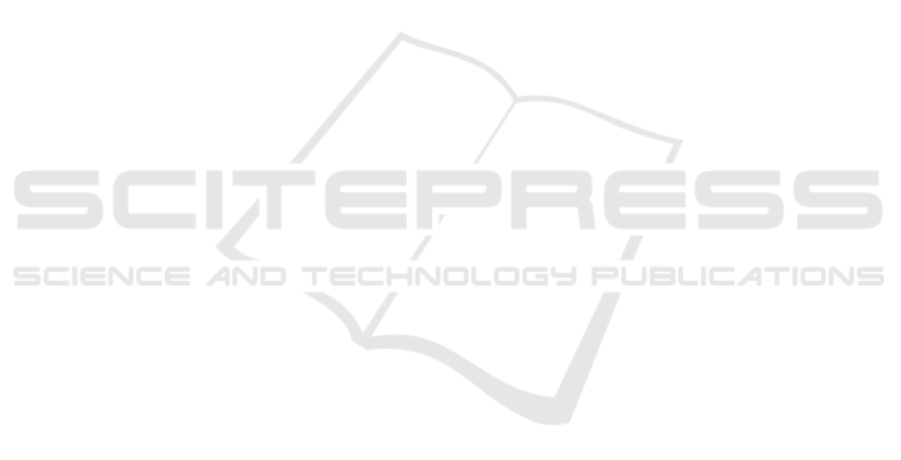
REFERENCES
AlDahoul, N., Md Sabri, A. Q., and Mansoor, A. M. (2018).
Real-time human detection for aerial captured video
sequences via deep models. Computational intelli-
gence and neuroscience, 2018.
Cristani, M., Del Bue, A., Murino, V., Setti, F., and Vincia-
relli, A. (2020). The visual social distancing problem.
arXiv preprint arXiv:2005.04813.
Hazar, M., Arous, O., and Hammami, M. (2019). Abnormal
crowd density estimation in aerial images. Journal of
Electronic Imaging, 28(1):013047.
He, K., Zhang, X., Ren, S., and Sun, J. (2016). Deep resid-
ual learning for image recognition. In Proceedings of
the IEEE conference on computer vision and pattern
recognition, pages 770–778.
Krizhevsky, A., Sutskever, I., and Hinton, G. E. (2012). Im-
agenet classification with deep convolutional neural
networks. In Advances in neural information process-
ing systems, pages 1097–1105.
Lewnard, J. A. and Lo, N. C. (2020). Scientific and ethical
basis for social-distancing interventions against covid-
19. The Lancet. Infectious diseases, 20(6):631.
Meynberg, O., Cui, S., and Reinartz, P. (2016). Detection
of high-density crowds in aerial images using texture
classification. Remote Sensing, 8(6):470.
Meynberg, O. and Kuschk, G. (2013). Airborne crowd den-
sity estimation. ISPRS Annals of the Photogramme-
try, Remote Sensing and Spatial Information Sciences,
2:49–54.
Mliki, H., Bouhlel, F., and Hammami, M. (2020). Hu-
man activity recognition from uav-captured video se-
quences. Pattern Recognition, 100:107140.
Nagendran, A., Harper, D., and Shah, M. (2010). UCF-
ARG dataset, university of central florida. http://crcv.
ucf.edu/data/UCF-ARG.php.
Park, S. W., Sun, K., Viboud, C., Grenfell, B. T., and
Dushoff, J. (2020). Potential roles of social distancing
in mitigating the spread of coronavirus disease 2019
(covid-19) in south korea. medRxiv.
Punn, N. S., Sonbhadra, S. K., and Agarwal, S. (2020).
Monitoring covid-19 social distancing with person de-
tection and tracking via fine-tuned yolo v3 and deep-
sort techniques. arXiv preprint arXiv:2005.01385.
Repici, A., Maselli, R., Colombo, M., Gabbiadini, R.,
Spadaccini, M., Anderloni, A., Carrara, S., Fugazza,
A., Di Leo, M., and Galtieri, P. A. (2020). Coron-
avirus (covid-19) outbreak: what the department of
endoscopy should know. Gastrointestinal endoscopy.
Simonyan, K. and Zisserman, A. (2014). Very deep con-
volutional networks for large-scale image recognition.
arXiv preprint arXiv:1409.1556.
Singh, R. and Adhikari, R. (2020). Age-structured impact
of social distancing on the covid-19 epidemic in india.
arXiv preprint arXiv:2003.12055.
Szegedy, C., Liu, W., Jia, Y., Sermanet, P., Reed, S.,
Anguelov, D., Erhan, D., Vanhoucke, V., and Rabi-
novich, A. (2015). Going deeper with convolutions.
In Proceedings of the IEEE conference on computer
vision and pattern recognition, pages 1–9.
Thota, S. D., Vemulapalli, K. S., Chintalapati, K., and
Gudipudi, P. S. S. (2013). Comparison between the
optical flow computational techniques. International
Journal of Engineering Trends and Technology, 4(10).
Welch, G. and Bishop, G. (1995). An introduction to the
kalman filter.
WHO (Septembre 4, 2020). World health organization
corona-viruses (covid-19). https://covid19.who.int/.
Wilder-Smith, A. and Freedman, D. O. (2020). Isolation,
quarantine, social distancing and community contain-
ment: pivotal role for old-style public health measures
in the novel coronavirus (2019-ncov) outbreak. Jour-
nal of travel medicine, 27(2):taaa020.
Zeiler, M. D. and Fergus, R. (2014). Visualizing and under-
standing convolutional networks. In European confer-
ence on computer vision, pages 818–833. Springer.
Zhang, J., Litvinova, M., Liang, Y., Wang, Y., Wang, W.,
Zhao, S., Wu, Q., Merler, S., Viboud, C., and Vespig-
nani, A. (2020). Age profile of susceptibility, mixing,
and social distancing shape the dynamics of the novel
coronavirus disease 2019 outbreak in china. medRxiv.
Zhou, F., Yu, T., Du, R., Fan, G., Liu, Y., Liu, Z., Xiang,
J., Wang, Y., Song, B., and Gu, X. (2020). Clinical
course and risk factors for mortality of adult inpatients
with covid-19 in wuhan, china: a retrospective cohort
study. The lancet.
Zitnick, C. L. and Doll
´
ar, P. (2014). Edge boxes: Locating
object proposals from edges. In European conference
on computer vision, pages 391–405. Springer.
VISAPP 2021 - 16th International Conference on Computer Vision Theory and Applications
280