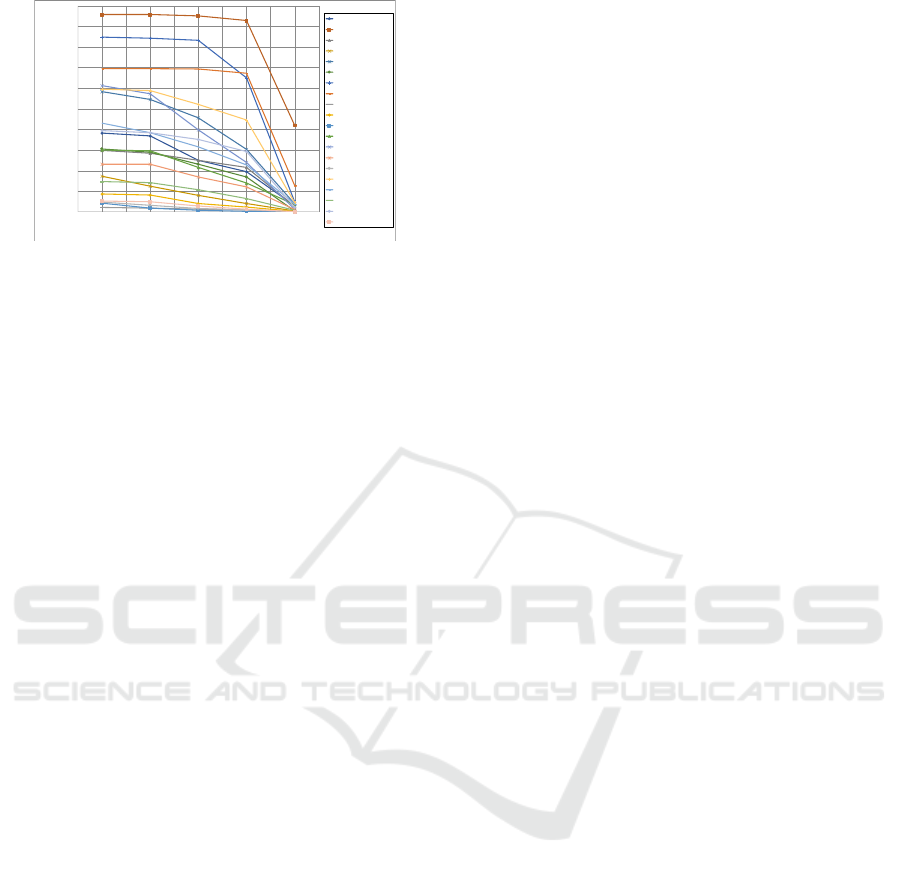
0.0%
10.0%
20.0%
30.0%
40.0%
50.0%
60.0%
70.0%
80.0%
90.0%
100.0%
0 0.1 0.2 0.3 0.4 0.5 0.6 0.7 0.8 0.9 1
AP
IoU thres hhold
read
kick
drink
eat_instr
cut_obj
cut_instr
hit_obj
catch
throw
ride
ski
lay
talk_on_phone
hit_instr
snowboard
eat_obj
work_on_computer
carry
skateboard
surf
Figure 10: AP vs. IoU threshold on V-COCO test set (ours
with Faster R-CNN).
are more commonly available than those for HOI de-
tection. The proposed method with Faster R-CNN at-
tained better performance on some labels than a state-
of-the-art method for HOI detection based on super-
vised learning.
In future work, we will evaluate the performance
when we use the person regions detected by a per-
son detector as inputs to the proposed method because
we assumed in this study that the person regions were
perfectly detected. In order to improve performance,
it is also our future work to extend another part of
PCL such as pseudo-ground truth BB generation for
HOI detection because we extended only the MIDN
part in this paper.
ACKNOWLEDGEMENTS
This study was supported by JSPS KAKENHI Grant
Number JP17K06608 and JP20K12115.
REFERENCES
Arbel
´
aez, P., Pont-Tuset, J., Barron, J. T., Marques, F., and
Malik, J. (2014). Multiscale combinatorial grouping.
In Proceedings of the IEEE Conference on Computer
Vision and Pattern Recognition, pages 328–335.
Bearman, A., Russakovsky, O., Ferrari, V., and Fei-Fei, L.
(2016). What’s the point: Semantic segmentation with
point supervision. In Proceedings of the European
Conference on Computer Vision, pages 549–565.
Bilen, H. and Vedaldi, A. (2016). Weakly supervised deep
detection networks. In Proceedings of the IEEE Con-
ference on Computer Vision and Pattern Recognition,
pages 2846–2854.
Deselaers, T., Alexe, B., and Ferrari, V. (2012). Weakly su-
pervised localization and learning with generic knowl-
edge. International Journal of Computer Vision,
100(3):275–293.
Gao, C., Zou, Y., and Huang, J.-B. (2018). ican: Instance-
centric attention network for human-object interaction
detection. In Proceedings of the British Machine Vi-
sion Conference.
Gkioxari, G., Girshick, R., Doll
´
ar, P., and He, K. (2018).
Detecting and recognizing human-object interactions.
In Proceedings of the IEEE Conference on Computer
Vision and Pattern Recognition, pages 8359–8367.
Gupta, S. and Malik, J. (2015). Visual semantic role label-
ing. arXiv preprint arXiv:1505.04474.
Lin, T.-Y., Maire, M., Belongie, S., Hays, J., Perona, P., Ra-
manan, D., Doll
´
ar, P., and Zitnick, C. L. (2014). Mi-
crosoft COCO: Common objects in context. In Pro-
ceedings of European Conference on Computer Vi-
sion, pages 740–755.
Ren, S., He, K., Girshick, R., and Sun, J. (2015). Faster R-
CNN: Towards real-time object detection with region
proposal networks. In Advances in Neural Informa-
tion Processing Systems, pages 91–99.
Simonyan, K. and Zisserman, A. (2015). Very deep con-
volutional networks for large-scale image recognition.
In International Conference on Learning Representa-
tions.
Tang, P., Wang, X., Bai, S., Shen, W., Bai, X., Liu, W.,
and Yuille, A. (2018). PCL: Proposal cluster learning
for weakly supervised object detection. IEEE Trans-
actions on Pattern Analysis and Machine Intelligence,
42(1):176–191.
Tang, P., Wang, X., Bai, X., and Liu, W. (2017). Multiple
instance detection network with online instance clas-
sifier refinement. In Proceedings of the IEEE Con-
ference on Computer Vision and Pattern Recognition,
pages 2843–2851.
Ulutan, O., Iftekhar, A. S. M., and Manjunath, B. S. (2020).
VSGNet: Spatial attention network for detecting hu-
man object interactions using graph convolutions. In
Proceedings of the IEEE Conference on Computer Vi-
sion and Pattern Recognition, pages 13617–13626.
Wan, F., Wei, P., Jiao, J., Han, Z., and Ye, Q. (2018).
Min-entropy latent model for weakly supervised ob-
ject detection. In Proceedings of the IEEE Conference
on Computer Vision and Pattern Recognition, pages
1297–1306.
Yang, Z., Mahajan, D., Ghadiyaram, D., Nevatia, R., and
Ramanathan, V. (2019). Activity driven weakly su-
pervised object detection. In Proceedings of the IEEE
Conference on Computer Vision and Pattern Recogni-
tion, pages 2917–2926.
VISAPP 2021 - 16th International Conference on Computer Vision Theory and Applications
300