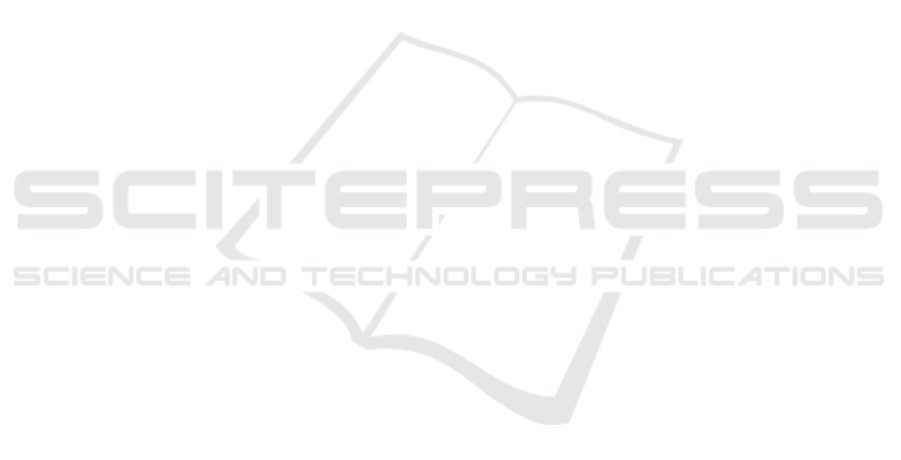
REFERENCES
Alexandre, L. A. (2012). 3d descriptors for object and cate-
gory recognition: a comparative evaluation. In Work-
shop on Color-Depth Camera Fusion in Robotics at
the IEEE/RSJ International Conference on Intelligent
Robots and Systems (IROS), Vilamoura, Portugal, vol-
ume 1, page 7.
Alhamzi, K., Elmogy, M., and Barakat, S. (2015). 3d object
recognition based on local and global features using
point cloud library. International Journal of Advance-
ments in Computing Technology, 7(3):43.
Carvalho, L. and von Wangenheim, A. (2019). 3d ob-
ject recognition and classification: a systematic lit-
erature review. Pattern Analysis and Applications,
22(4):1243–1292.
Chen, J., Fang, Y., and Cho, Y. K. (2018). Performance
evaluation of 3d descriptors for object recognition in
construction applications. Automation in Construc-
tion, 86:44–52.
Cop, K. P., Borges, P. V., and Dub
´
e, R. (2018). Delight: An
efficient descriptor for global localisation using lidar
intensities. In 2018 IEEE International Conference on
Robotics and Automation (ICRA), pages 3653–3660.
IEEE.
Cortes, C. and Vapnik, V. (1995). Support-vector networks.
Machine learning, 20(3):273–297.
Costanzo., M., Maria., G. D., Lettera., G., Natale., C., and
Pirozzi., S. (2018). Flexible motion planning for ob-
ject manipulation in cluttered scenes. In Proceed-
ings of the 15th International Conference on Informat-
ics in Control, Automation and Robotics - Volume 1:
ICINCO,, pages 110–121. INSTICC, SciTePress.
do Monte Lima, J. P. S. and Teichrieb, V. (2016). An ef-
ficient global point cloud descriptor for object recog-
nition and pose estimation. In 2016 29th SIBGRAPI
Conference on Graphics, Patterns and Images (SIB-
GRAPI), pages 56–63. IEEE.
Filipe, S. and Alexandre, L. A. (2014). A comparative
evaluation of 3d keypoint detectors in a rgb-d object
dataset. In 2014 International Conference on Com-
puter Vision Theory and Applications (VISAPP), vol-
ume 1, pages 476–483. IEEE.
Frome, A., Huber, D., Kolluri, R., B
¨
ulow, T., and Malik,
J. (2004). Recognizing objects in range data using
regional point descriptors. In European conference on
computer vision, pages 224–237. Springer.
Garstka., J. and Peters., G. (2016). Evaluation of local 3-
d point cloud descriptors in terms of suitability for
object classification. In Proceedings of the 13th In-
ternational Conference on Informatics in Control, Au-
tomation and Robotics - Volume 2: ICINCO,, pages
540–547. INSTICC, SciTePress.
Guo, J., Borges, P. V., Park, C., and Gawel, A. (2019).
Local descriptor for robust place recognition using li-
dar intensity. IEEE Robotics and Automation Letters,
4(2):1470–1477.
Guo, Y., Bennamoun, M., Sohel, F., Lu, M., and Wan,
J. (2014). 3d object recognition in cluttered scenes
with local surface features: a survey. IEEE Transac-
tions on Pattern Analysis and Machine Intelligence,
36(11):2270–2287.
Guo, Y., Bennamoun, M., Sohel, F., Lu, M., Wan, J., and
Kwok, N. M. (2016). A comprehensive performance
evaluation of 3d local feature descriptors. Interna-
tional Journal of Computer Vision, 116(1):66–89.
Guo, Y., Wang, H., Hu, Q., Liu, H., Liu, L., and Ben-
namoun, M. (2020). Deep learning for 3d point
clouds: A survey. IEEE Transactions on Pattern Anal-
ysis and Machine Intelligence.
Hana, X.-F., Jin, J. S., Xie, J., Wang, M.-J., and Jiang, W.
(2018). A comprehensive review of 3d point cloud
descriptors. arXiv preprint arXiv:1802.02297.
Jolliffe, I. T. and Cadima, J. (2016). Principal compo-
nent analysis: a review and recent developments.
Philosophical Transactions of the Royal Society A:
Mathematical, Physical and Engineering Sciences,
374(2065):20150202.
Lai, K., Bo, L., Ren, X., and Fox, D. (2011). A large-
scale hierarchical multi-view rgb-d object dataset. In
2011 IEEE international conference on robotics and
automation, pages 1817–1824. IEEE.
Lee, U., Jung, J., Shin, S., Jeong, Y., Park, K., Shim, D. H.,
and Kweon, I.-s. (2016). Eurecar turbo: A self-driving
car that can handle adverse weather conditions. In
2016 IEEE/RSJ International Conference on Intelli-
gent Robots and Systems (IROS), pages 2301–2306.
IEEE.
Maturana, D. and Scherer, S. (2015). Voxnet: A 3d con-
volutional neural network for real-time object recog-
nition. In 2015 IEEE/RSJ International Conference
on Intelligent Robots and Systems (IROS), pages 922–
928. IEEE.
Qi, C. R., Yi, L., Su, H., and Guibas, L. J. (2017). Point-
net++: Deep hierarchical feature learning on point sets
in a metric space. In Guyon, I., Luxburg, U. V., Ben-
gio, S., Wallach, H., Fergus, R., Vishwanathan, S.,
and Garnett, R., editors, Advances in Neural Infor-
mation Processing Systems, volume 30, pages 5099–
5108. Curran Associates, Inc.
Salti, S., Tombari, F., and Di Stefano, L. (2014). Shot:
Unique signatures of histograms for surface and tex-
ture description. Computer Vision and Image Under-
standing, 125:251–264.
Schwarz, M., Schulz, H., and Behnke, S. (2015). Rgb-d
object recognition and pose estimation based on pre-
trained convolutional neural network features. In 2015
IEEE international conference on robotics and au-
tomation (ICRA), pages 1329–1335. IEEE.
Tombari, F., Salti, S., and Di Stefano, L. (2010). Unique
shape context for 3d data description. In Proceedings
of the ACM workshop on 3D object retrieval, pages
57–62.
Wolcott, R. W. and Eustice, R. M. (2014). Visual local-
ization within lidar maps for automated urban driving.
In 2014 IEEE/RSJ International Conference on Intel-
ligent Robots and Systems, pages 176–183. IEEE.
Zaki, H. F., Shafait, F., and Mian, A. (2016). Convolu-
tional hypercube pyramid for accurate rgb-d object
category and instance recognition. In 2016 IEEE In-
ternational Conference on Robotics and Automation
(ICRA), pages 1685–1692. IEEE.
VISAPP 2021 - 16th International Conference on Computer Vision Theory and Applications
308