manning, C. (2014). Glove:
Global vectors for word representation. In Proceed-
ings of the 2014 conference on empirical methods in
natural language processing (EMNLP), pages 1532–
1543.
HEALTHINF 2021 - 14th International Conference on Health Informatics
56