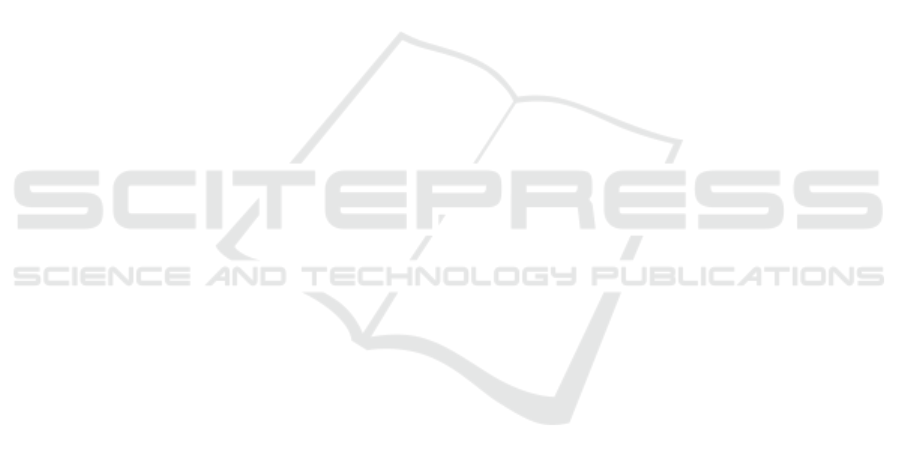
encouraging results, we think there is a huge poten-
tial for ML-based technologies to help people with
dyslexia. As usual with ML, accuracy can still be
improved by gathering more data. ML-based tech-
nologies could definitely avoid the need of manual
analysis and global performances may be improved.
In the future, a better understanding of the correla-
tion between the different disorders could also help
in providing more informed predictions. For instance
adding to audio records, a picture of a handwritten
text could help to make the prediction still more ac-
curate. As far as we know, this is the first time audio
signals analysis is used to detect dyslexia, leading the
path to a non invasive, fast and cost effective screen-
ing tool.
REFERENCES
Al-Barhamtoshy, H. M. and Motaweh, D. M. (2017). Di-
agnosis of dyslexia using computation analysis. In
2017 International Conference on Informatics, Health
Technology (ICIHT), pages 1–7.
American Psychiatric Association (2013). Diagnostic and
statistical manual of mental disorders: DSM-5. Autor,
Washington, DC, 5th ed. edition.
Asvestopoulou, T., Manousaki, V., Psistakis, A., Smyr-
nakis, I., Andreadakis, V., Aslanides, I. M., and
Papadopouli, M. (2019). Dyslexml: Screening
tool for dyslexia using machine learning. CoRR,
abs/1903.06274.
Bellocchi, S., Mathilde, M., Bastien-Toniazzo, M., and
Ducrot, S. (2012). I can read it in your eyes: What eye
movements tell us about visuo-attentional processes in
developmental dyslexia. Research in developmental
disabilities, 34:452–460.
Costa, M., Zavaleta, J., Cruz, S., Manhaes, L., Cerceau,
R., Carvalho, L., and Mousinho, R. (2013). A com-
putational approach for screening dyslexia. In Pro-
ceedings of the 26th IEEE International Symposium
on Computer-Based Medical Systems, pages 565–566.
de Leeuw, R. (2010). Special font for dyslexia?
Duke, U. (2016). Dyslexia international: Bet-
ter training, better teaching. https://www.
dyslexia-international.org/wp-content/uploads/
2016/04/DI-Duke-Report-final-4-29-14.pdf.
Frid, A. and Manevitz, L. M. (2018). Features and machine
learning for correlating and classifying between brain
areas and dyslexia. CoRR, abs/1812.10622.
Gaggi, O., Galiazzo, G., Palazzi, C., Facoetti, A., and
Franceschini, S. (2012). A serious game for predicting
the risk of developmental dyslexia in pre-readers chil-
dren. In 21st International Conference on Computer
Communications and Networks (ICCCN), pages 1–5.
Gaggi, O., Palazzi, C. E., Ciman, M., Galiazzo, G., Frances-
chini, S., Ruffino, M., Gori, S., and Facoetti, A.
(2017). Serious games for early identification of de-
velopmental dyslexia. Comput. Entertain., 15(2):4:1–
4:24.
Geurts, L., Vanden Abeele, V., Celis, V., Husson, J., Au-
denaeren, L., Loyez, L., Goeleven, A., Wouters, J.,
and Ghesqui
`
ere, P. (2015). DIESEL-X: A game-based
tool for early risk detection of dyslexia in preschool-
ers, pages 93–114. Springer International Publishing.
Hart, M. (1971). Project gutenberg. www.gutenberg.org.
Hochreiter S, S. J. (1997). Long short-term memory. Neural
Computation, 9(8):1735–1780.
Huettig, F. and Brouwer, S. (2015). Delayed anticipatory
spoken language processing in adults with dyslexia-
evidence from eye-tracking: Word reading and pre-
dictive language processing. Dyslexia, 21.
Hyona, J., Olson, R., Defries, J., Fulker, D., Penning-
ton, B., and Smith, S. (1995). Eye fixation patterns
among dyslexic and normal readers: Effects of word
length and word frequency. Journal of Experimen-
tal Psychology: Learning, Memory, and Cognition,
21:1430–1440.
Johnson, D. J. (1980). Persistent auditory disorders in
young dyslexic adults. Bulletin of the Orton Society,
30:268–276.
L., V., F., M., G., T., and M., H. (2016). Direct view-
ing of dyslexics’ compensatory strategies in speech
in noise using auditory classification images. PLoS
ONE, 11(4).
Lyytinen, H., Ronimus, M., Alanko, A., Poikkeus, A.-
M., and Taanila, M. (2007). Early identification of
dyslexia and the use of computer game-based prac-
tice to support reading acquisition. Nordic Psychol-
ogy, 59(2):109–126.
M., N. B., G., O. S., J, Y., A., P. T. R., and C, J. (2016).
Screening for dyslexia using eye tracking during read-
ing. PLoS ONE, 11(12).
Palacios, A. M., S
´
anchez, L., and Couso, I. (2010). Diag-
nosis of dyslexia with low quality data with genetic
fuzzy systems. International Journal of Approximate
Reasoning, 51(8):993 – 1009.
PH., T., IG., C., G., P., JN., R., DP., M., and L., I. (2013).
High-throughput classification of clinical populations
from natural viewing eye movements. J Neurol.,
1(260):275–284.
Rauschenberger, M., Rello, L., Baeza-Yates, R., Gomez, E.,
and Bigham, J. P. (2017). Towards the prediction of
dyslexia by a web-based game with musical elements.
In Proceedings of the 14th Web for All Conference on
The Future of Accessible Work, W4A ’17. Association
for Computing Machinery.
Rello, L. and Ballesteros, M. (2015). Detecting readers
with dyslexia using machine learning with eye track-
ing measures. Proceedings of the 12th Web for All
Conference W4A ’15, pages 1–8.
Rello, L., Romero, E., Rauschenberger, M., Ali, A.,
Williams, K., Bigham, J. P., and White, N. C. (2018).
Screening dyslexia for english using HCI measures
and machine learning. In Kostkova, P., Grasso, F.,
Castillo, C., Mejova, Y., Bosman, A., and Edel-
stein, M., editors, Proceedings of the 2018 Interna-
tional Conference on Digital Health, DH 2018, Lyon,
France, April 23-26, 2018, pages 80–84. ACM.
Detecting Dyslexia from Audio Records: An AI Approach
65