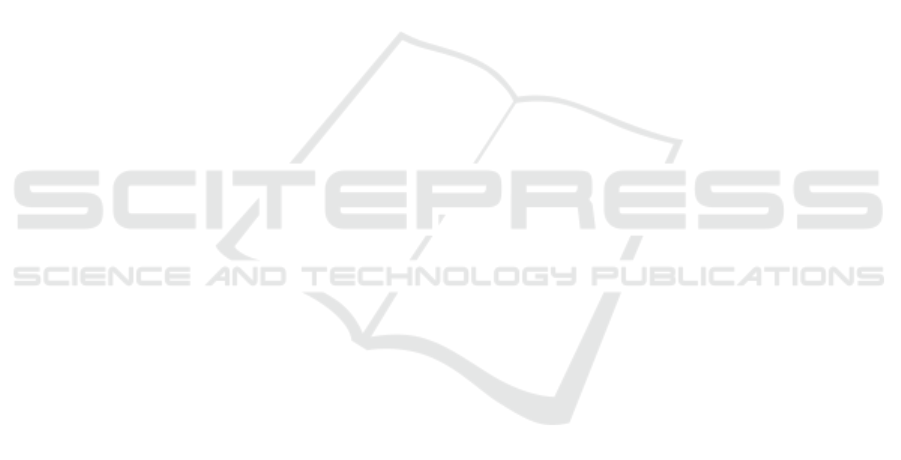
ing (Toderici et al., 2016). However, this was not the
goal of our paper. Rather, our purpose was to ex-
plore the potential of skeletons as an alternative tool
to image representation. We believe that our results
show that skeletons, when combined with saliency
maps, offer a promising tool for lossy image encod-
ing, which can be refined next in the direction of com-
petitive image compression.
We next aim to study more effective ways to en-
code skeletons prior to compression using piecewise-
spline representations. Separately, we aim to test
our method for simplifying general 2D and 3D
scalar fields in scientific visualization, weighted with
uncertainty-based saliency maps. In the long run, as
outlined above, we believe that skeletons and saliency
maps can provide effective and efficient tools for
general-purpose, but also application-specific, lossy
image representation.
ACKNOWLEDGEMENTS
The first author acknowledges the China Scholarship
Council (Grant number: 201806320354) for financial
support.
REFERENCES
Alaei, A., Raveaux, R., and Conte, D. (2017). Image quality
assessment based on regions of interest. Signal, Image
and Video Processing, 11:673–680.
Andrushia, A. and Thangarjan, R. (2018). Saliency-based
image compression using Walsh-Hadamard transform
(WHT). In Lecture Notes in Computational Vision and
Biomechanics, pages 21–42. Springer.
Borji, A., Cheng, M., Jiang, H., and Li, J. (2015).
Salient object detection: A benchmark. IEEE TIP,
24(12):5706–5722.
Cazzato, D., Leo, M., Distante, C., and Voos, H. (2020).
When i look into your eyes: A survey on computer
vision contributions for human gaze estimation and
tracking. Sensors, 20:3739.
Chen, T., Cheng, M., Tan, P., Shamir, A., and Hu, S. (2009).
Sketch2photo: Internet image montage. ACM Trans.
Graph., 28(5):1–10.
Cheng, M. (2014). MSRA10K salient object database. http:
//mmcheng.net/msra10k.
Cheng, M., Mitra, N. J., Huang, X., Torr, P. H., and Hu, S.
(2014). Global contrast based salient region detection.
IEEE TPAMI, 37(3):569–582.
Costa, L. d. F. D. and Cesar, R. M. (2000). Shape Analysis
and Classification: Theory and Practice. CRC Press,
Inc., USA, 1st edition.
de Melo Joao, L., de Castro Belem, F., and Falcao, A. X.
(2020). Itself: Iterative saliency estimation flexible
framework. Available at https://arxiv.org/abs/2006.
16956.
Engelke, U. and Le Callet, P. (2015). Perceived interest
and overt visual attention in natural images. Signal
Processing: Image Communication, 39:386–404.
Falc
˜
ao, A., Stolfi, J., and Lotufo, R. (2004). The image
foresting transform: Theory, algorithms, and applica-
tions. IEEE TPAMI, 26(1):19–29.
Goferman, S., Zelnik, L., and Tal, A. (2011). Context-aware
saliency detection. IEEE TPAMI, 34(10):1915–1926.
Jiang, H., Wang, J., Yuan, Z., Wu, Y., Zheng, N., and Li,
S. (2013). Salient object detection: A discriminative
regional feature integration approach. In 2013 IEEE
Conference on Computer Vision and Pattern Recogni-
tion, pages 2083–2090.
Jiang, P., Ling, H., Yu, J., and Peng, J. (2013). Salient re-
gion detection by ufo: Uniqueness, focusness and ob-
jectness. In Proc. ICCV, pages 1976–1983.
Koehoorn, J., Sobiecki, A., Boda, D., Diaconeasa, A.,
Doshi, S., Paisey, S., Jalba, A., and Telea, A. (2015).
Automated digital hair removal by threshold decom-
position and morphological analysis. In Proc. ISMM,
volume 9082, pages 15–26. Springer.
Koehoorn, J., Sobiecki, A., Rauber, P., Jalba, A., and Telea,
A. (2016). Efficient and effective automated digital
hair removal from dermoscopy images. Math. Mor-
phol. Theory Appl., 1.
Le Callet, P. and Niebur, E. (2013). Visual attention and
applications in multimedia technologies. Proceedings
of the IEEE, 101(9):2058–2067.
Li, C. and Bovik, A. (2010). Content-weighted video qual-
ity assessment using a three-component image model.
J. Electronic Imaging, 19:110–130.
Li, X., Lu, H., Zhang, L., Ruan, X., and Yang, M. (2013).
Saliency detection via dense and sparse reconstruc-
tion. In Proc. IEEE ICCV, pages 2976–2983.
Li, X., Lu, H., Zhang, L., Ruan, X., and Yang, M. (2013).
Saliency detection via dense and sparse reconstruc-
tion. In 2013 IEEE International Conference on Com-
puter Vision, pages 2976–2983.
Liu, H., Engelke, U., Wang, J., Callet, Le, P., and Heyn-
derickx, I. (2013). How does image content affect
the added value of visual attention in objective image
quality assessment? IEEE Signal Processing Letters,
20:355–358.
Liu, H. and Heynderickx, I. (2011). Visual attention in
objective image quality assessment: Based on eye-
tracking data. IEEE TCSVT, 21(7):971–982.
Liu, H. and Heynderickx, I. (2011). Visual attention in
objective image quality assessment: Based on eye-
tracking data. IEEE Transactions on Circuits and Sys-
tems for Video Technology, 21(7):971–982.
Movahedi, V. and Elder, J. H. (2010). Design and perceptual
validation of performance measures for salient object
segmentation. In 2010 IEEE Computer Society Con-
ference on Computer Vision and Pattern Recognition
- Workshops, pages 49–56.
Nguyen, T. and Marpe, D. (2012). Performance analysis of
HEVC-based intra coding for still image compression.
In Proc. IEEE Picture Coding Symp., pages 233–236.
VISAPP 2021 - 16th International Conference on Computer Vision Theory and Applications
54