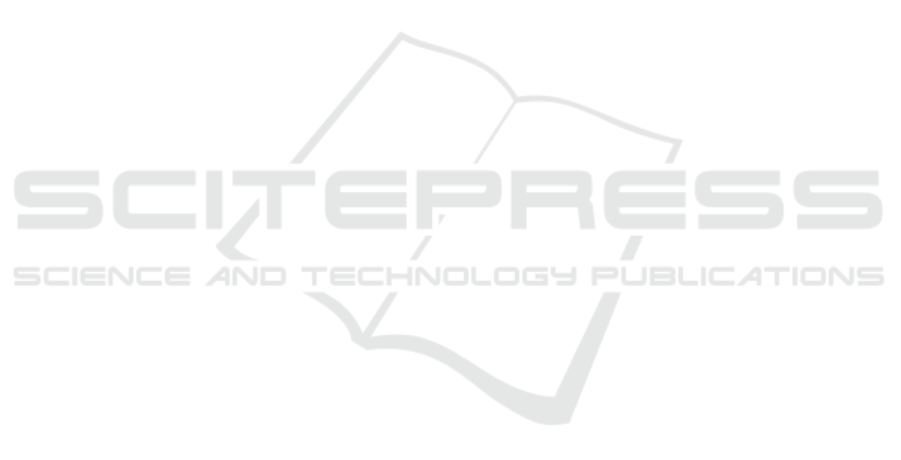
gium, in the context of the BRAIN-be project IN-
SIGHT (Intelligent Neural Systems as InteGrated
Heritage Tools). The authors would like to thank all
researchers that have worked on collecting and an-
notating the datasets used in this study, in particu-
lar I. Salmon’s lab (ULB, BE) and D. Cataldo’s lab
(ULi
`
ege, BE) for the DP datasets. Matthia Sabatelli
wishes to thank Gilles Louppe for the fruitful brain-
storming sessions, and Michela Paganini for the in-
sightful discussions about the Lottery-Ticket Hypoth-
esis and its potential applications to transfer learning.
REFERENCES
Dong, X., Chen, S., and Pan, S. (2017). Learning to prune
deep neural networks via layer-wise optimal brain sur-
geon. In Advances in Neural Information Processing
Systems, pages 4857–4867.
Everingham, M., Van Gool, L., Williams, C. K., Winn, J.,
and Zisserman, A. (2010). The pascal visual object
classes (voc) challenge. International journal of com-
puter vision, 88(2):303–338.
Frankle, J. and Carbin, M. (2018). The lottery ticket hypoth-
esis: Finding sparse, trainable neural networks. arXiv
preprint arXiv:1803.03635.
Frankle, J., Dziugaite, G. K., Roy, D., and Carbin, M.
(2019a). Stabilizing the lottery ticket hypothesis.
arXiv preprint arXiv:1903.01611.
Frankle, J., Dziugaite, G. K., Roy, D. M., and Carbin, M.
(2019b). Linear mode connectivity and the lottery
ticket hypothesis. arXiv preprint arXiv:1912.05671.
Gohil, V., Narayanan, S. D., and Jain, A. (2020). One ticket
to win them all: generalizing lottery ticket initializa-
tions across datasets and optimizers. ReScience-C.
Han, S., Mao, H., and Dally, W. J. (2015a). Deep compres-
sion: Compressing deep neural networks with prun-
ing, trained quantization and huffman coding. arXiv
preprint arXiv:1510.00149.
Han, S., Pool, J., Tran, J., and Dally, W. (2015b). Learning
both weights and connections for efficient neural net-
work. In Advances in neural information processing
systems, pages 1135–1143.
He, K., Zhang, X., Ren, S., and Sun, J. (2016). Deep resid-
ual learning for image recognition. In Proceedings of
the IEEE conference on computer vision and pattern
recognition, pages 770–778.
Kainz, P., Burgsteiner, H., Asslaber, M., and Ahammer, H.
(2017). Training echo state networks for rotation-
invariant bone marrow cell classification. Neural
Computing and Applications, 28(6):1277–1292.
Lin, J., Rao, Y., Lu, J., and Zhou, J. (2017). Runtime neural
pruning. In Advances in Neural Information Process-
ing Systems, pages 2181–2191.
Lin, T.-Y., Maire, M., Belongie, S., Hays, J., Perona, P.,
Ramanan, D., Doll
´
ar, P., and Zitnick, C. L. (2014).
Microsoft coco: Common objects in context. In Euro-
pean conference on computer vision, pages 740–755.
Springer.
Mar
´
ee, R., Rollus, L., St
´
evens, B., Hoyoux, R., Louppe,
G., Vandaele, R., Begon, J.-M., Kainz, P., Geurts, P.,
and Wehenkel, L. (2016). Collaborative analysis of
multi-gigapixel imaging data using cytomine. Bioin-
formatics, 32(9):1395–1401.
Mehta, R. (2019). Sparse transfer learning via winning lot-
tery tickets. arXiv preprint arXiv:1905.07785.
Mensink, T. and Van Gemert, J. (2014). The rijksmuseum
challenge: Museum-centered visual recognition. In
Proceedings of International Conference on Multime-
dia Retrieval, pages 451–454.
Molchanov, P., Tyree, S., Karras, T., Aila, T., and Kautz,
J. (2016). Pruning convolutional neural networks
for resource efficient inference. arXiv preprint
arXiv:1611.06440.
Morcos, A., Yu, H., Paganini, M., and Tian, Y. (2019).
One ticket to win them all: generalizing lottery ticket
initializations across datasets and optimizers. In Ad-
vances in Neural Information Processing Systems,
pages 4933–4943.
Mormont, R., Geurts, P., and Mar
´
ee, R. (2018). Com-
parison of deep transfer learning strategies for digital
pathology. In Proceedings of the IEEE Conference on
Computer Vision and Pattern Recognition Workshops,
pages 2262–2271.
Phillips, F. and Mackintosh, B. (2011). Wiki art gallery,
inc.: A case for critical thinking. Issues in Accounting
Education, 26(3):593–608.
Russakovsky, O., Deng, J., Su, H., Krause, J., Satheesh, S.,
Ma, S., Huang, Z., Karpathy, A., Khosla, A., Bern-
stein, M., et al. (2015). Imagenet large scale visual
recognition challenge. International journal of com-
puter vision, 115(3):211–252.
Sabatelli, M., Kestemont, M., Daelemans, W., and Geurts,
P. (2018). Deep transfer learning for art classification
problems. In Proceedings of the European Conference
on Computer Vision (ECCV), pages 631–646.
Strezoski, G. and Worring, M. (2017). Omniart: multi-task
deep learning for artistic data analysis. arXiv preprint
arXiv:1708.00684.
Strezoski, G. and Worring, M. (2018). Omniart: a large-
scale artistic benchmark. ACM Transactions on Mul-
timedia Computing, Communications, and Applica-
tions (TOMM), 14(4):1–21.
Sun, T., Shao, Y., Li, X., Liu, P., Yan, H., Qiu, X.,
and Huang, X. (2019). Learning sparse sharing
architectures for multiple tasks. arXiv preprint
arXiv:1911.05034.
Szegedy, C., Liu, W., Jia, Y., Sermanet, P., Reed, S.,
Anguelov, D., Erhan, D., Vanhoucke, V., and Rabi-
novich, A. (2015). Going deeper with convolutions.
In Proceedings of the IEEE conference on computer
vision and pattern recognition, pages 1–9.
Van Soelen, R. and Sheppard, J. W. (2019). Using win-
ning lottery tickets in transfer learning for convolu-
tional neural networks. In 2019 International Joint
Conference on Neural Networks (IJCNN), pages 1–8.
IEEE.
VISAPP 2021 - 16th International Conference on Computer Vision Theory and Applications
68